Blog
Blog
Blog
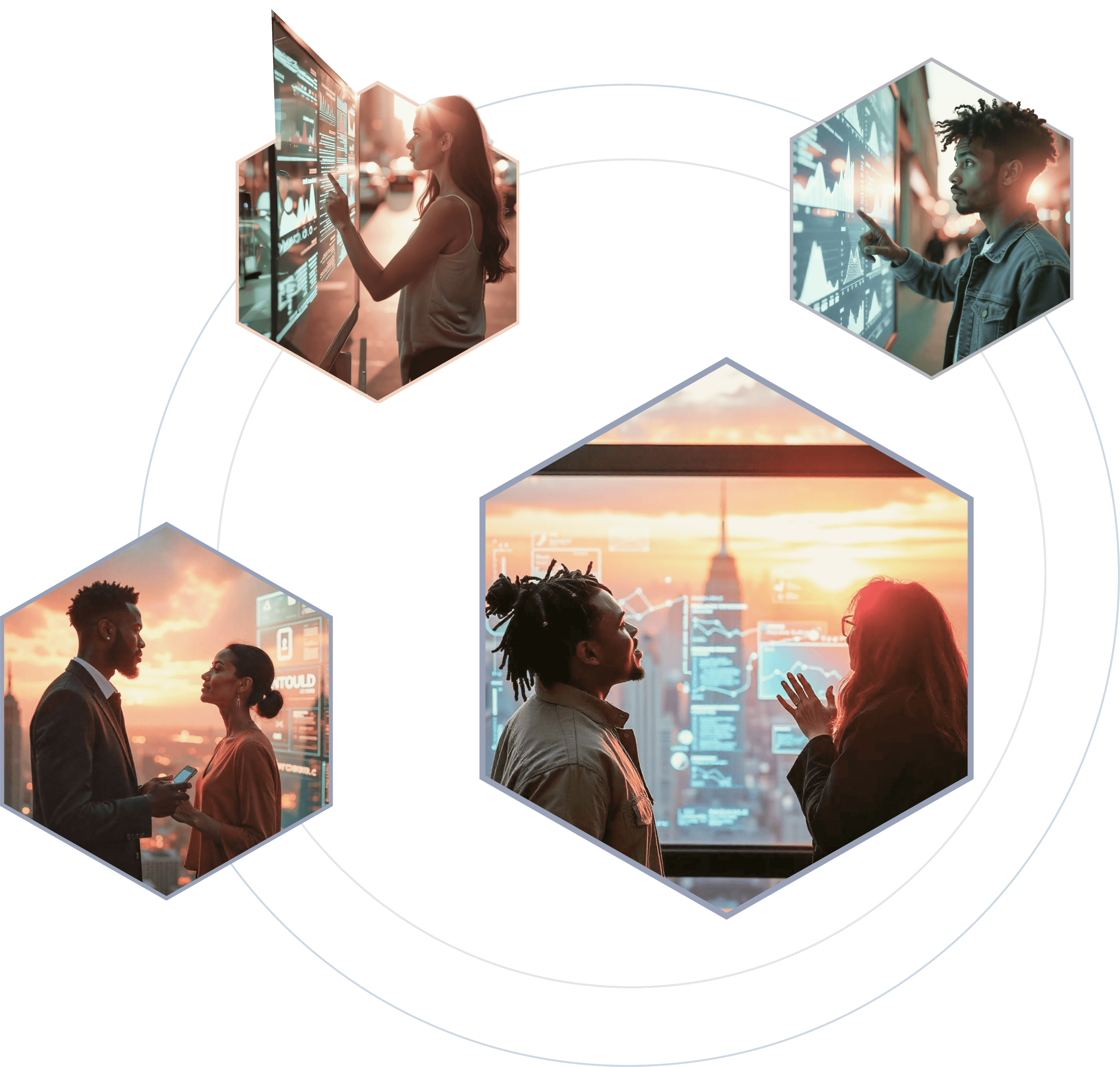
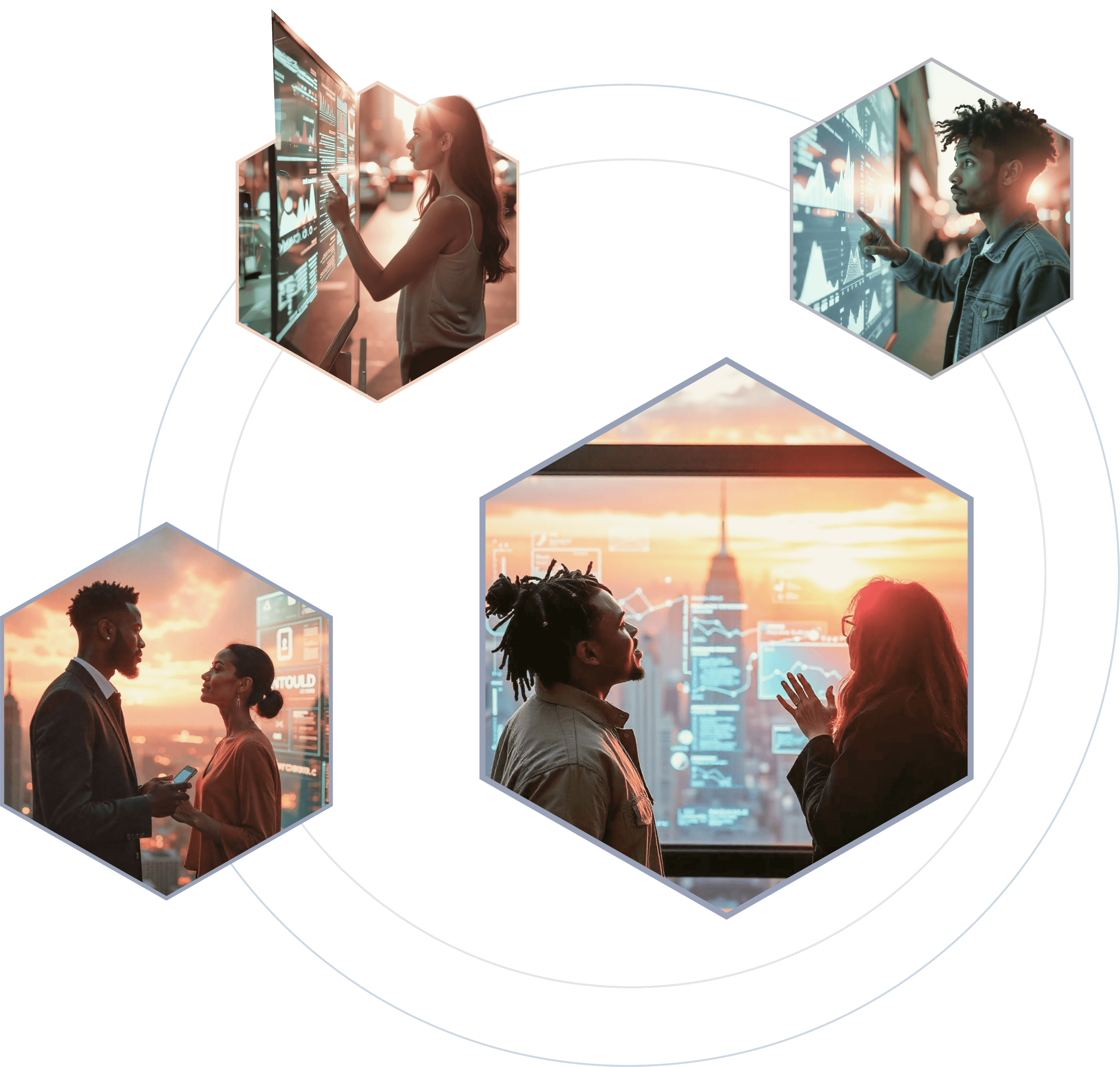
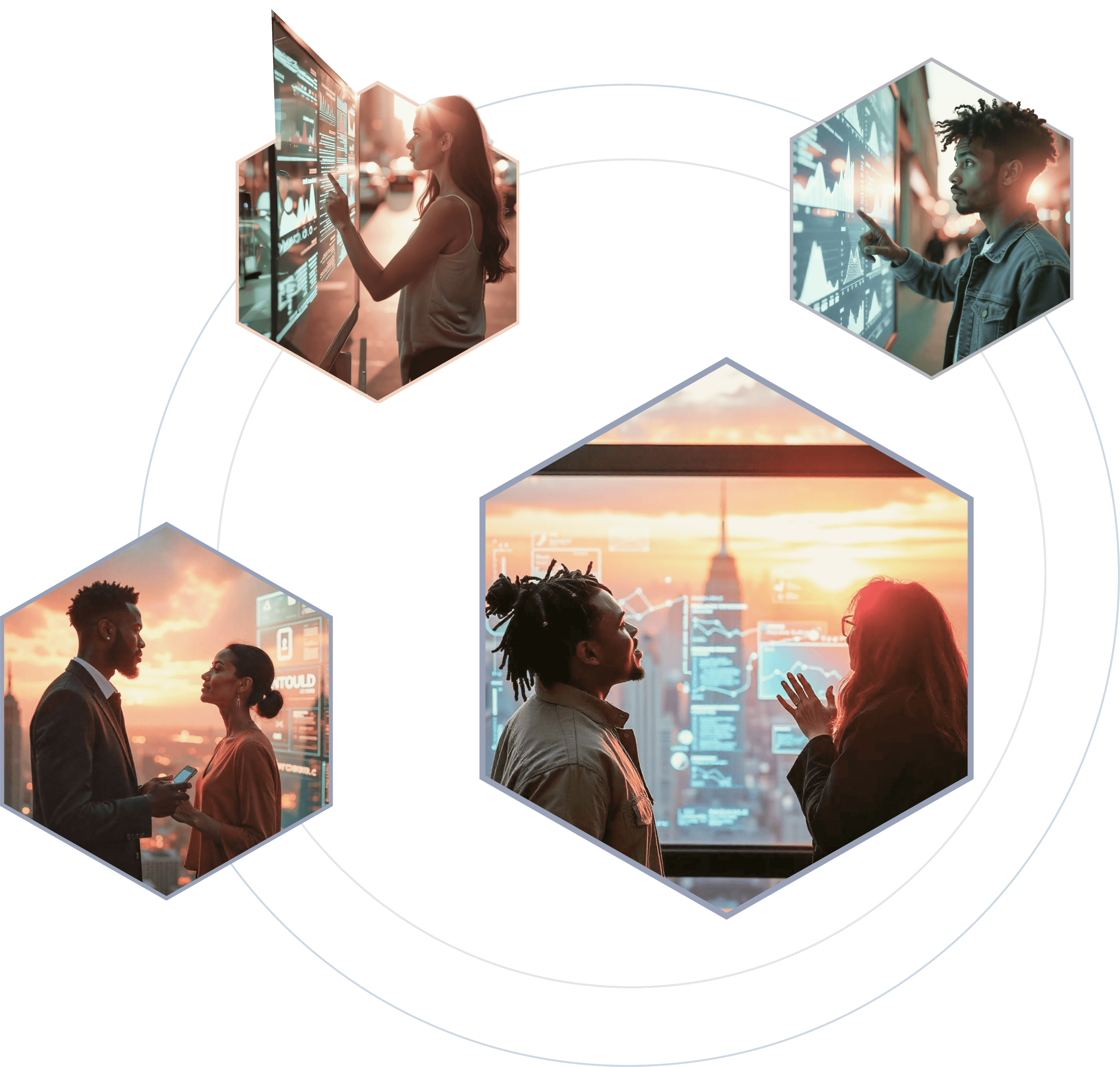
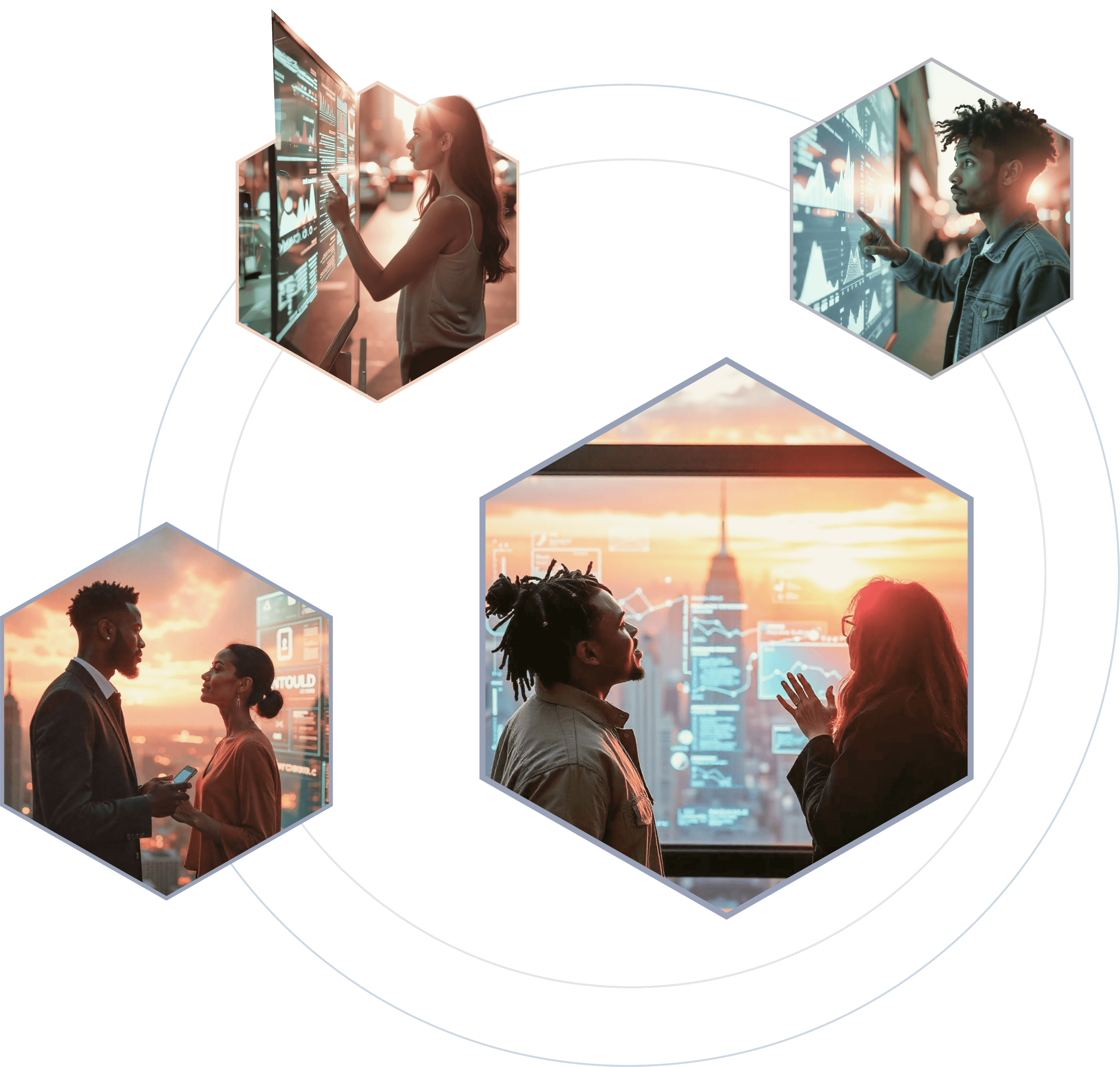
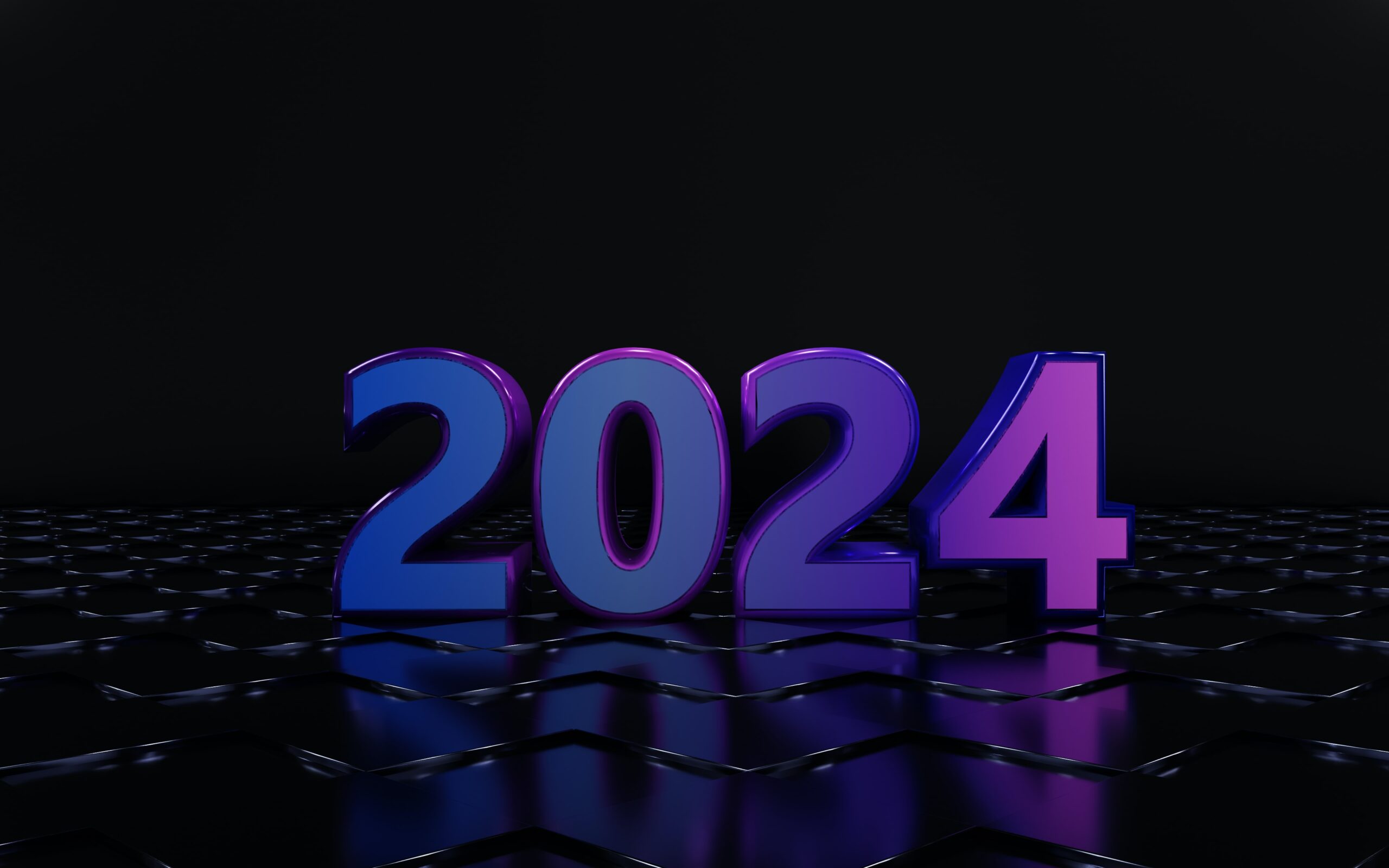
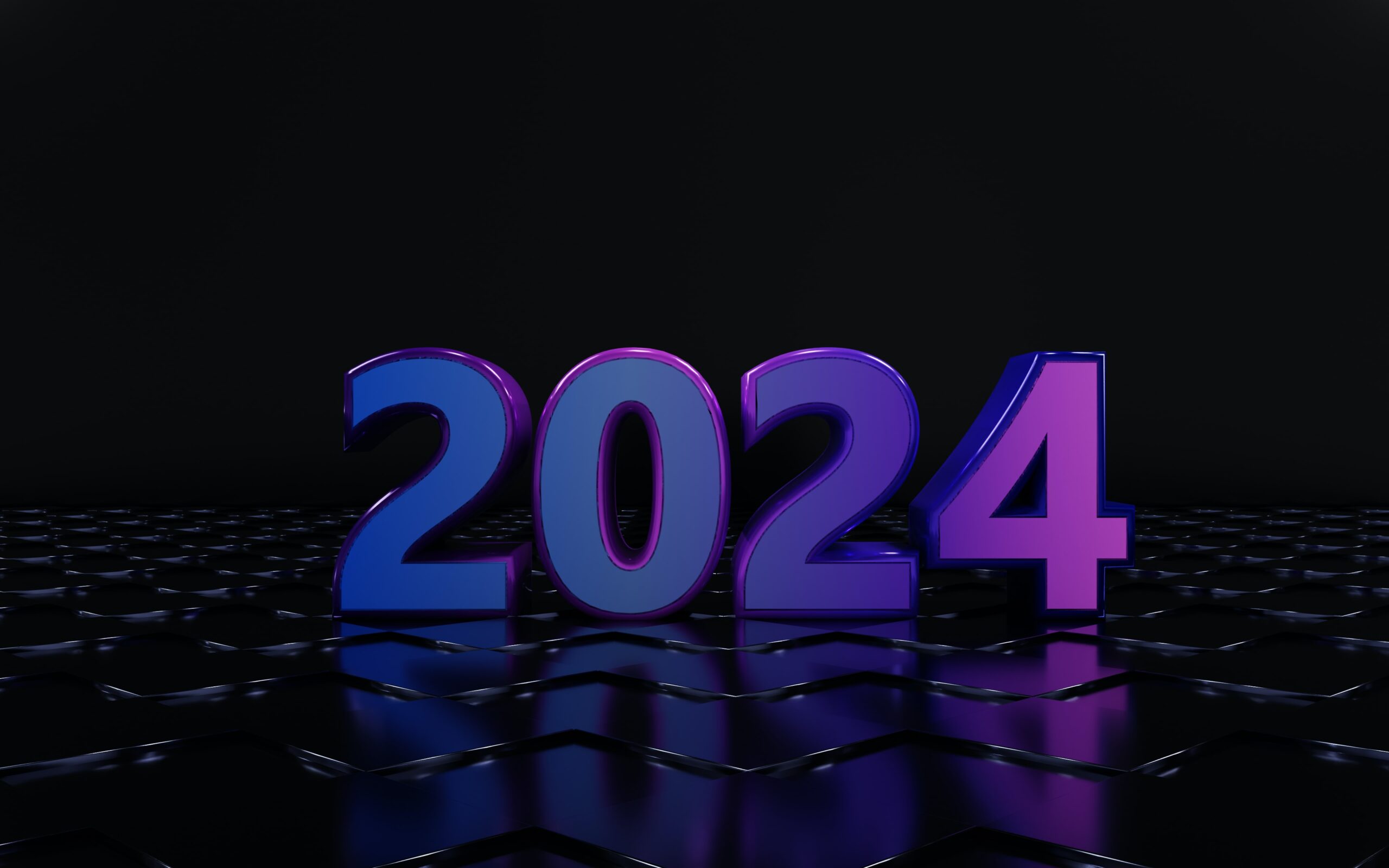
2024 Predictions: What Lies Ahead for Automation, AI, & Your Professional Future
2024 Predictions: What Lies Ahead for Automation, AI, & Your Professional Future
2024 Predictions: What Lies Ahead for Automation, AI, & Your Professional Future
2024 Predictions: What Lies Ahead for Automation, AI, & Your Professional Future
Dec 12, 2023
Dec 12, 2023
Explore
Explore
Blog
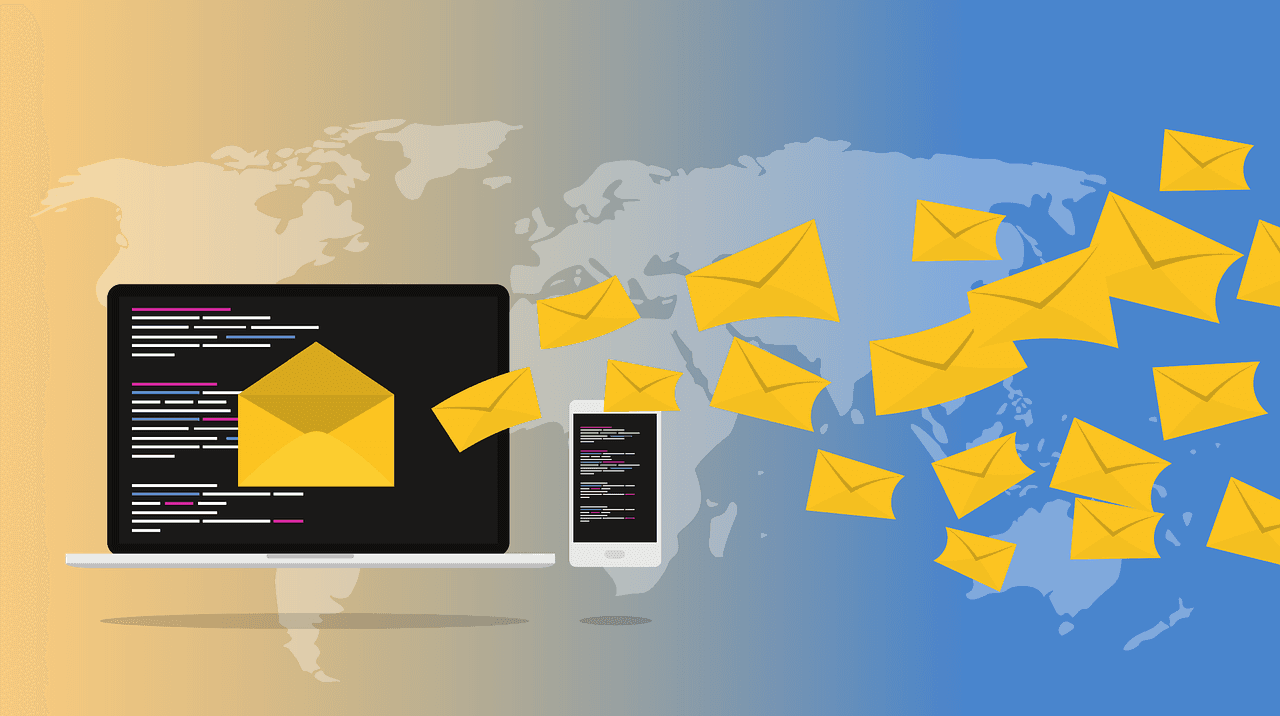
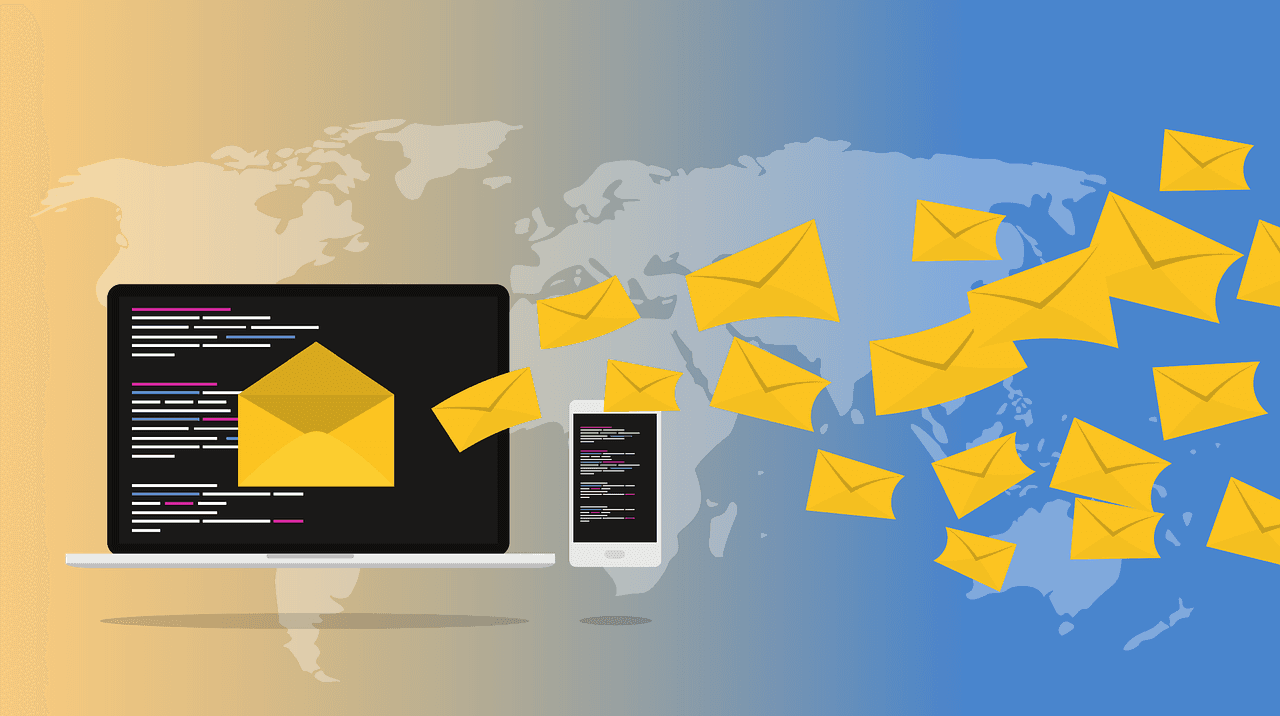
A Game-Changer in Email Marketing is Coming: Key Updates to Know
A Game-Changer in Email Marketing is Coming: Key Updates to Know
A Game-Changer in Email Marketing is Coming: Key Updates to Know
A Game-Changer in Email Marketing is Coming: Key Updates to Know
Dec 1, 2023
Dec 1, 2023
Explore
Explore
Blog
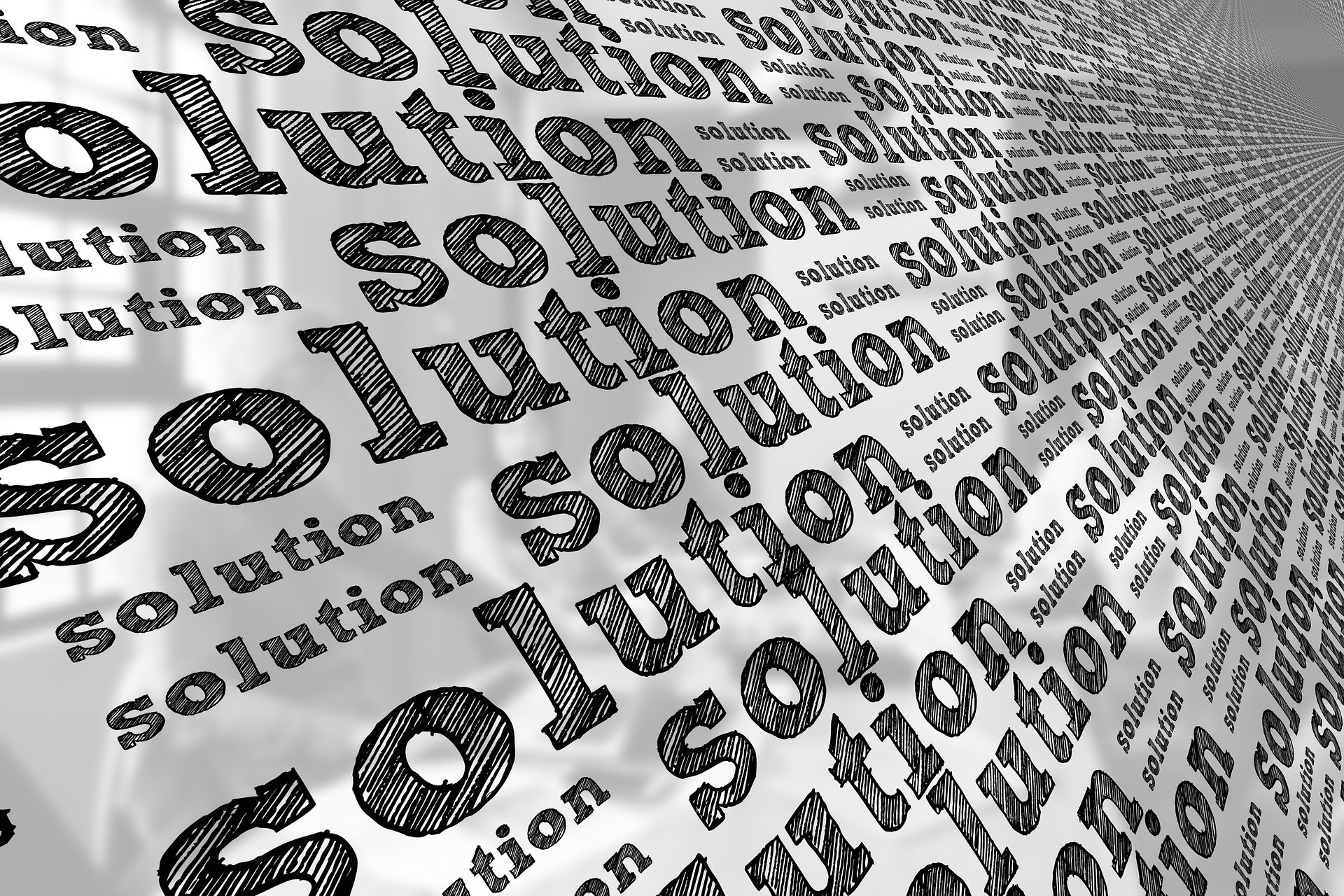
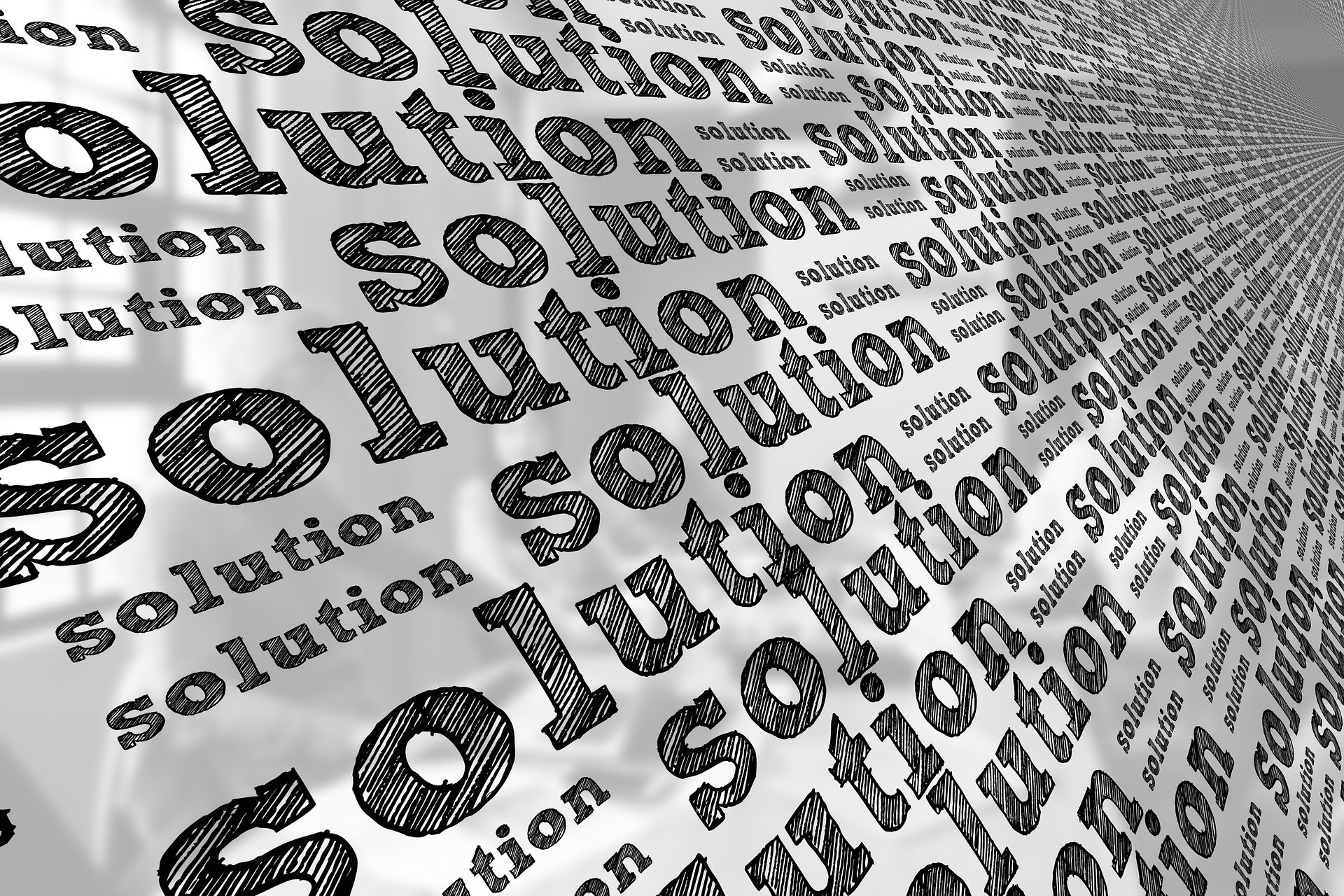
AI-Generated Content: Are You Seeing Results?
AI-Generated Content: Are You Seeing Results?
AI-Generated Content: Are You Seeing Results?
AI-Generated Content: Are You Seeing Results?
Apr 11, 2023
Apr 11, 2023
Explore
Explore
Blog
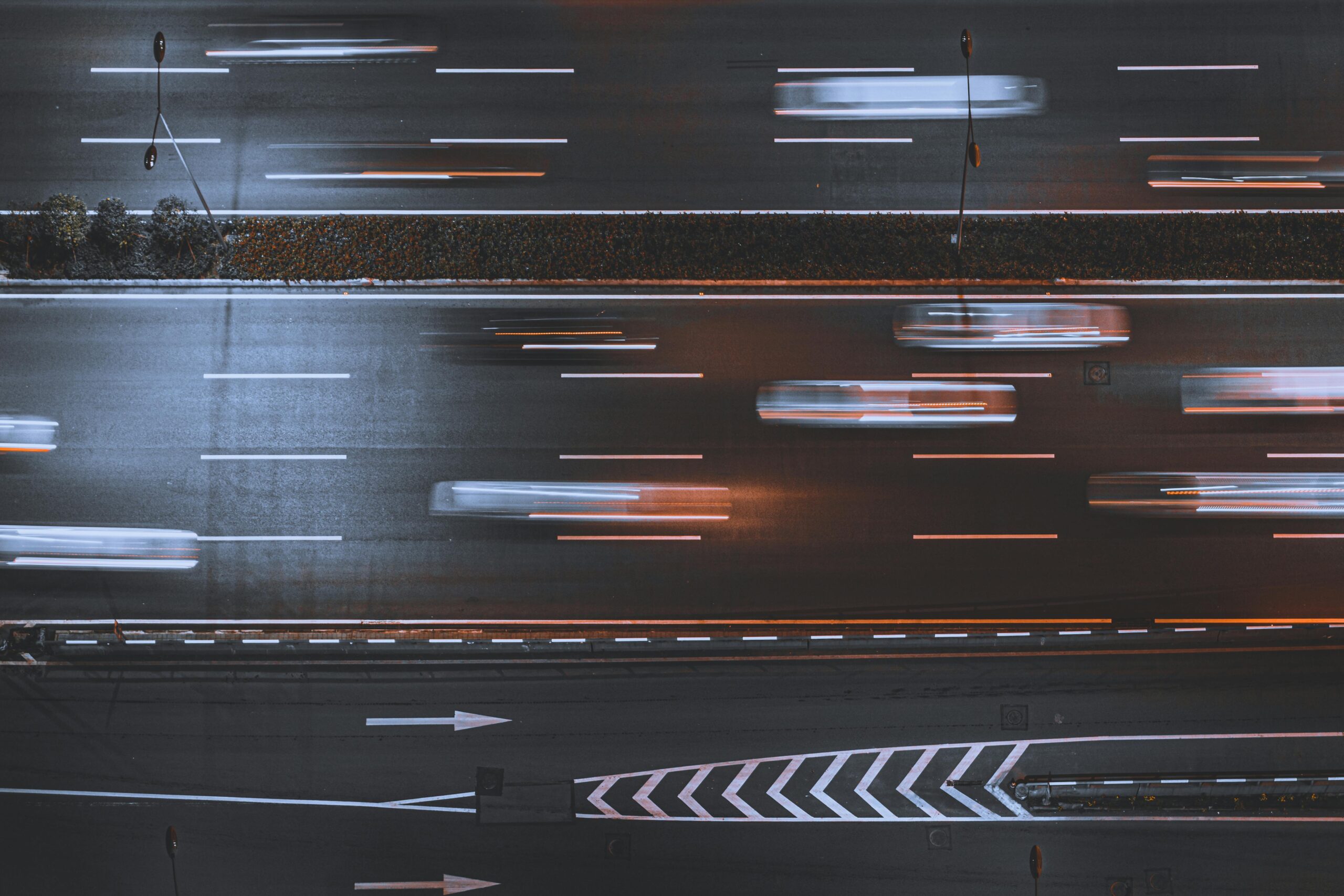
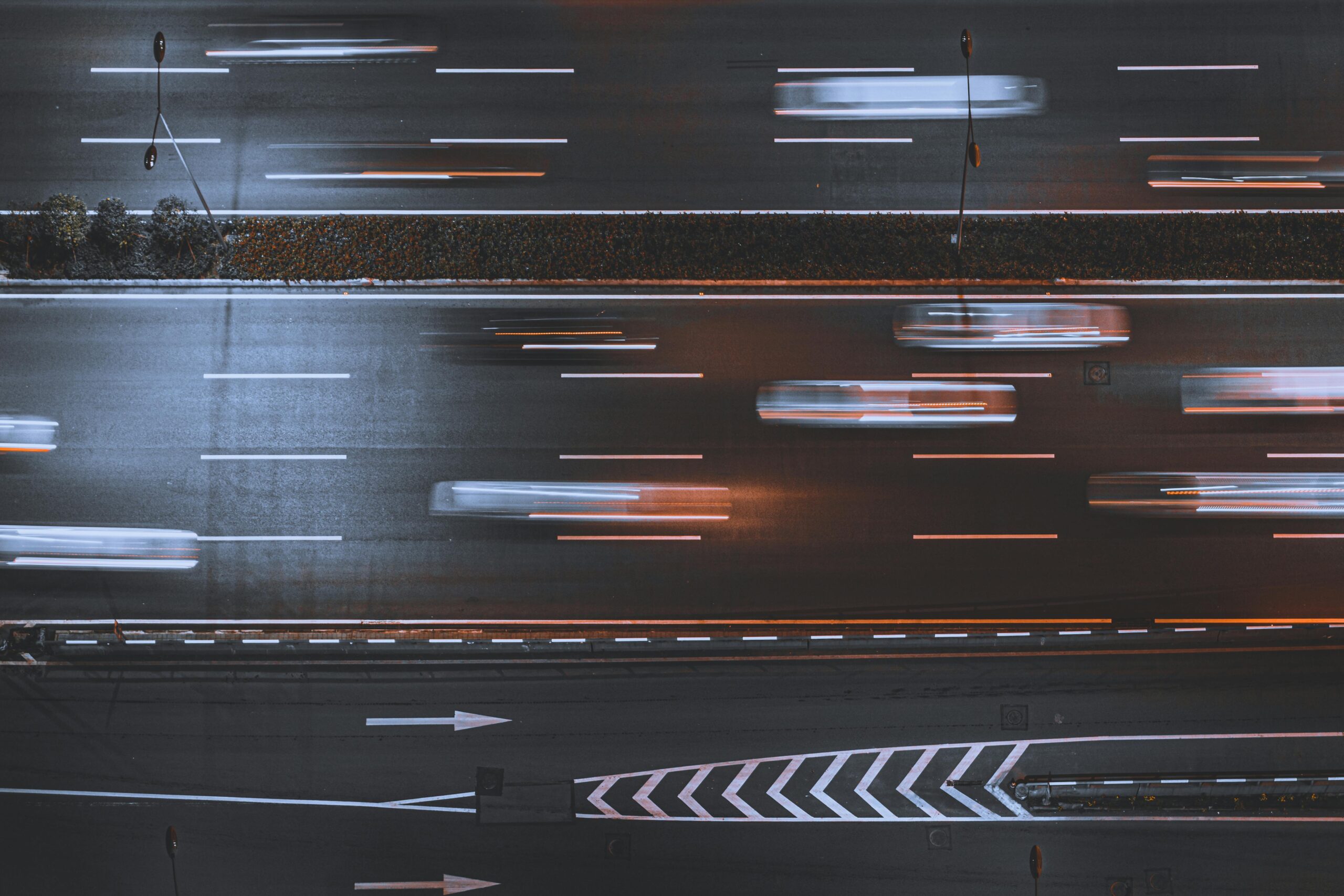
AI’s future impact on marketing will include a lot more personalization
AI’s future impact on marketing will include a lot more personalization
AI’s future impact on marketing will include a lot more personalization
AI’s future impact on marketing will include a lot more personalization
Mar 22, 2024
Mar 22, 2024
Explore
Explore
Blog
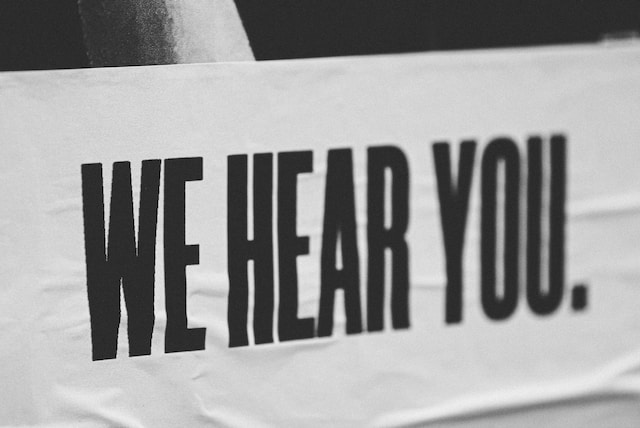
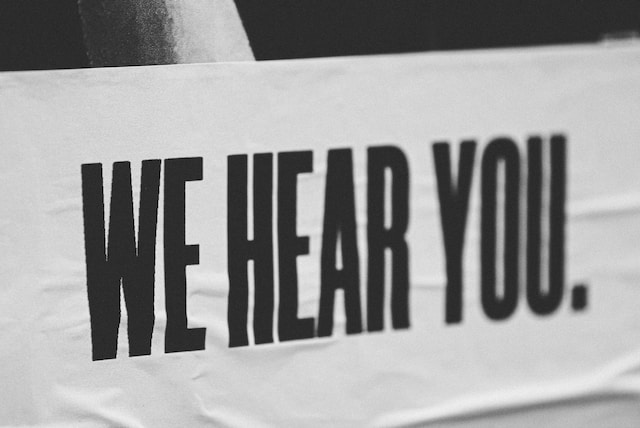
Are You Using the Right Data?
Are You Using the Right Data?
Are You Using the Right Data?
Are You Using the Right Data?
May 4, 2023
May 4, 2023
Explore
Explore
Blog
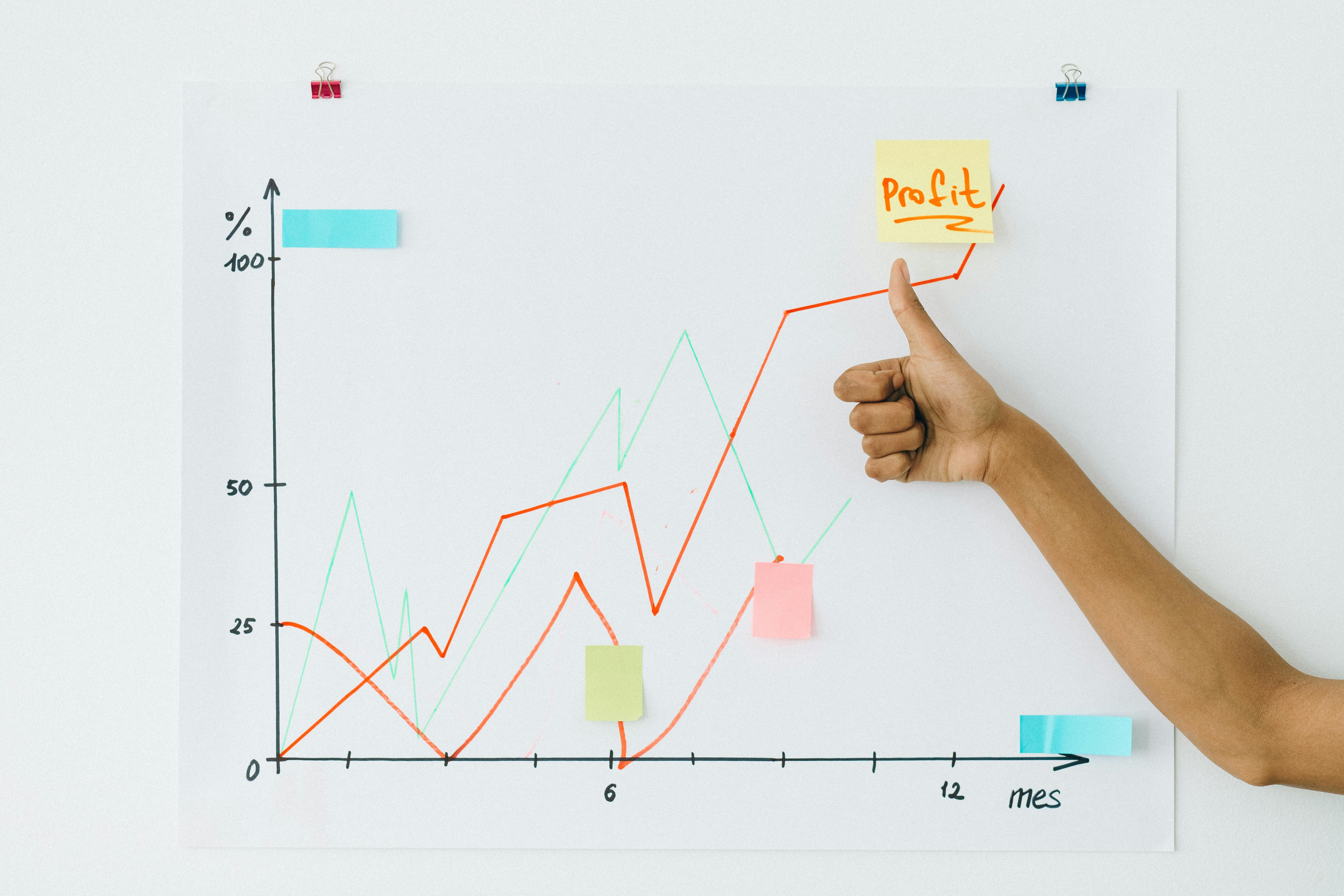
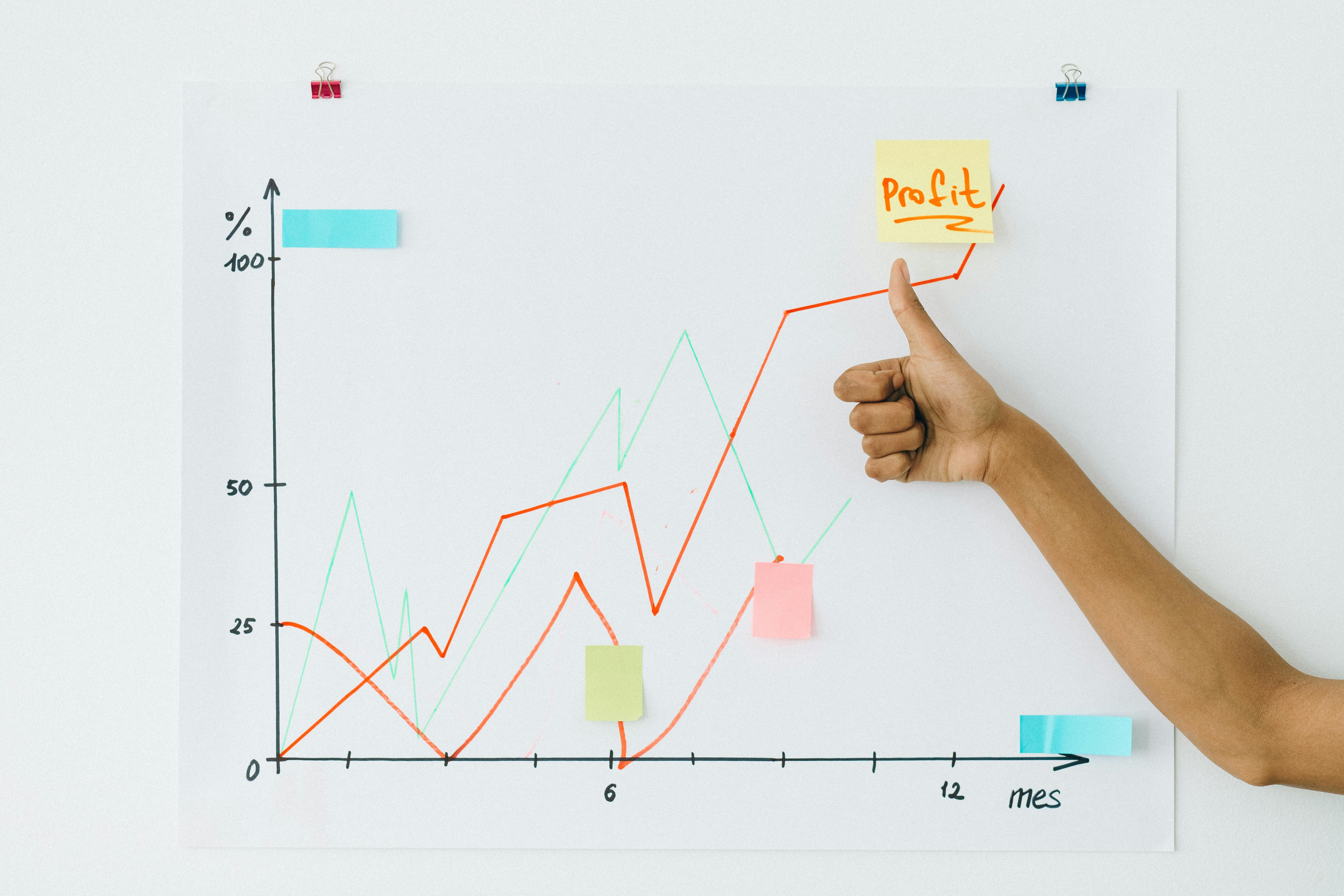
Case Study: How Lead Scores Reduced Costs and Increased Sales
Case Study: How Lead Scores Reduced Costs and Increased Sales
Case Study: How Lead Scores Reduced Costs and Increased Sales
Case Study: How Lead Scores Reduced Costs and Increased Sales
Aug 31, 2022
Aug 31, 2022
Explore
Explore
Case Study
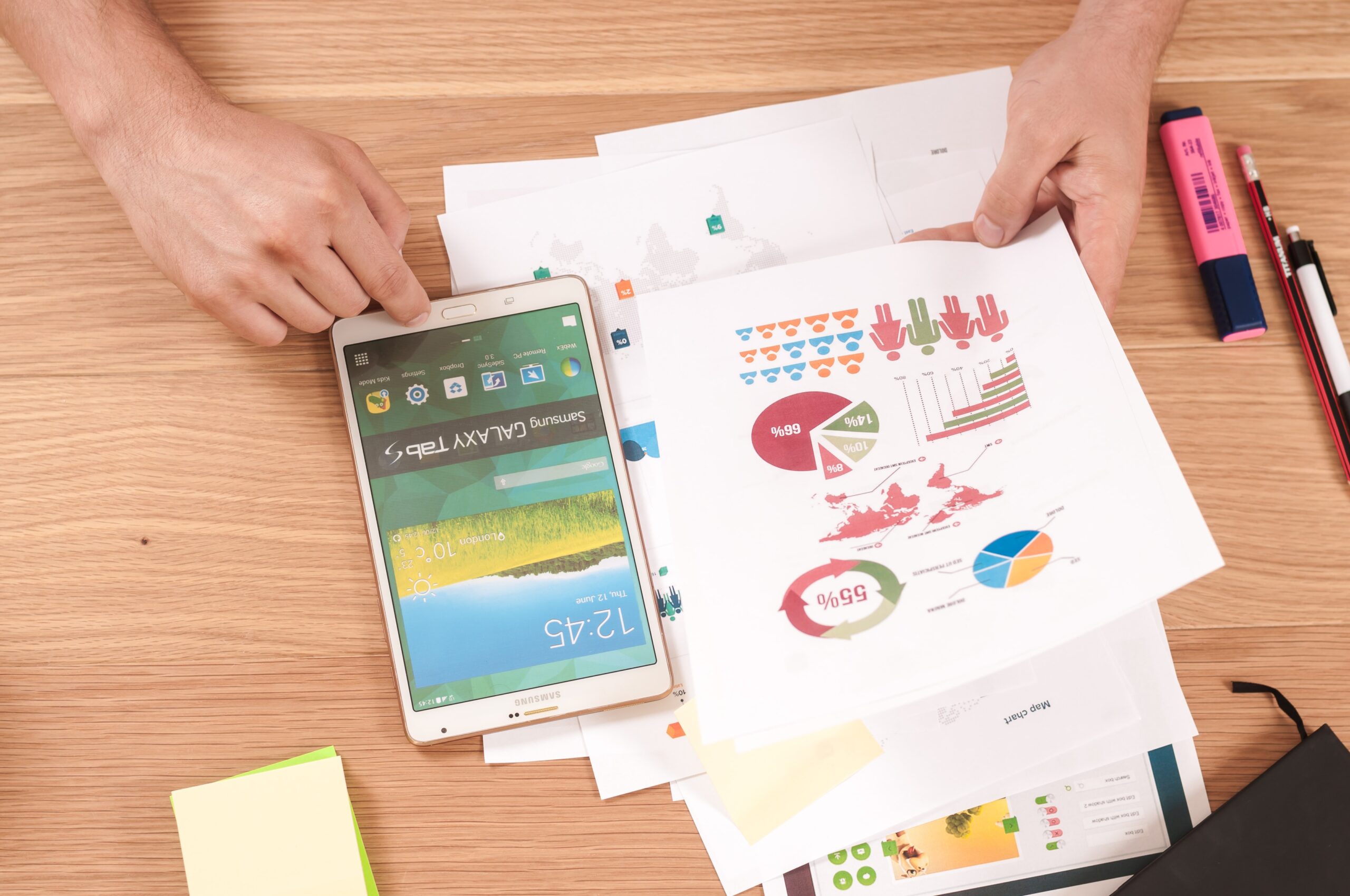
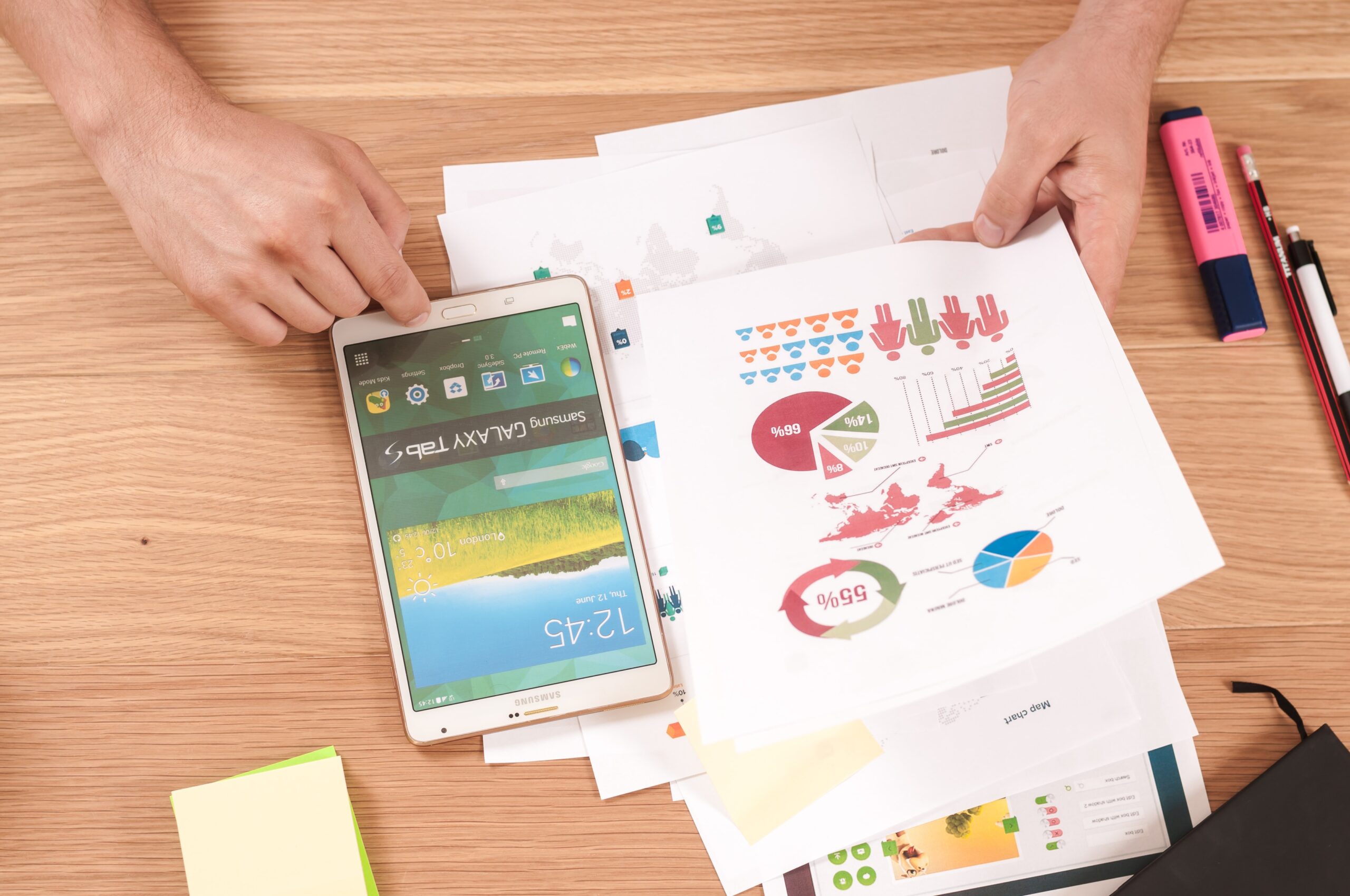
Case Study: Using AI for Segmentation and Personas
Case Study: Using AI for Segmentation and Personas
Case Study: Using AI for Segmentation and Personas
Case Study: Using AI for Segmentation and Personas
Oct 7, 2022
Oct 7, 2022
Explore
Explore
Case Study
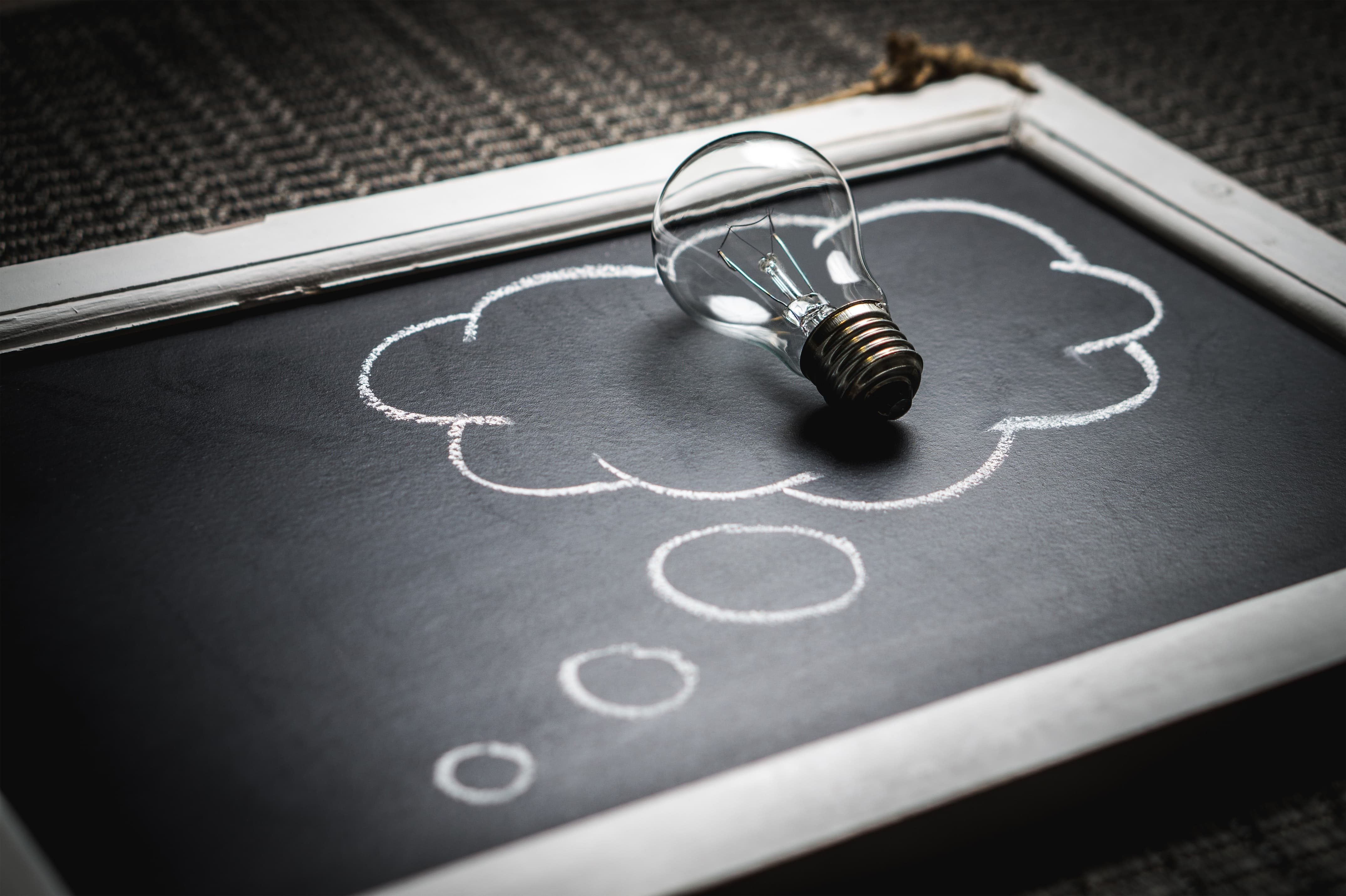
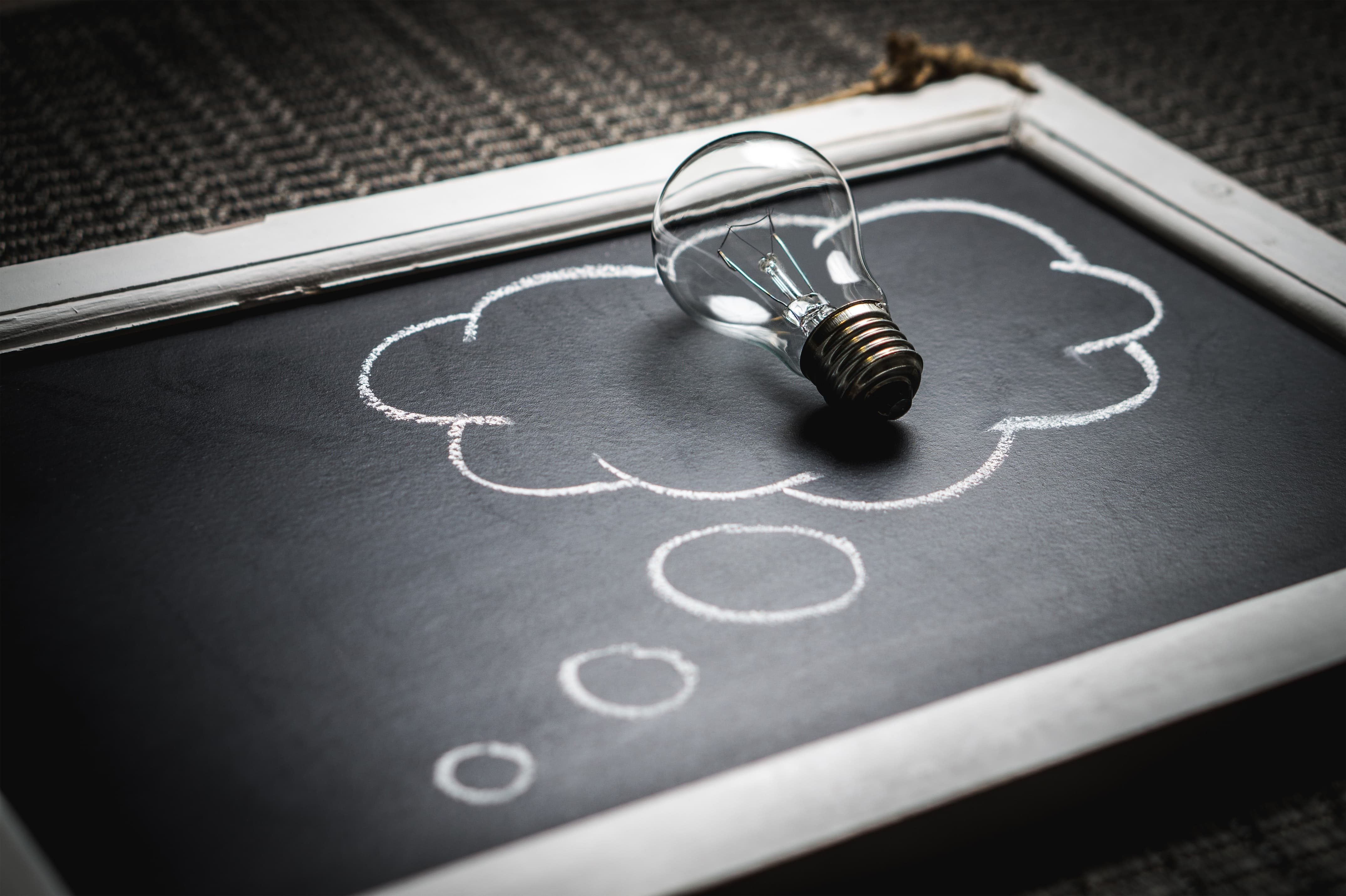
Case Study: Why We Built an App to Make Personalization Easier
Case Study: Why We Built an App to Make Personalization Easier
Case Study: Why We Built an App to Make Personalization Easier
Case Study: Why We Built an App to Make Personalization Easier
Jan 3, 2019
Jan 3, 2019
Explore
Explore
Case Study
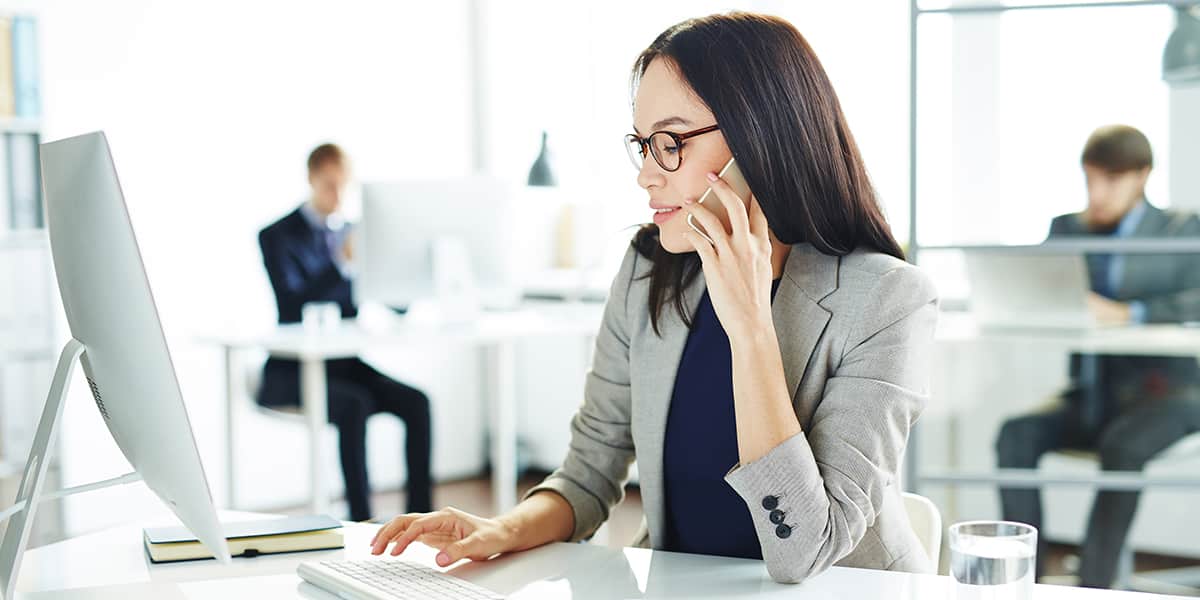
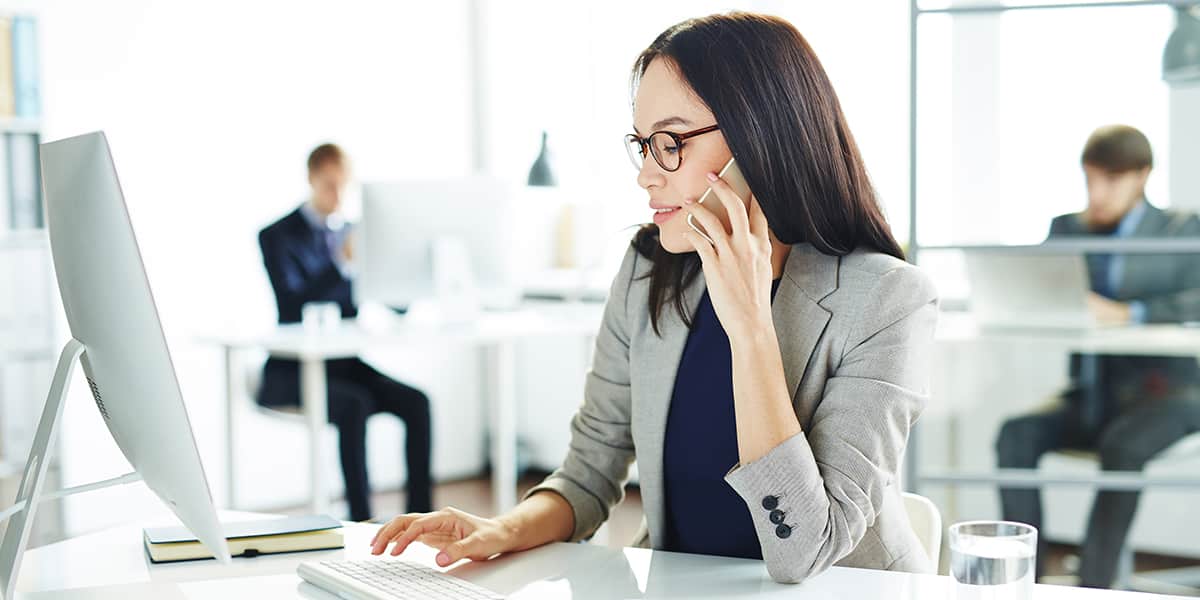
Cold Calling is Not Dead
Cold Calling is Not Dead
Cold Calling is Not Dead
Cold Calling is Not Dead
May 8, 2020
May 8, 2020
Explore
Explore
Blog
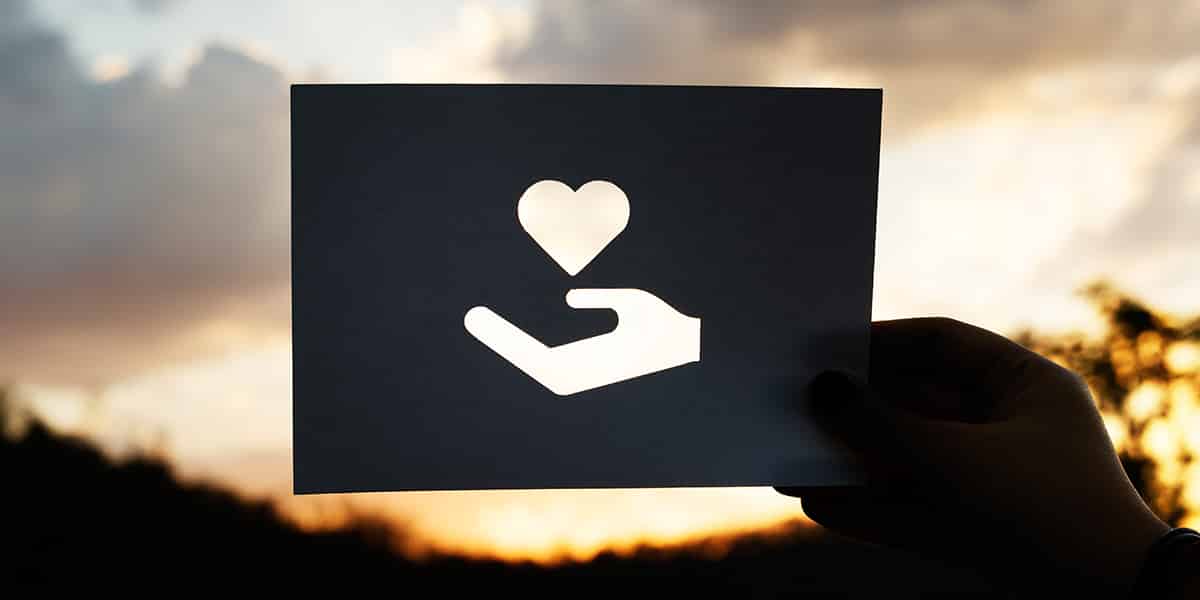
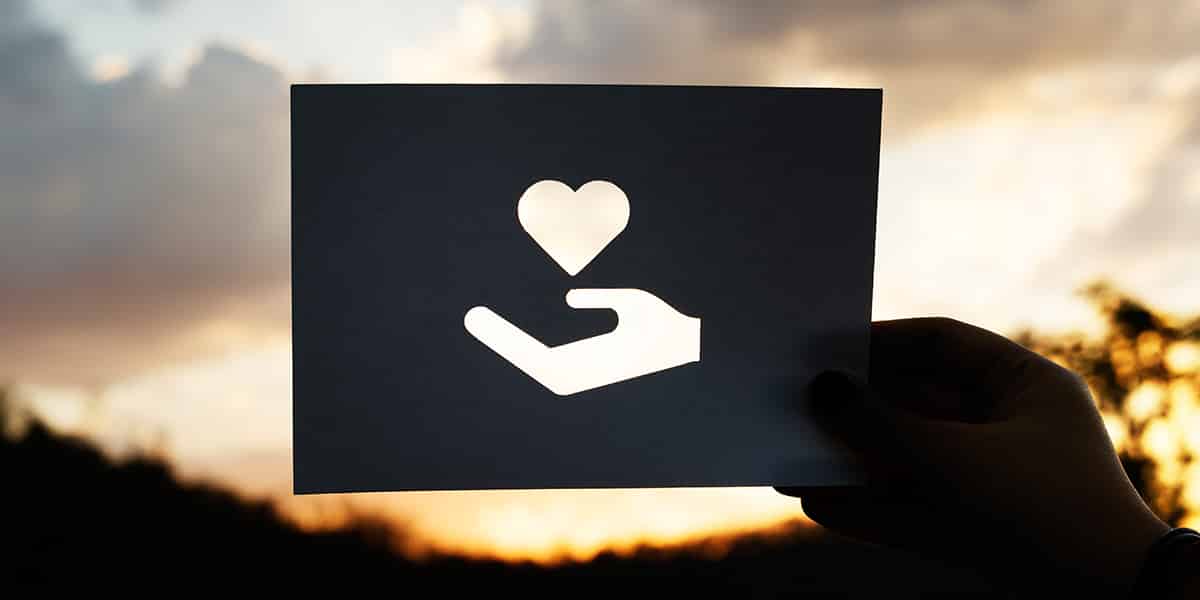
COVID-19 Marketing: Goodwill Marketing is the Current Brand Marketing
COVID-19 Marketing: Goodwill Marketing is the Current Brand Marketing
COVID-19 Marketing: Goodwill Marketing is the Current Brand Marketing
COVID-19 Marketing: Goodwill Marketing is the Current Brand Marketing
Apr 18, 2020
Apr 18, 2020
Explore
Explore
Blog
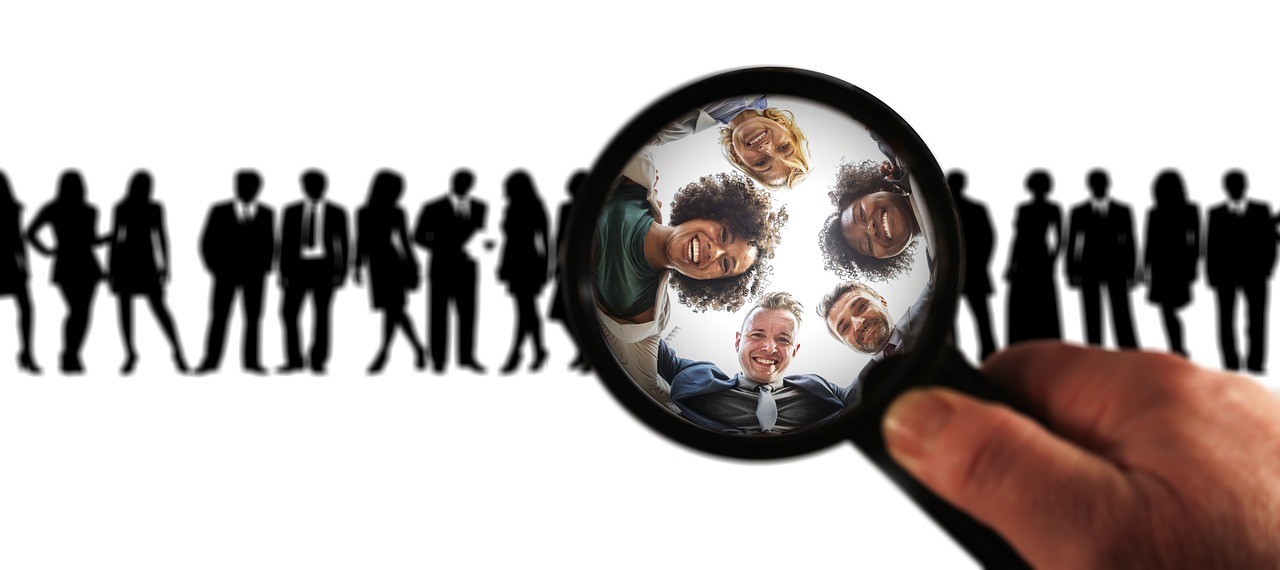
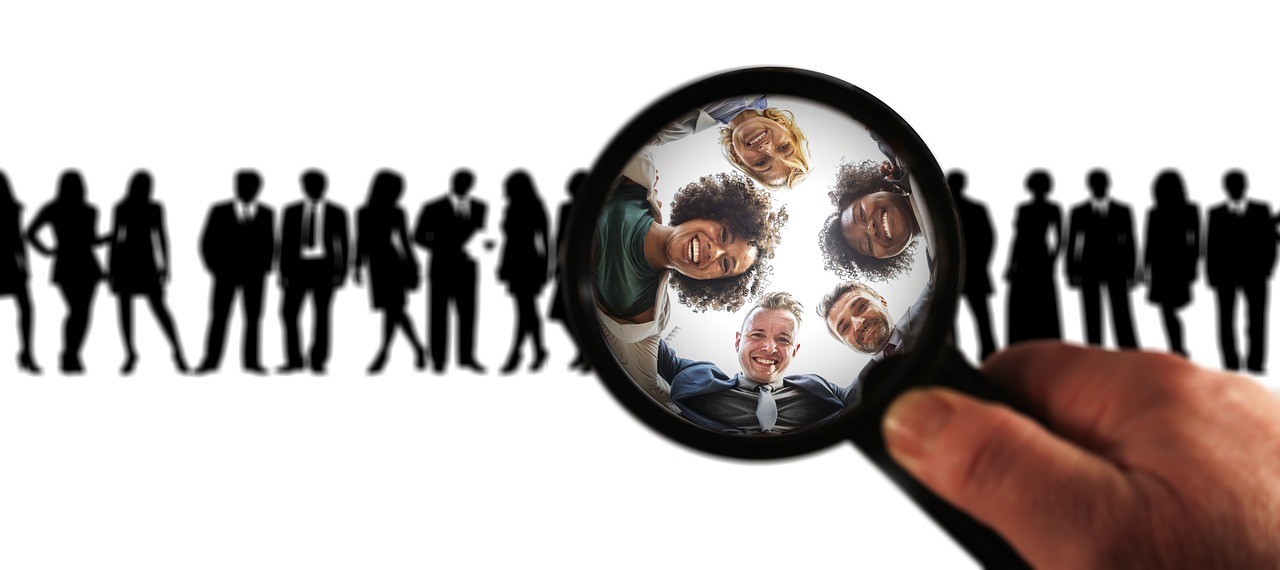
Get Inside Your Audience’s Head: Discovering Customer Affinities with AI
Get Inside Your Audience’s Head: Discovering Customer Affinities with AI
Get Inside Your Audience’s Head: Discovering Customer Affinities with AI
Get Inside Your Audience’s Head: Discovering Customer Affinities with AI
Jul 28, 2023
Jul 28, 2023
Explore
Explore
Blog
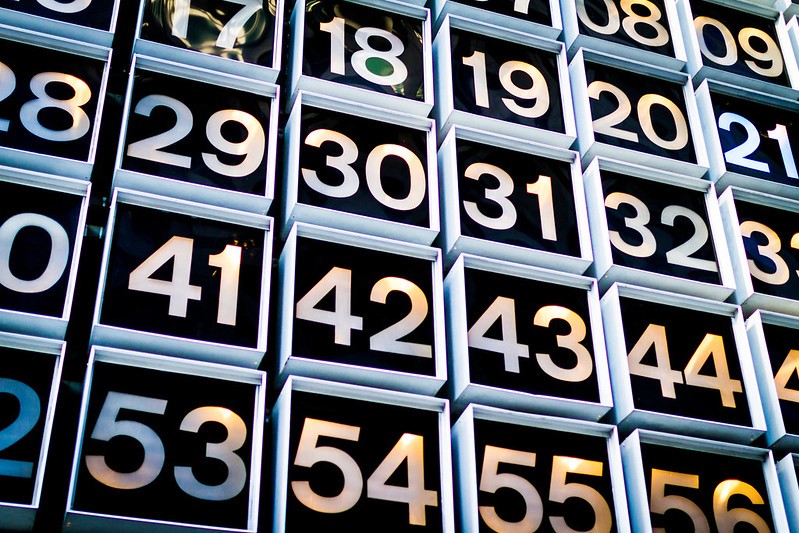
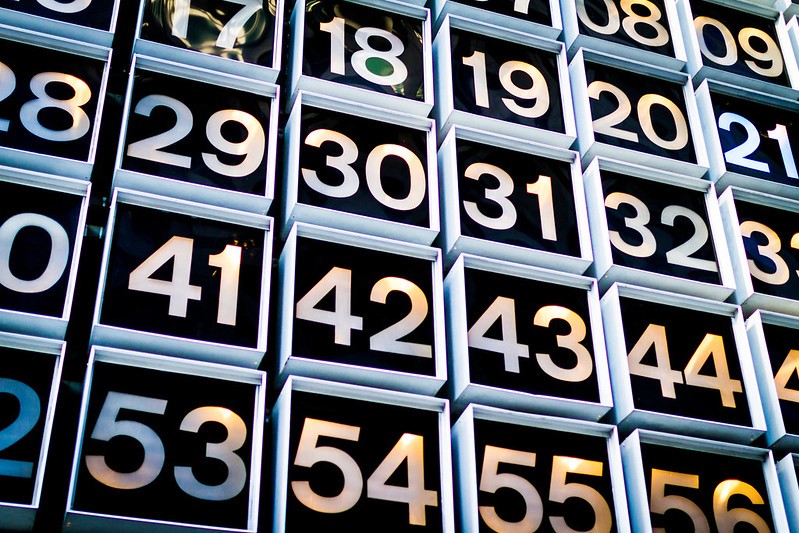
How AI-Based Lead Scores Increase Conversions and Revenue
How AI-Based Lead Scores Increase Conversions and Revenue
How AI-Based Lead Scores Increase Conversions and Revenue
How AI-Based Lead Scores Increase Conversions and Revenue
Apr 5, 2023
Apr 5, 2023
Explore
Explore
Blog
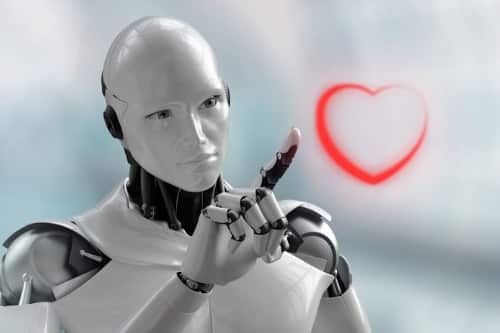
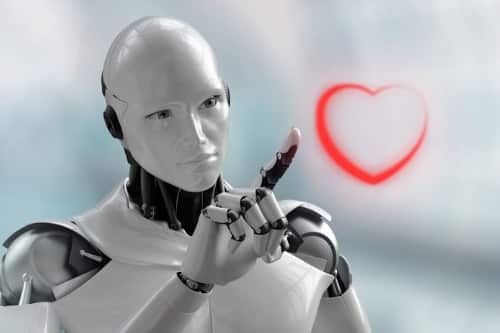
How marketing and sales AI can make your brand more human
How marketing and sales AI can make your brand more human
How marketing and sales AI can make your brand more human
How marketing and sales AI can make your brand more human
Jun 5, 2020
Jun 5, 2020
Explore
Explore
Blog
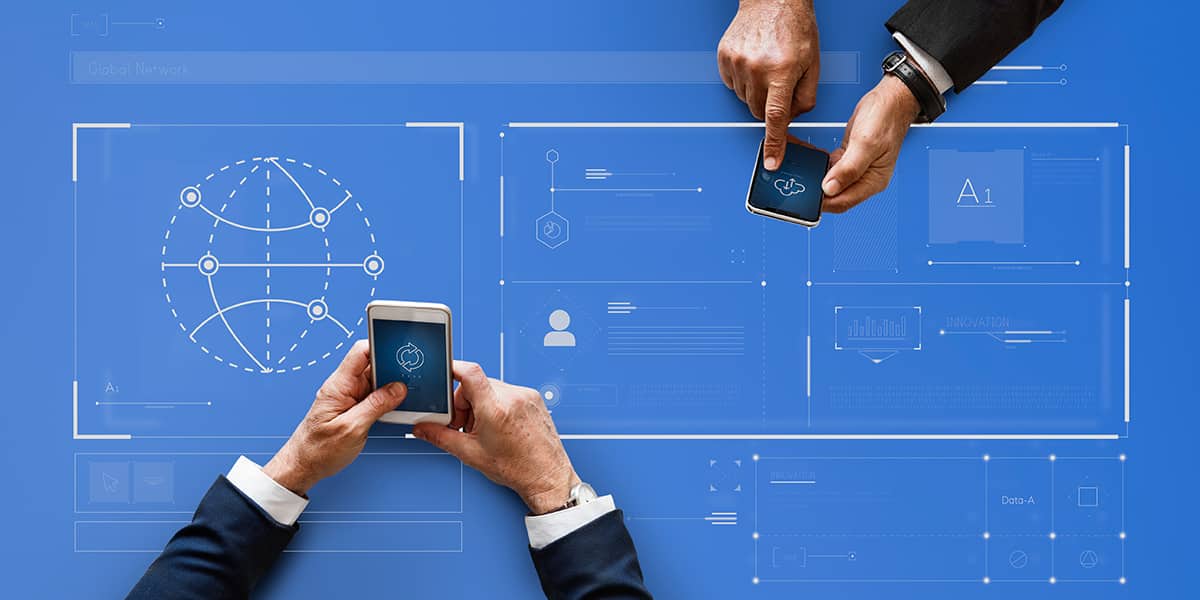
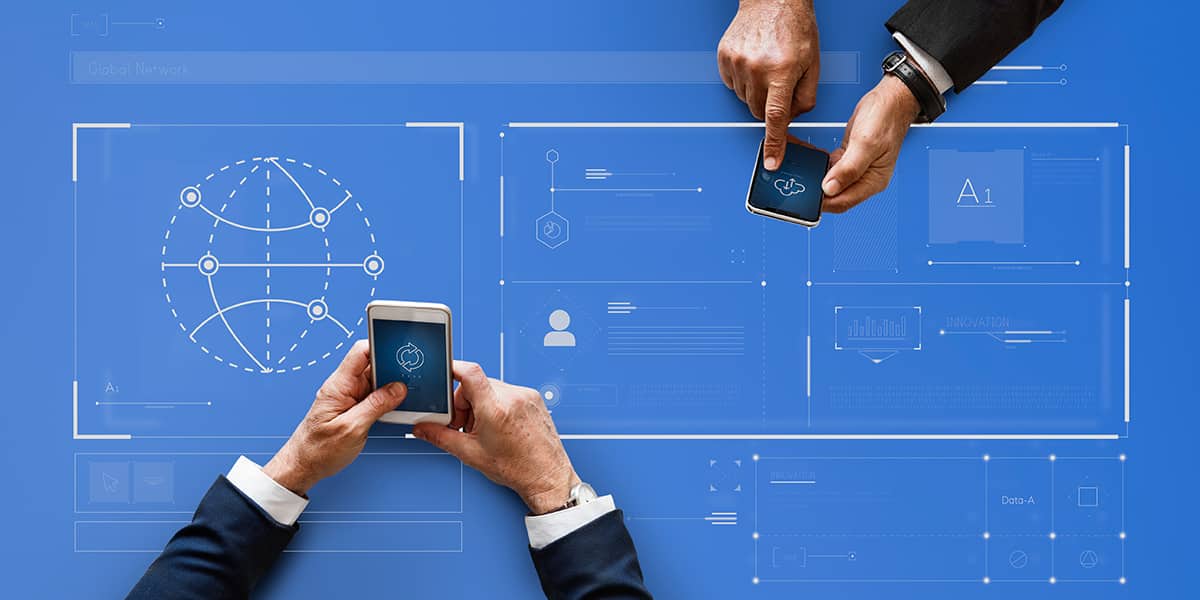
Keeping the balance against AI biases
Keeping the balance against AI biases
Keeping the balance against AI biases
Keeping the balance against AI biases
May 1, 2020
May 1, 2020
Explore
Explore
Blog
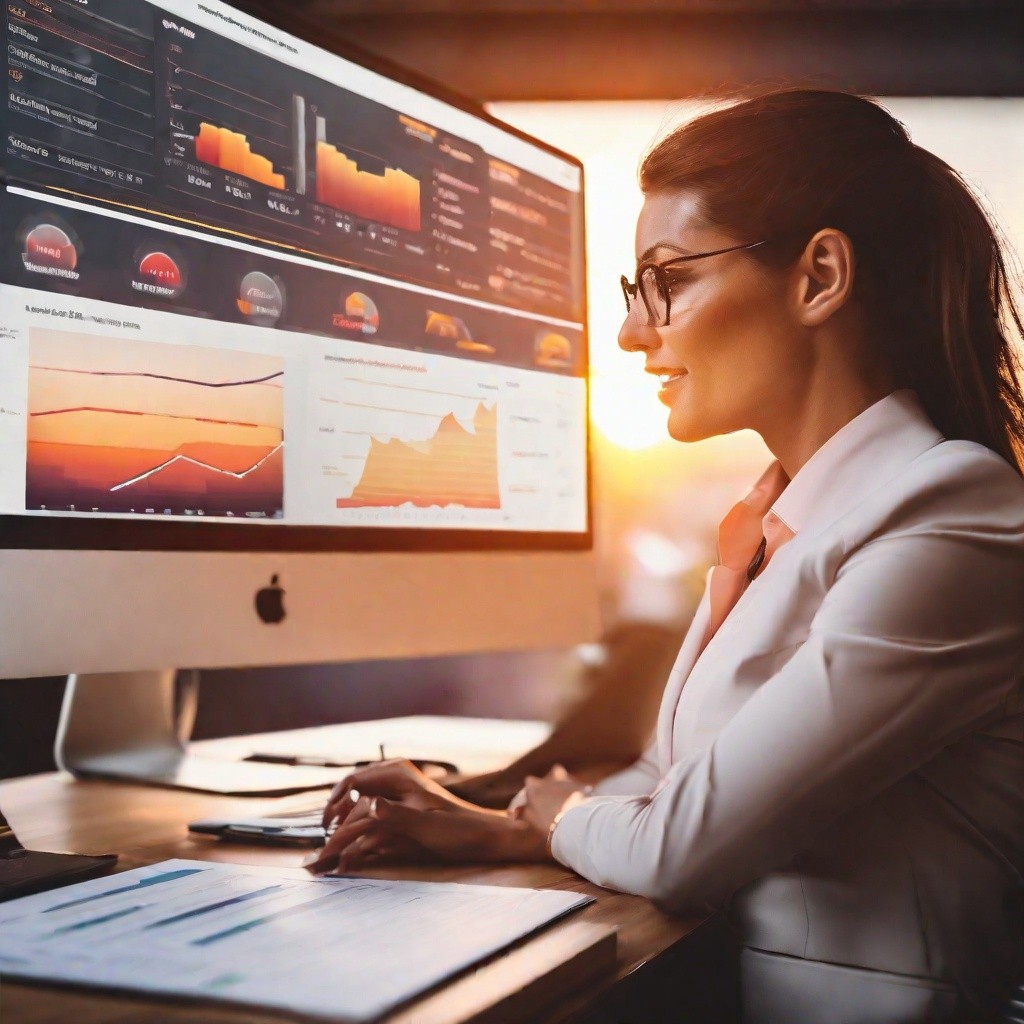
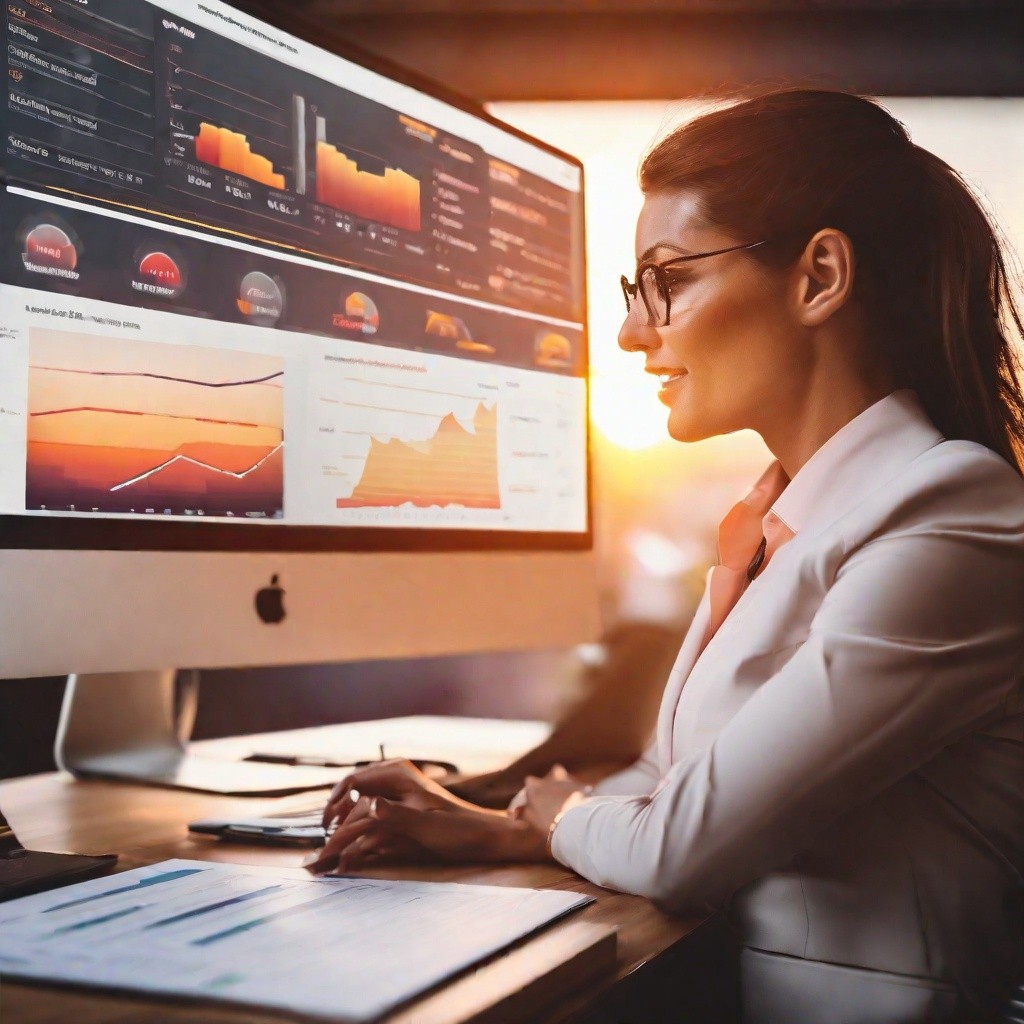
Key Strategies for Amplifying B2B Demand Generation
Key Strategies for Amplifying B2B Demand Generation
Key Strategies for Amplifying B2B Demand Generation
Key Strategies for Amplifying B2B Demand Generation
Dec 6, 2023
Dec 6, 2023
Explore
Explore
Blog
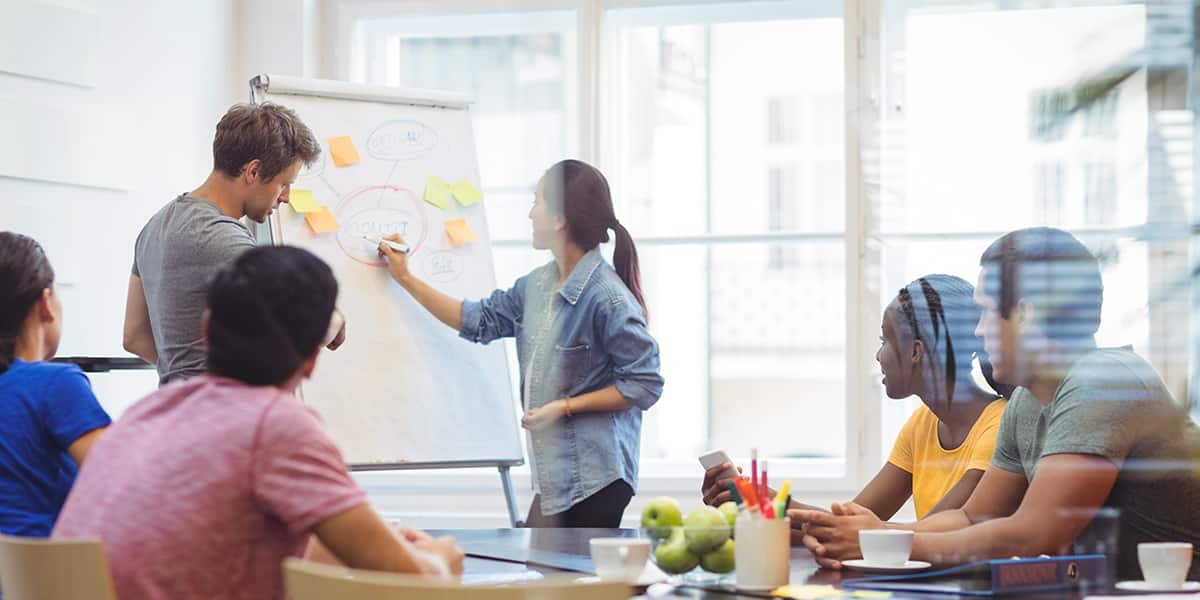
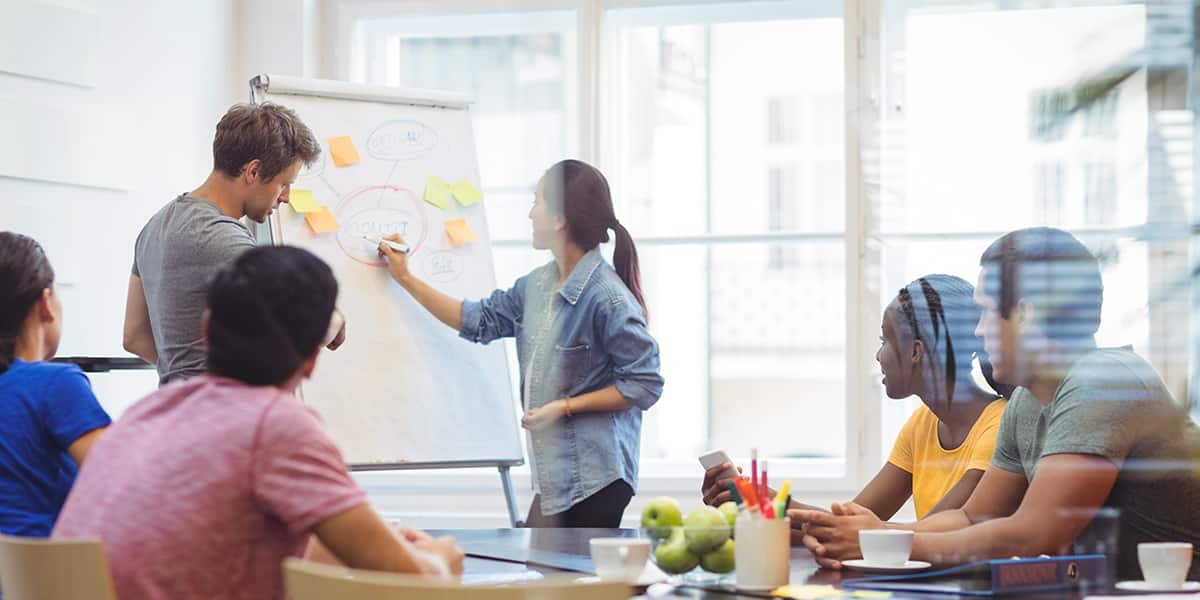
Marketing & Sales Beyond the Pandemic 101: Keep Building
Marketing & Sales Beyond the Pandemic 101: Keep Building
Marketing & Sales Beyond the Pandemic 101: Keep Building
Marketing & Sales Beyond the Pandemic 101: Keep Building
Apr 25, 2020
Apr 25, 2020
Explore
Explore
Blog
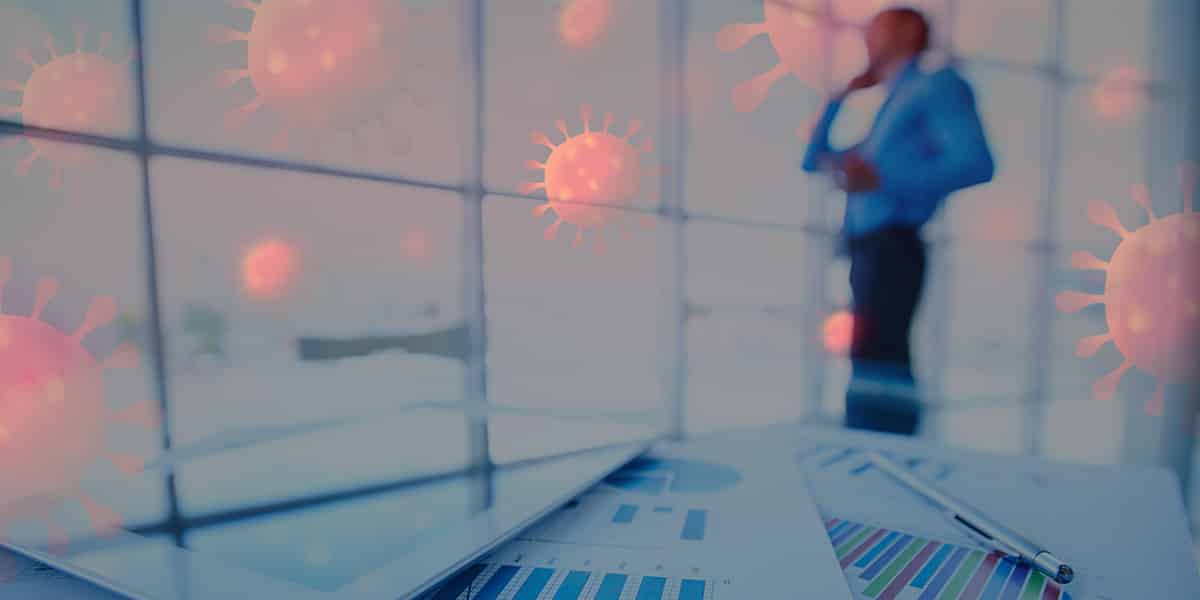
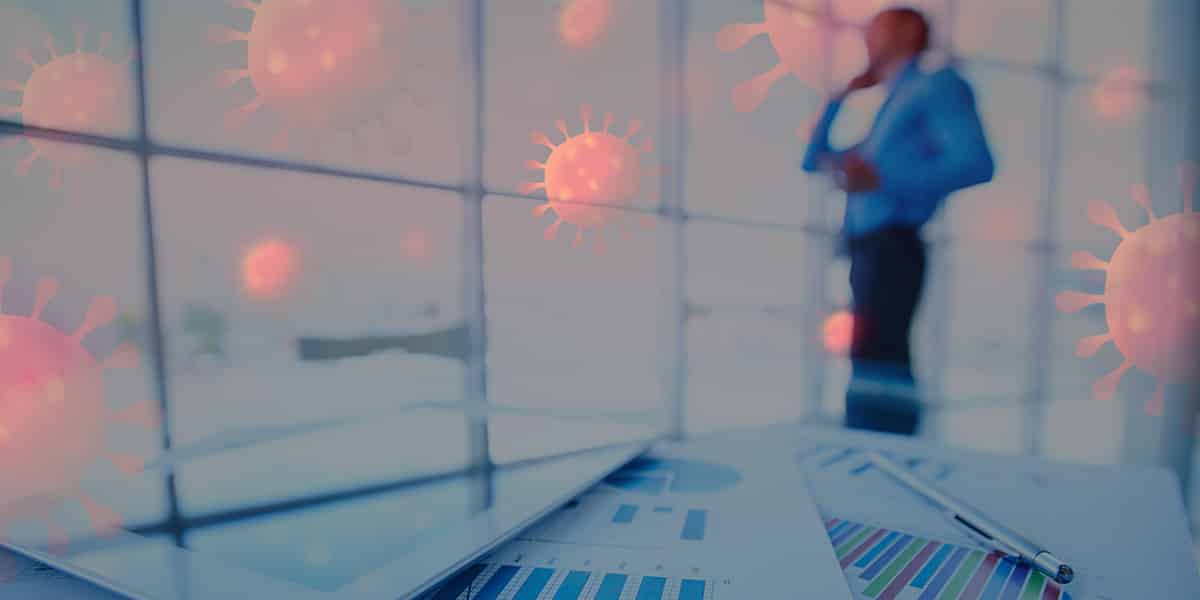
Marketing in the Time of COVID-19
Marketing in the Time of COVID-19
Marketing in the Time of COVID-19
Marketing in the Time of COVID-19
Apr 3, 2020
Apr 3, 2020
Explore
Explore
Blog
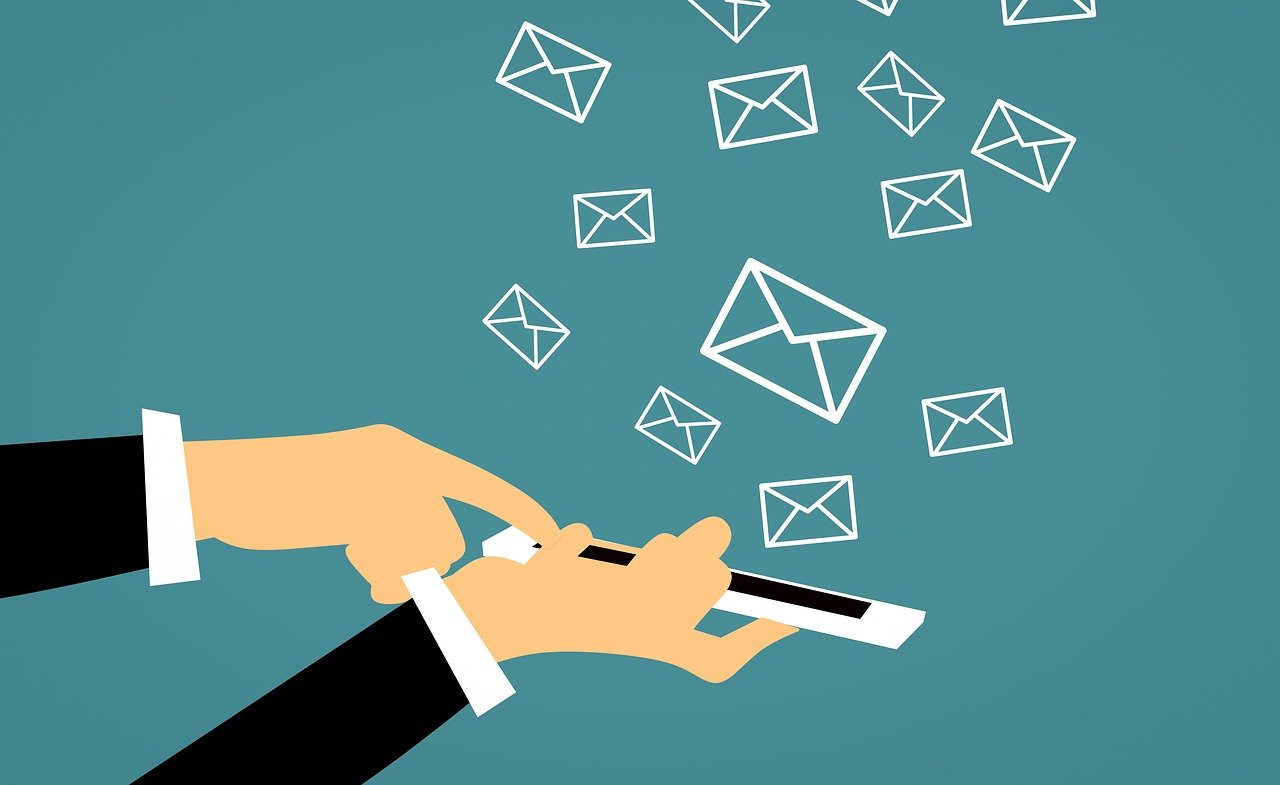
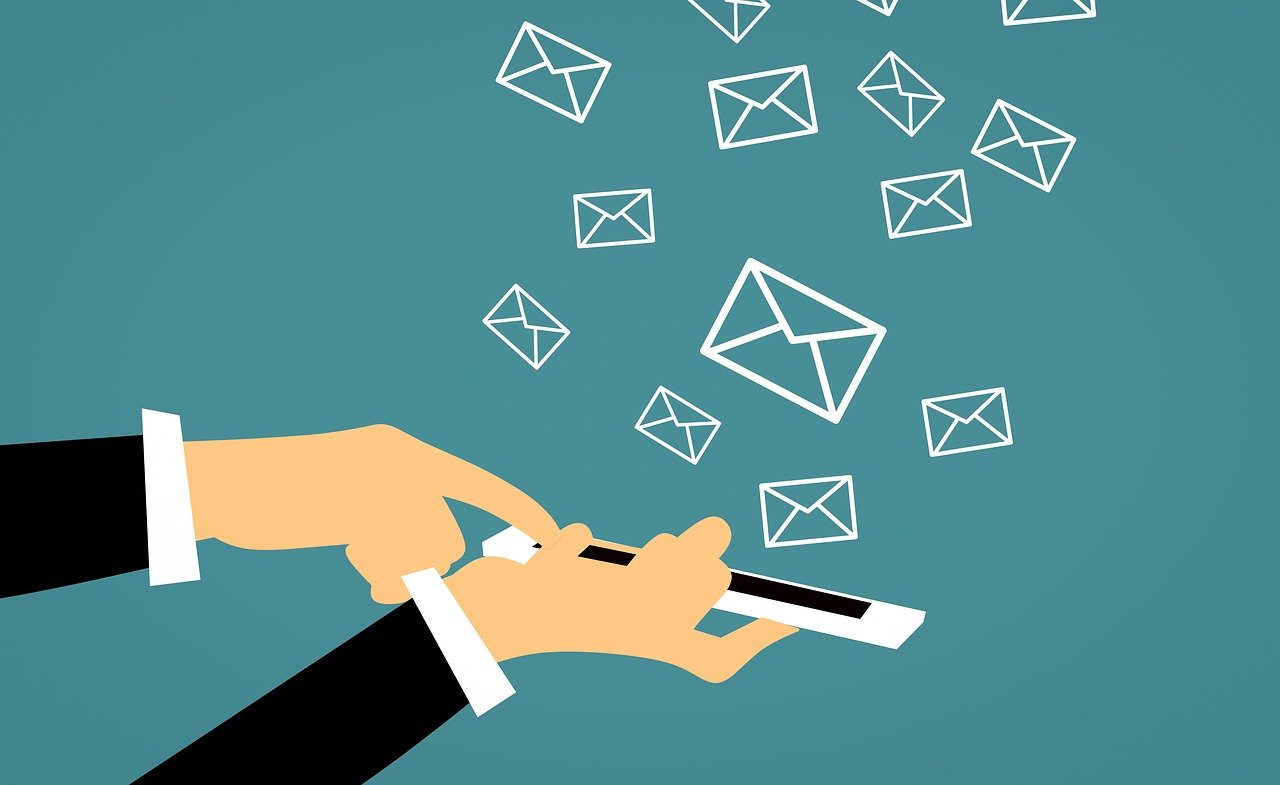
Navigating New Email Standards: Your 2024 Readiness Checklist
Navigating New Email Standards: Your 2024 Readiness Checklist
Navigating New Email Standards: Your 2024 Readiness Checklist
Navigating New Email Standards: Your 2024 Readiness Checklist
Dec 1, 2023
Dec 1, 2023
Explore
Explore
Blog
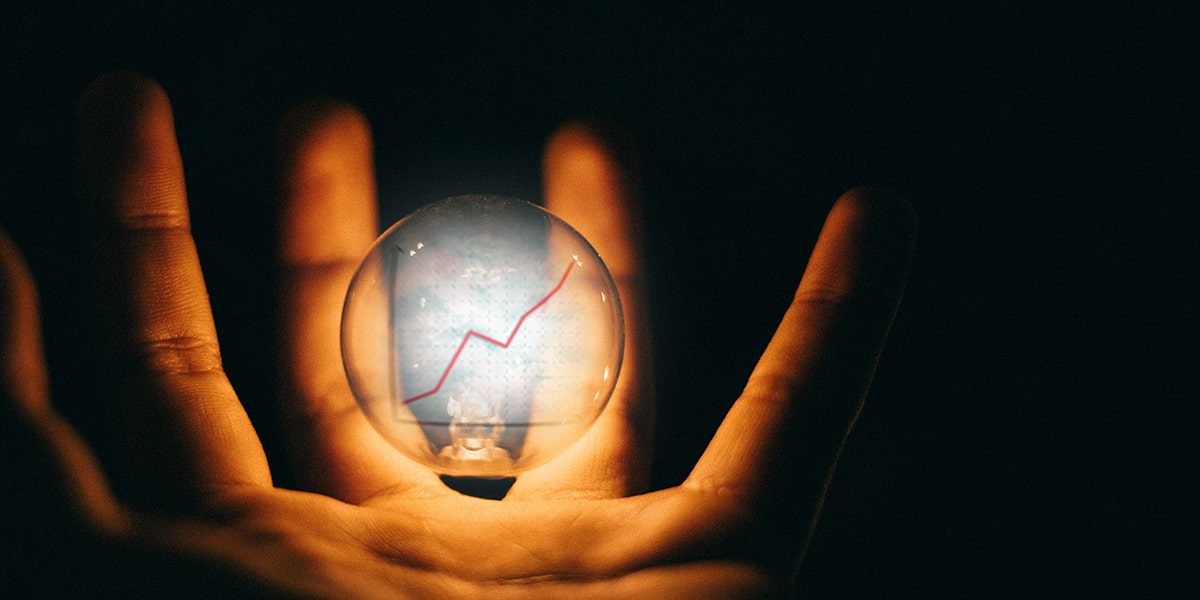
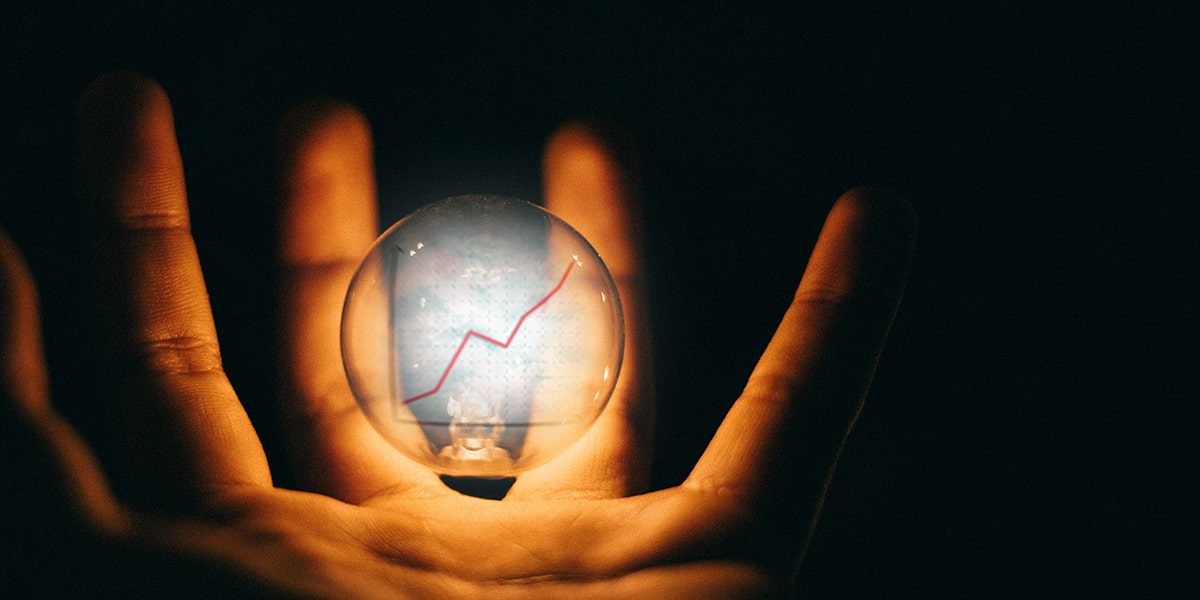
New tech makes account based marketing more achievable
New tech makes account based marketing more achievable
New tech makes account based marketing more achievable
New tech makes account based marketing more achievable
Feb 23, 2020
Feb 23, 2020
Explore
Explore
Blog
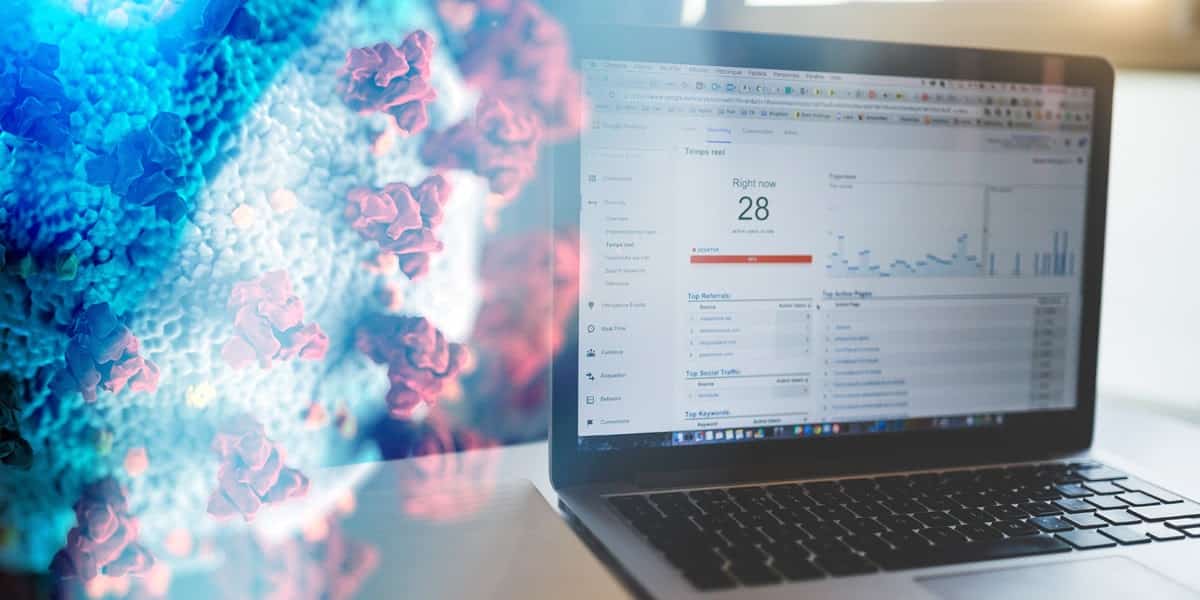
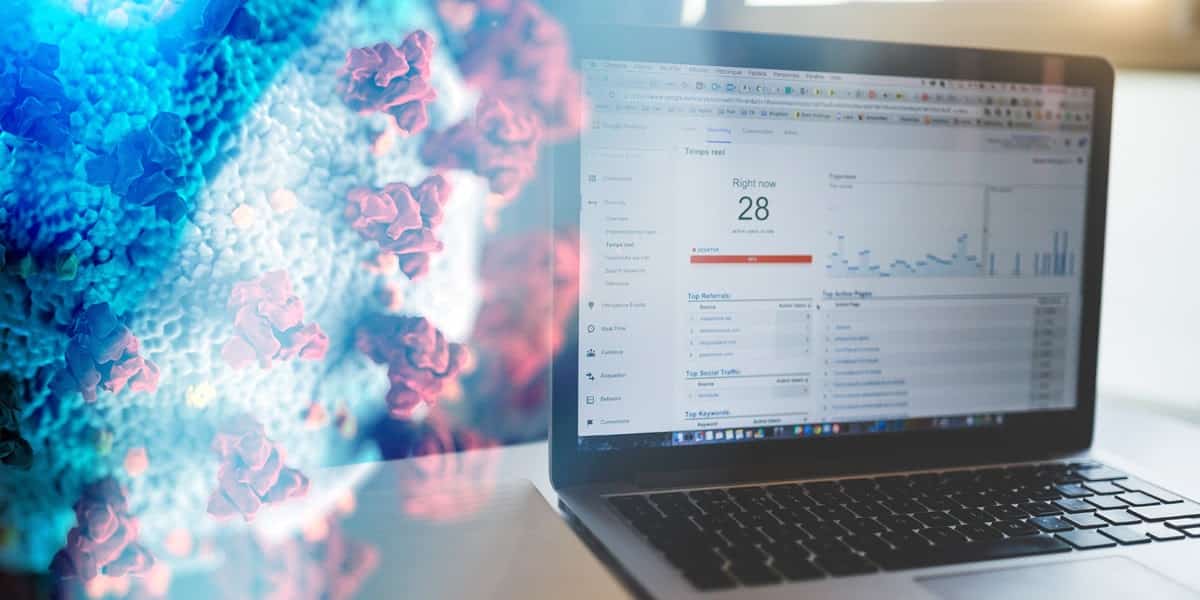
Optimizing Your Sales Pipeline During COVID-19
Optimizing Your Sales Pipeline During COVID-19
Optimizing Your Sales Pipeline During COVID-19
Optimizing Your Sales Pipeline During COVID-19
Mar 12, 2020
Mar 12, 2020
Explore
Explore
Blog
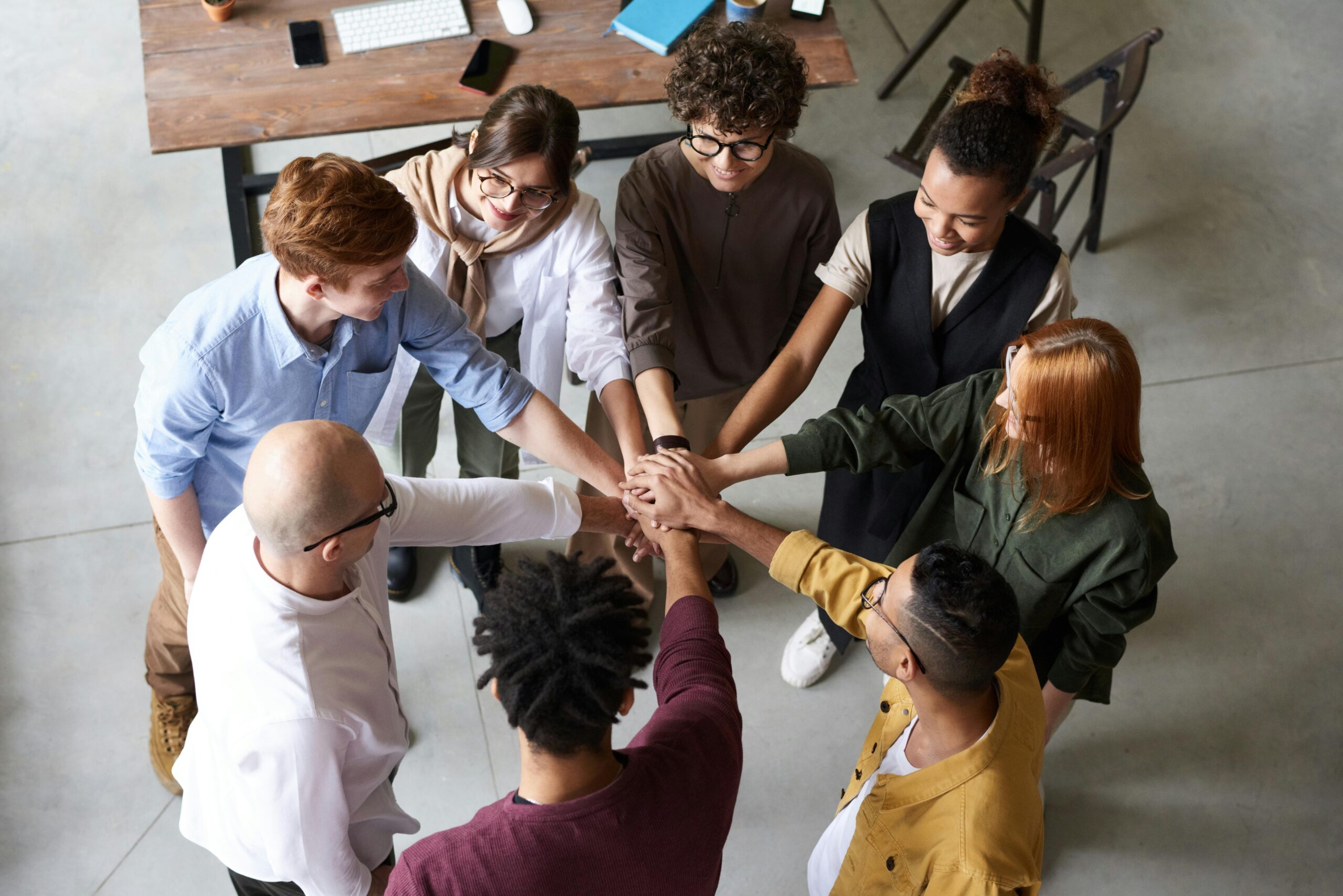
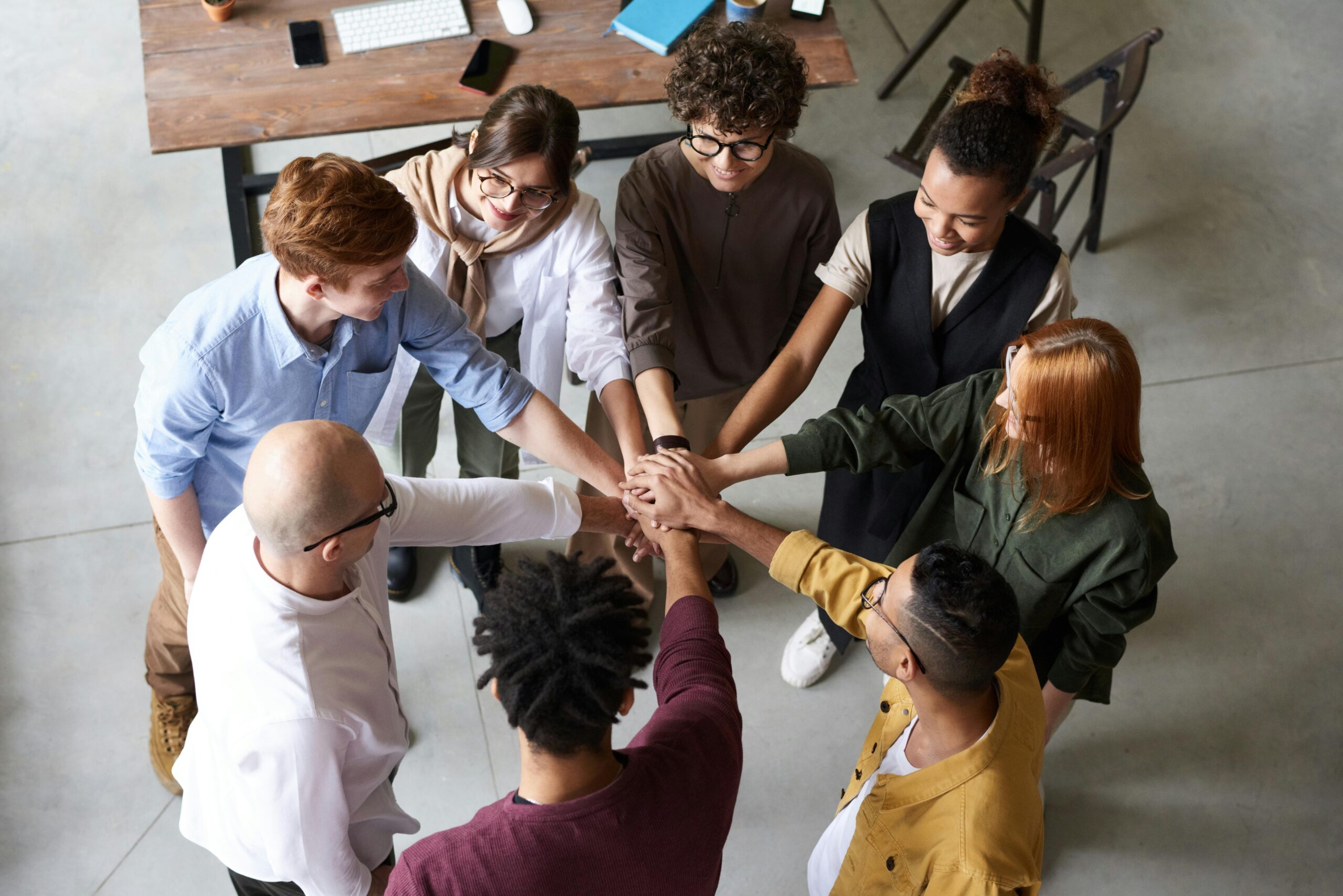
Our Approach to Combating Bias in AI Modeling
Our Approach to Combating Bias in AI Modeling
Our Approach to Combating Bias in AI Modeling
Our Approach to Combating Bias in AI Modeling
Mar 8, 2024
Mar 8, 2024
Explore
Explore
Blog
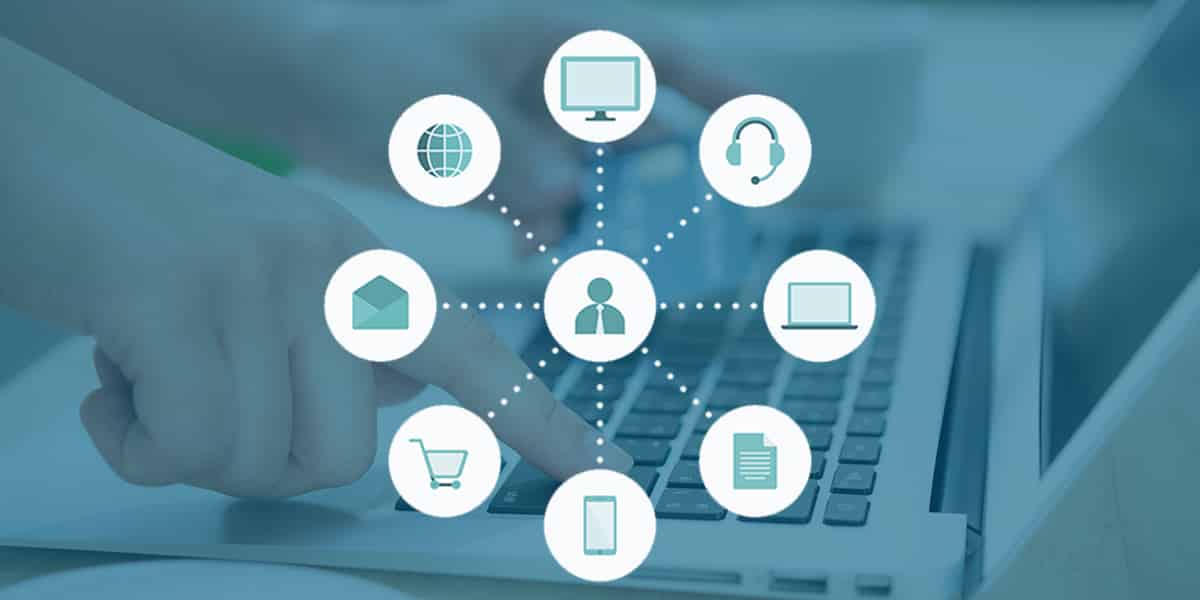
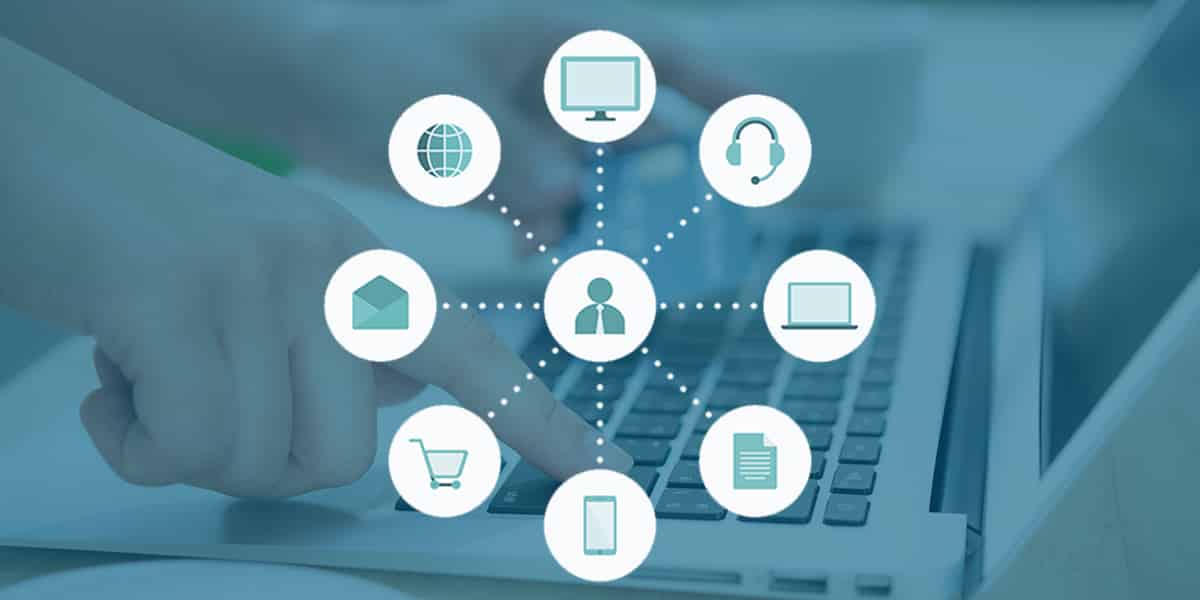
Personalized marketing is omnichannel marketing
Personalized marketing is omnichannel marketing
Personalized marketing is omnichannel marketing
Personalized marketing is omnichannel marketing
Mar 8, 2020
Mar 8, 2020
Explore
Explore
Blog
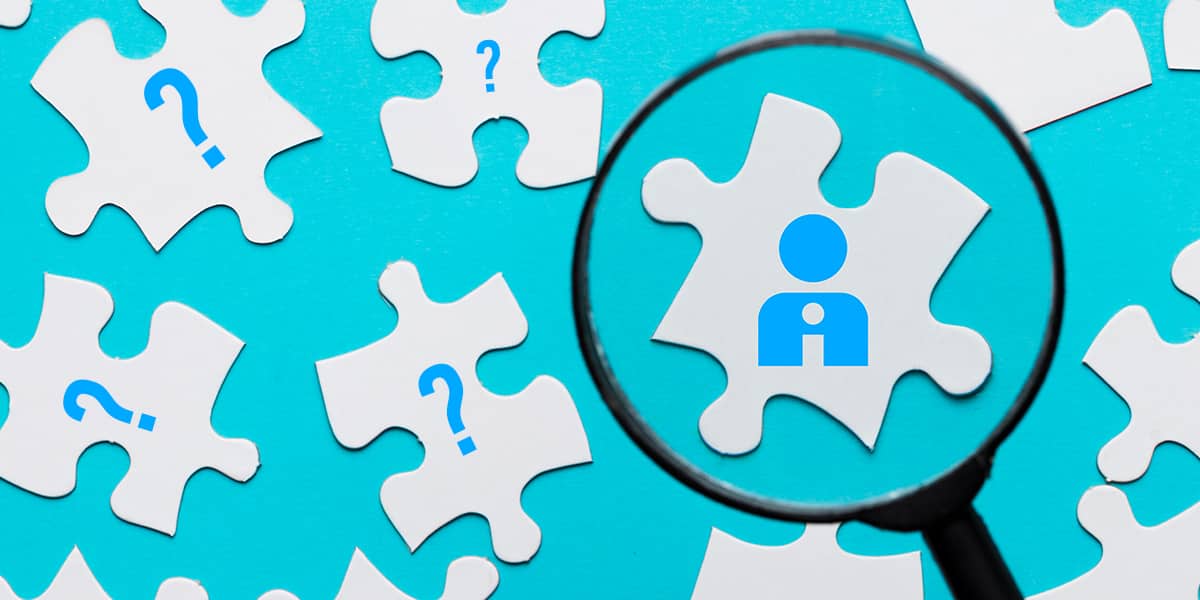
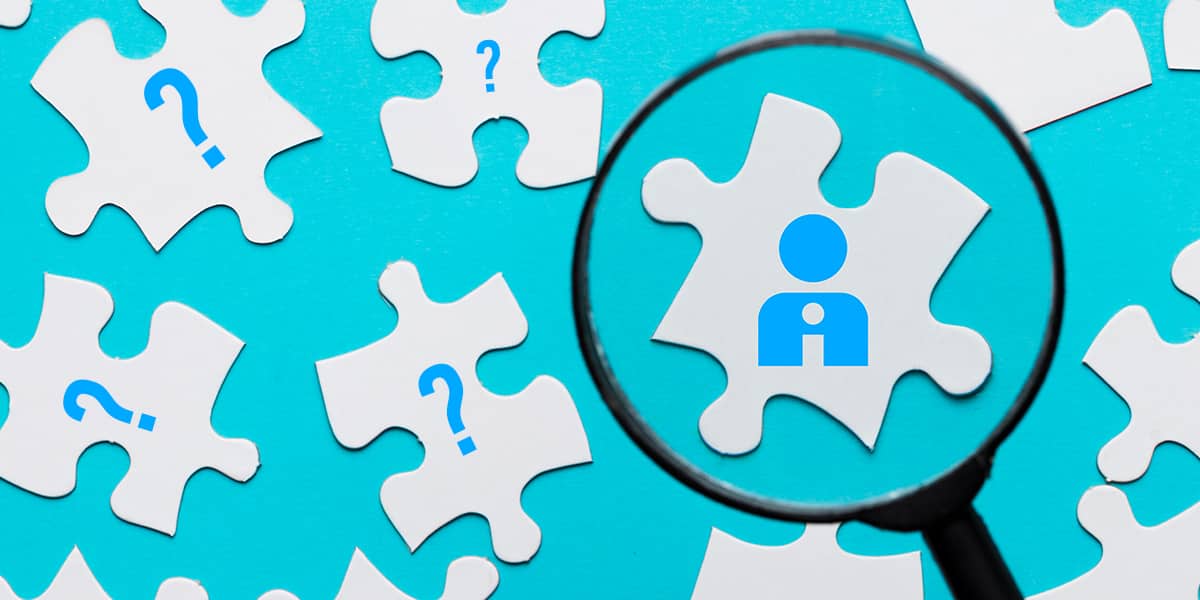
Personally Identifiable Information (PII) 101
Personally Identifiable Information (PII) 101
Personally Identifiable Information (PII) 101
Personally Identifiable Information (PII) 101
Mar 15, 2020
Mar 15, 2020
Explore
Explore
Blog
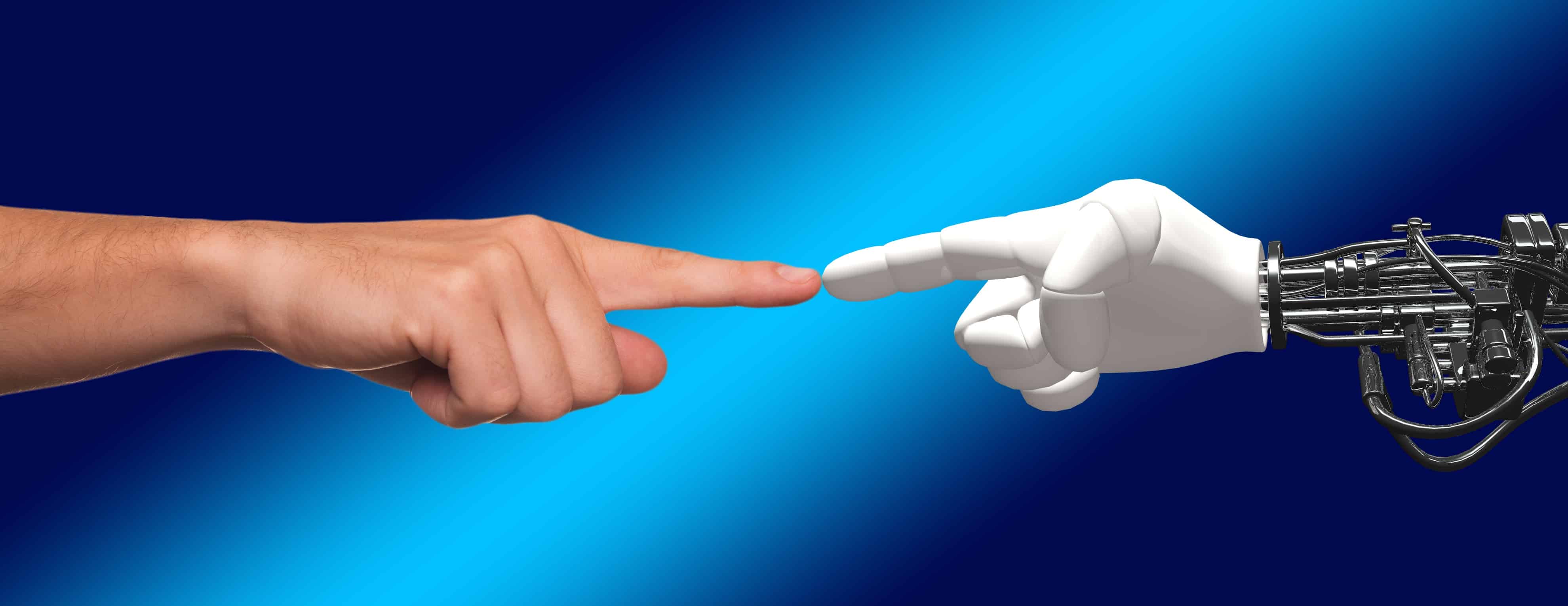
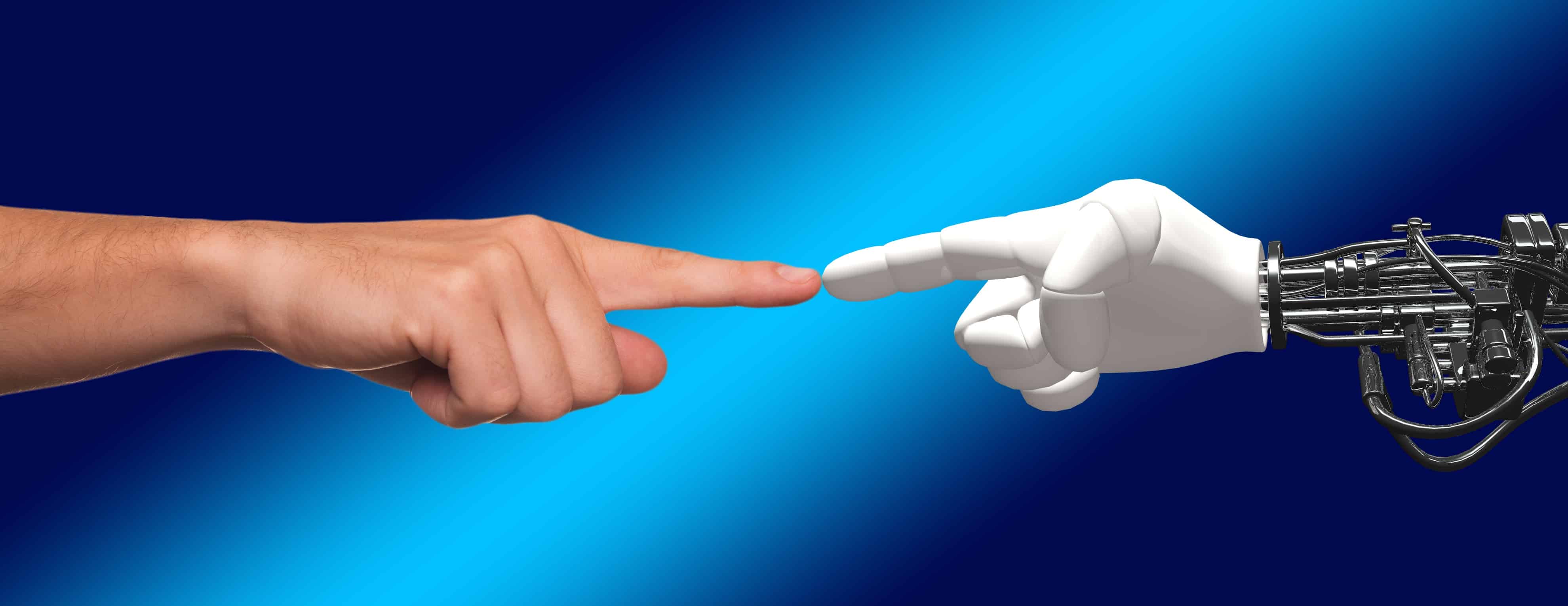
RE-POST: ARTIFICIAL INTELLIGENCE WILL ELIMINATE JOBS – AND CREATE THEM
RE-POST: ARTIFICIAL INTELLIGENCE WILL ELIMINATE JOBS – AND CREATE THEM
RE-POST: ARTIFICIAL INTELLIGENCE WILL ELIMINATE JOBS – AND CREATE THEM
RE-POST: ARTIFICIAL INTELLIGENCE WILL ELIMINATE JOBS – AND CREATE THEM
May 12, 2023
May 12, 2023
Explore
Explore
Blog
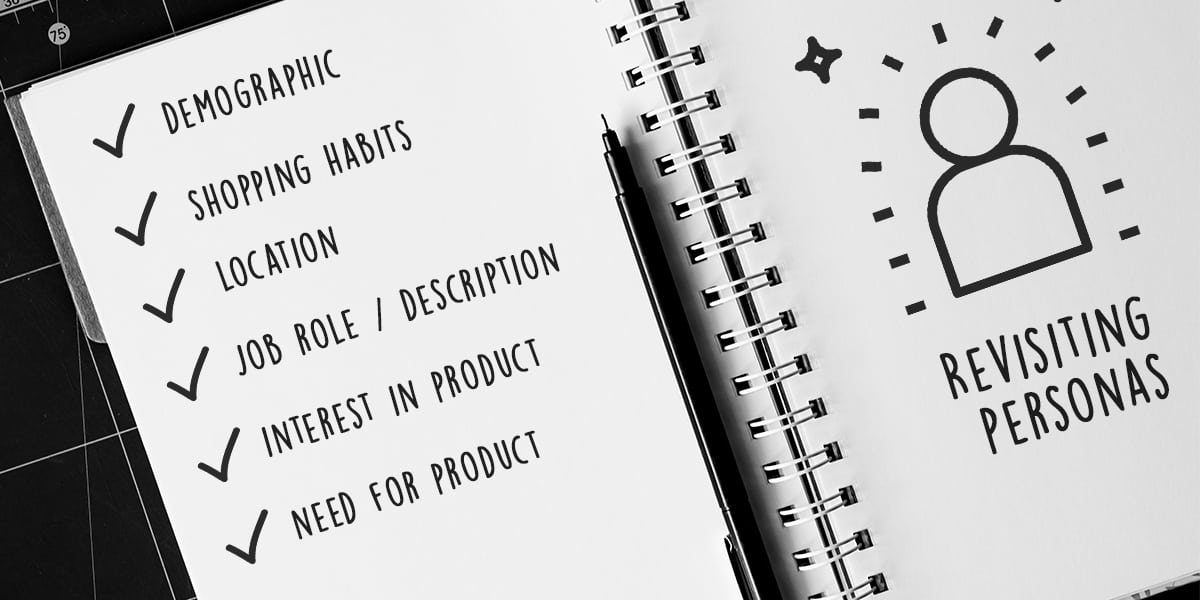
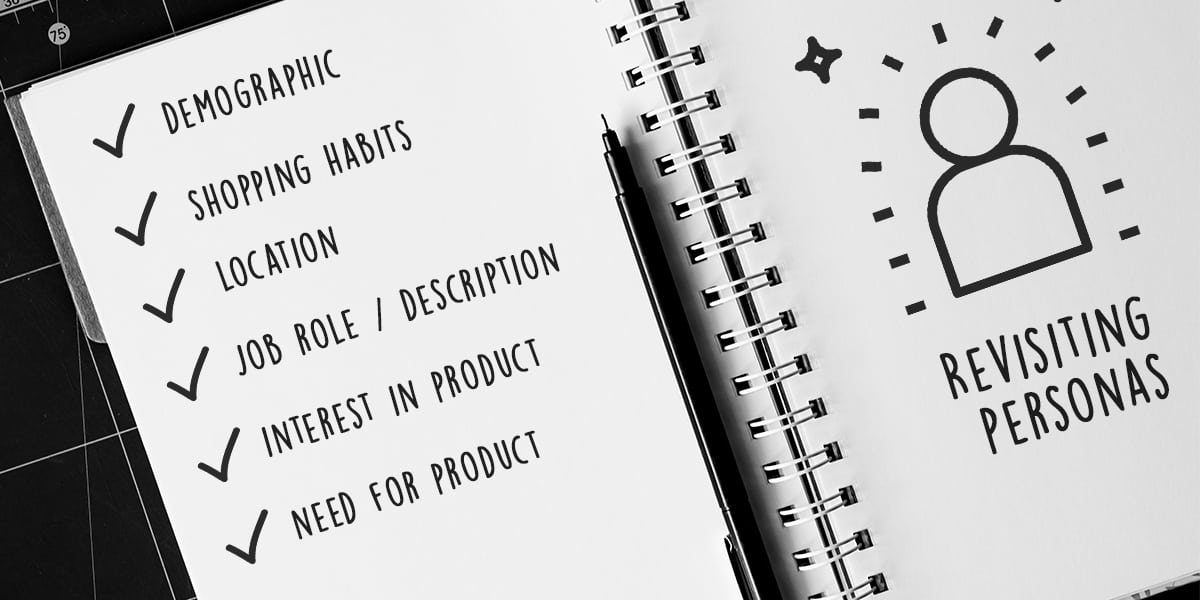
Revisiting Personas: Why they matter in the age of personalization
Revisiting Personas: Why they matter in the age of personalization
Revisiting Personas: Why they matter in the age of personalization
Revisiting Personas: Why they matter in the age of personalization
Mar 18, 2020
Mar 18, 2020
Explore
Explore
Blog
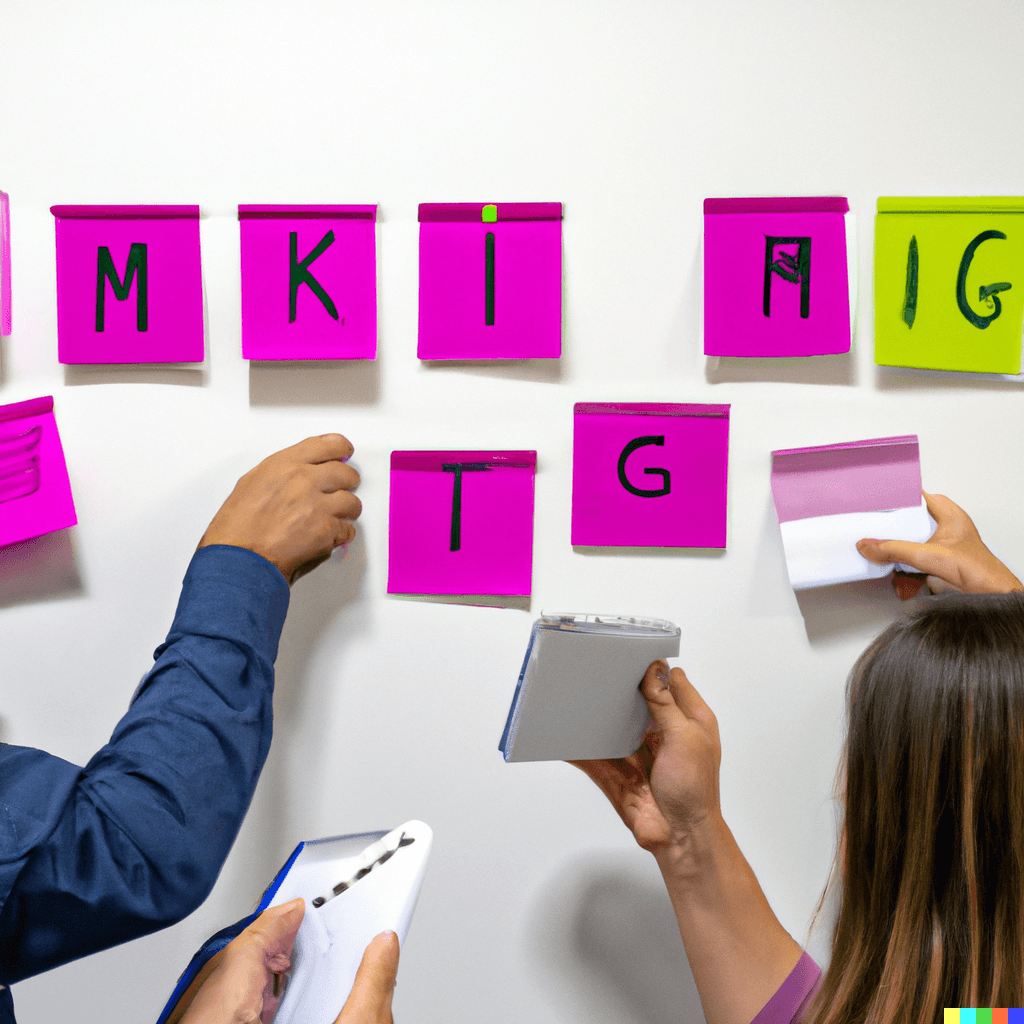
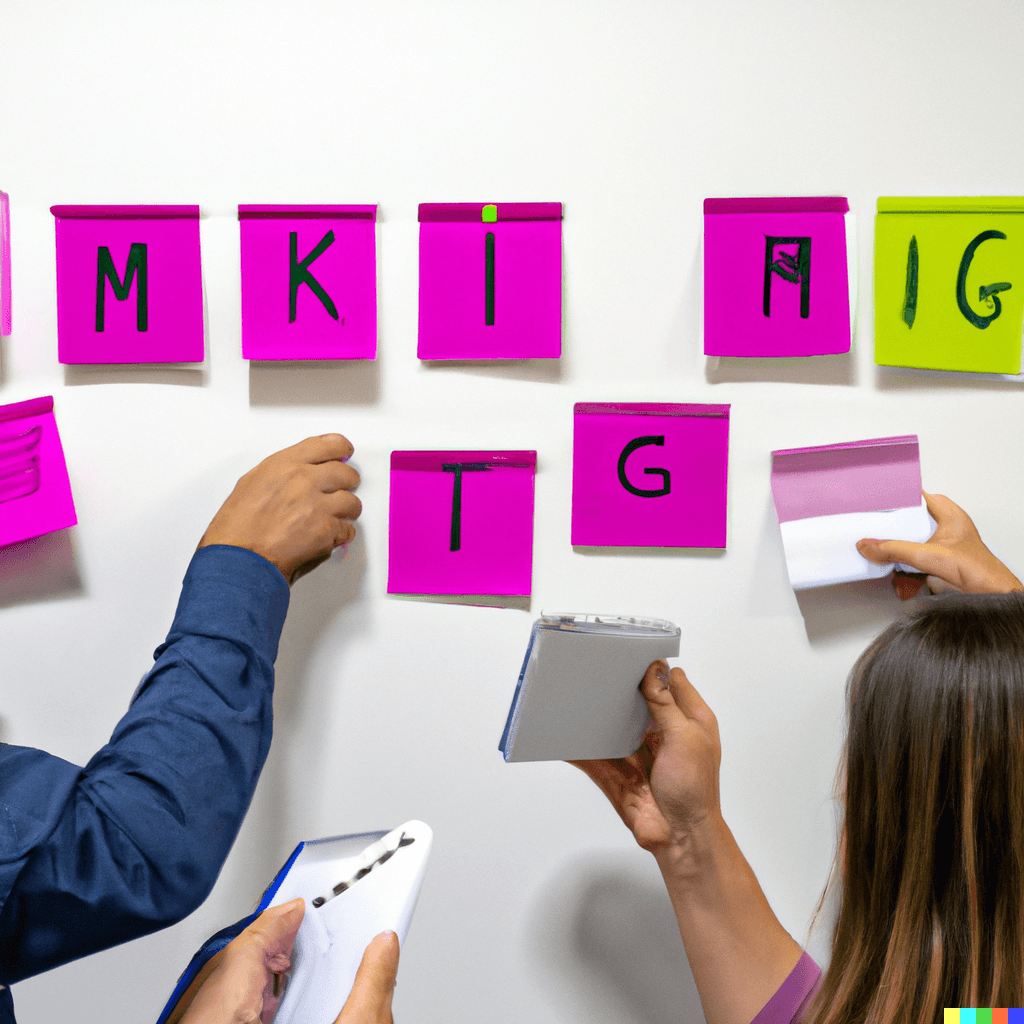
Tailoring Your Marketing Message: Beyond One-Size-Fits-All
Tailoring Your Marketing Message: Beyond One-Size-Fits-All
Tailoring Your Marketing Message: Beyond One-Size-Fits-All
Tailoring Your Marketing Message: Beyond One-Size-Fits-All
Jan 13, 2024
Jan 13, 2024
Explore
Explore
Blog
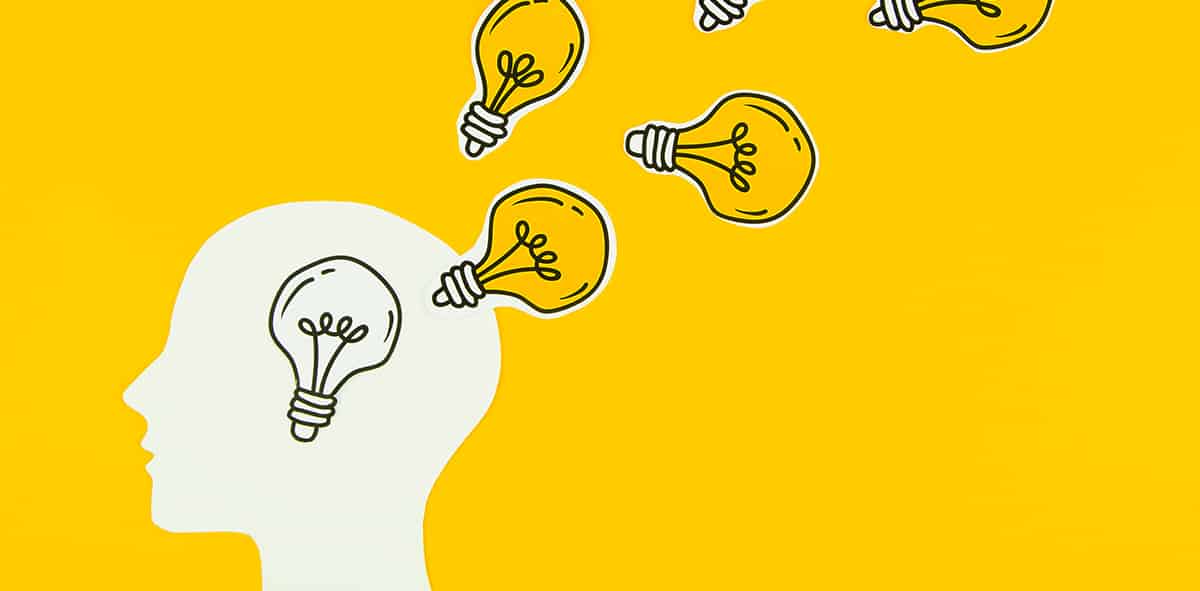
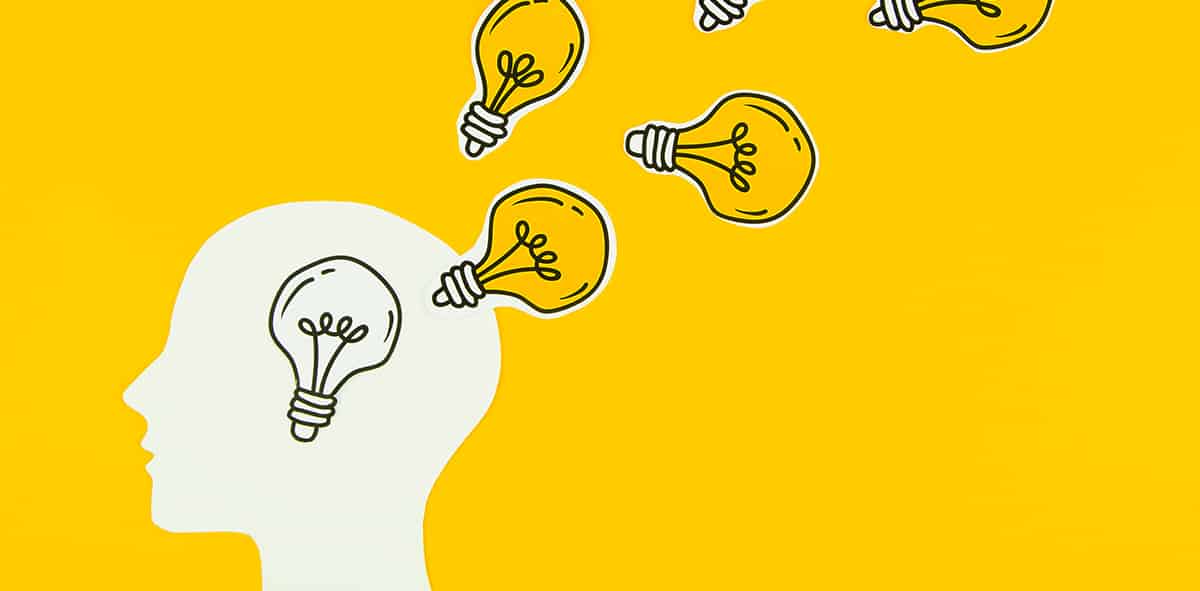
The Art of Using Persuasion Angles In Marketing & Sales
The Art of Using Persuasion Angles In Marketing & Sales
The Art of Using Persuasion Angles In Marketing & Sales
The Art of Using Persuasion Angles In Marketing & Sales
Jul 14, 2020
Jul 14, 2020
Explore
Explore
Blog
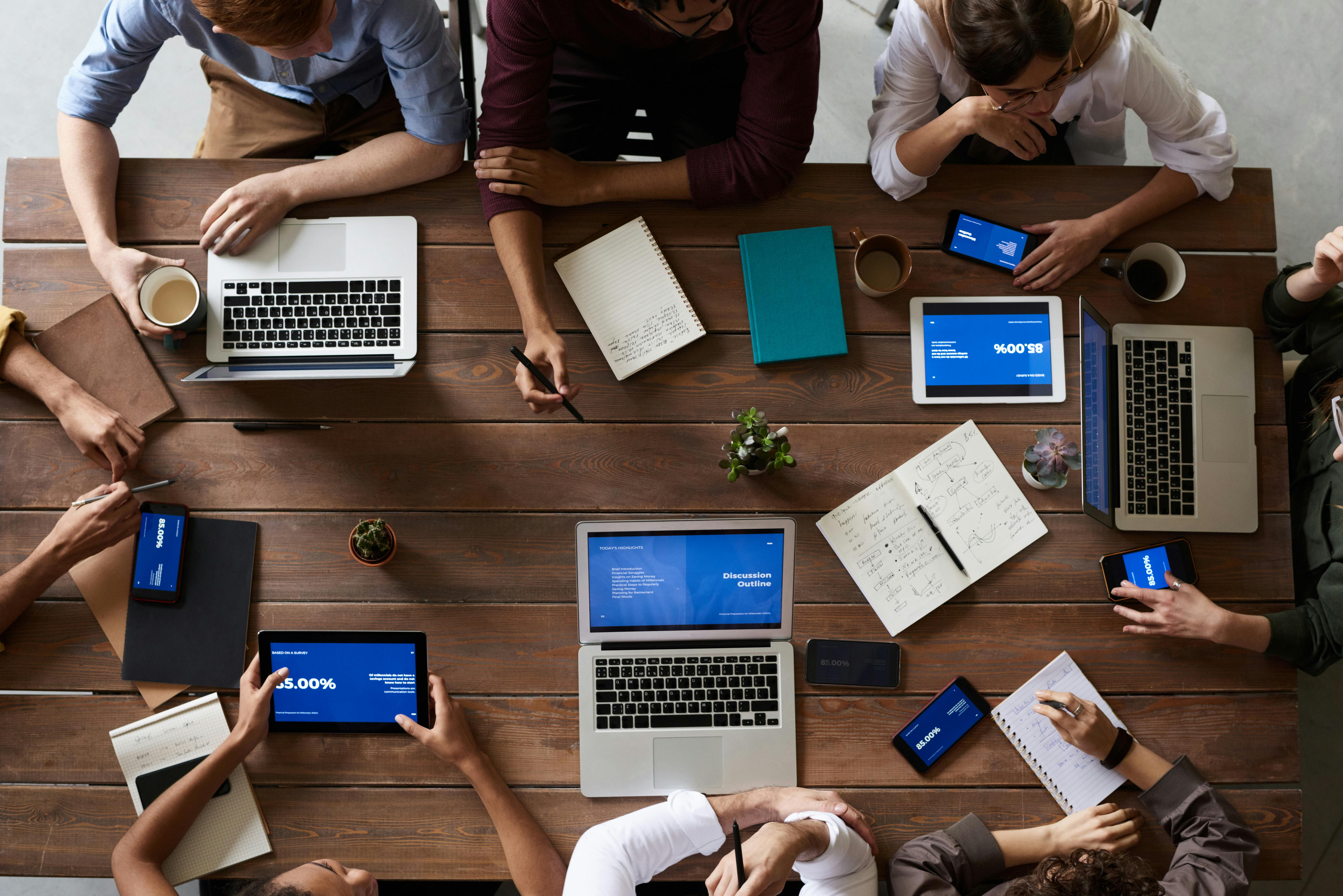
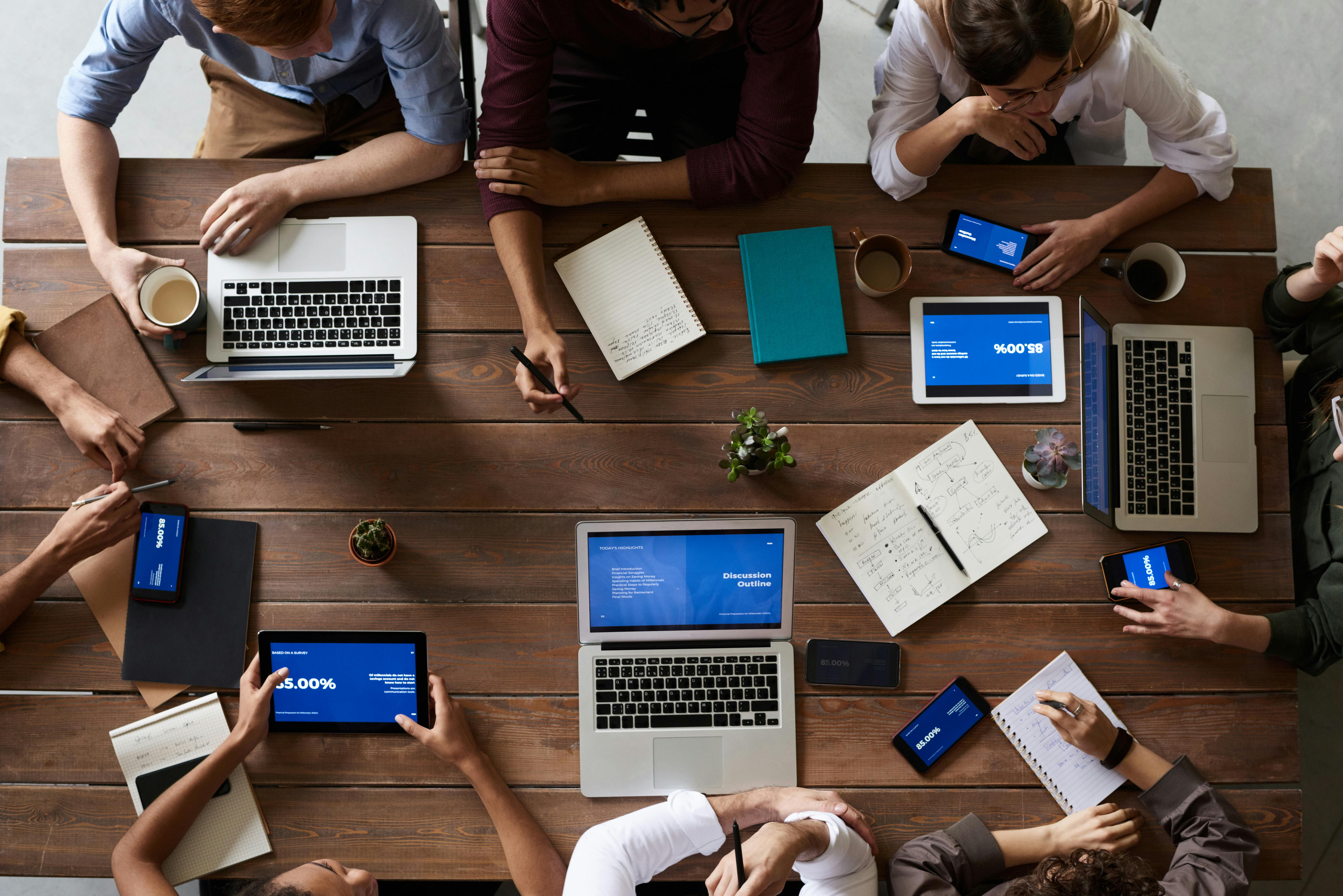
The Impact of AI in HR, Part 2: Try this approach for hiring or getting hired
The Impact of AI in HR, Part 2: Try this approach for hiring or getting hired
The Impact of AI in HR, Part 2: Try this approach for hiring or getting hired
The Impact of AI in HR, Part 2: Try this approach for hiring or getting hired
Mar 27, 2024
Mar 27, 2024
Explore
Explore
Blog
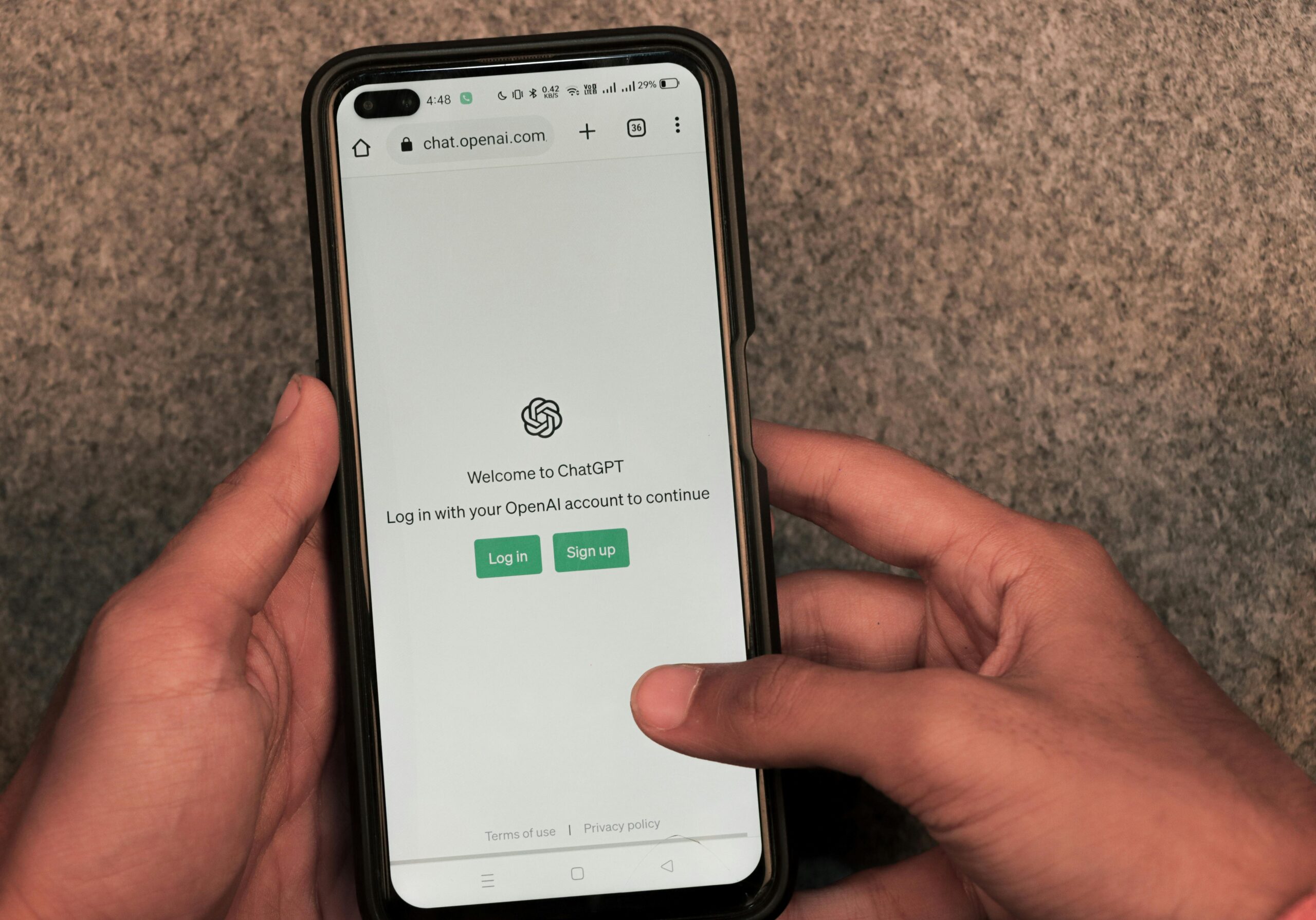
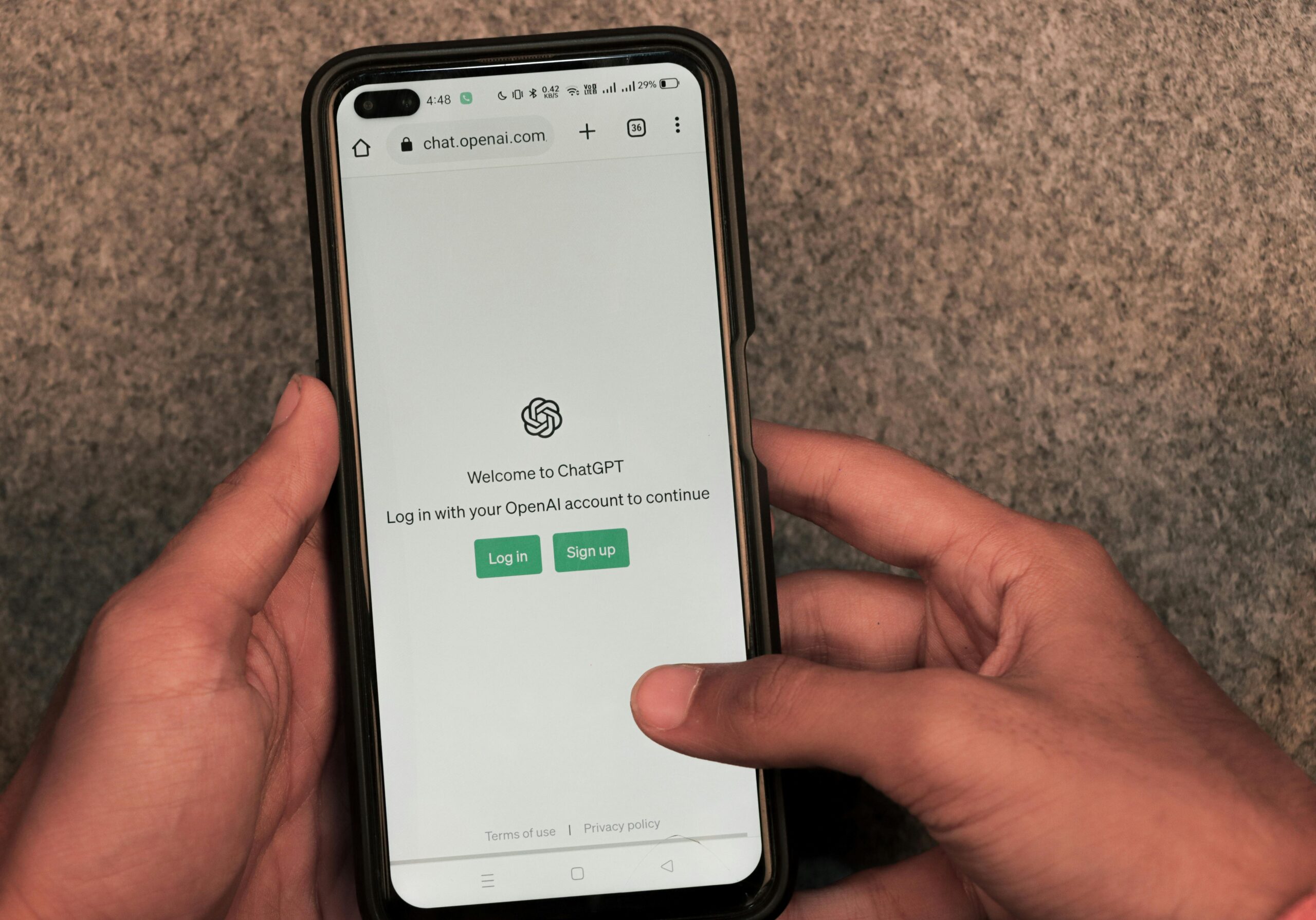
The Impact of AI on HR, Part 1: Everyone is using a GPT to get hired
The Impact of AI on HR, Part 1: Everyone is using a GPT to get hired
The Impact of AI on HR, Part 1: Everyone is using a GPT to get hired
The Impact of AI on HR, Part 1: Everyone is using a GPT to get hired
Mar 26, 2024
Mar 26, 2024
Explore
Explore
Blog
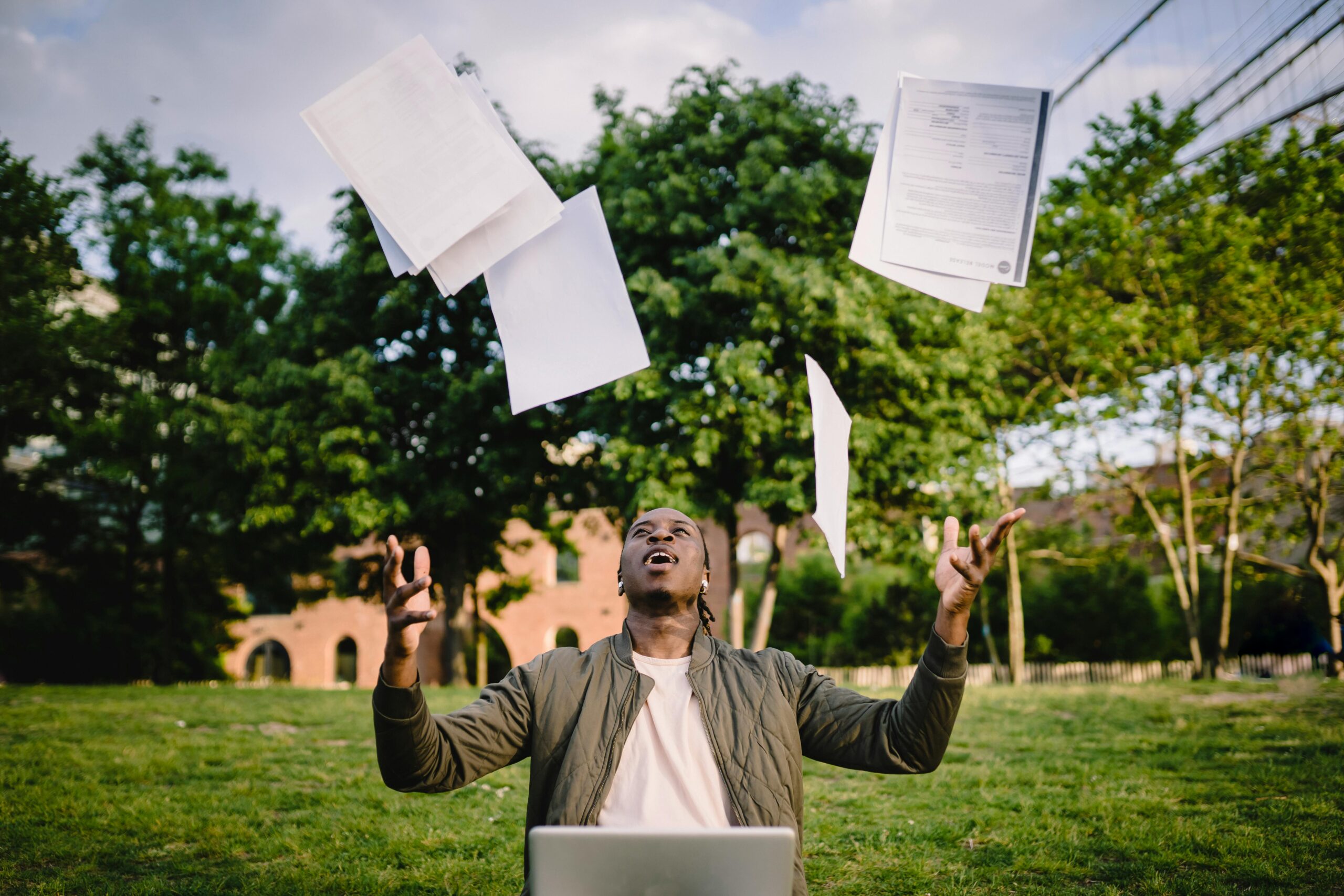
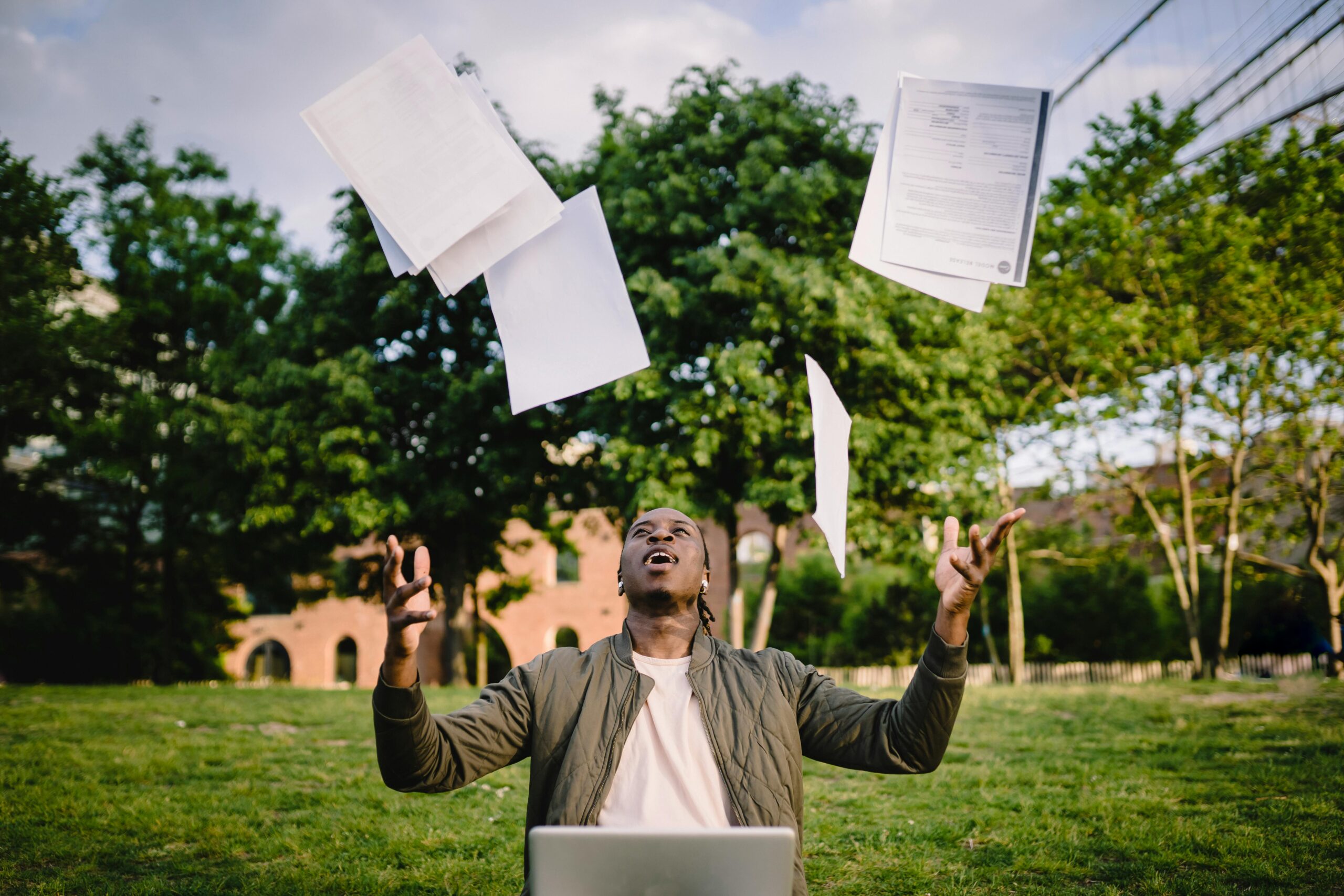
The Impact of AI on HR, part 3: Go beyond resumes to find the right people
The Impact of AI on HR, part 3: Go beyond resumes to find the right people
The Impact of AI on HR, part 3: Go beyond resumes to find the right people
The Impact of AI on HR, part 3: Go beyond resumes to find the right people
Mar 28, 2024
Mar 28, 2024
Explore
Explore
Blog
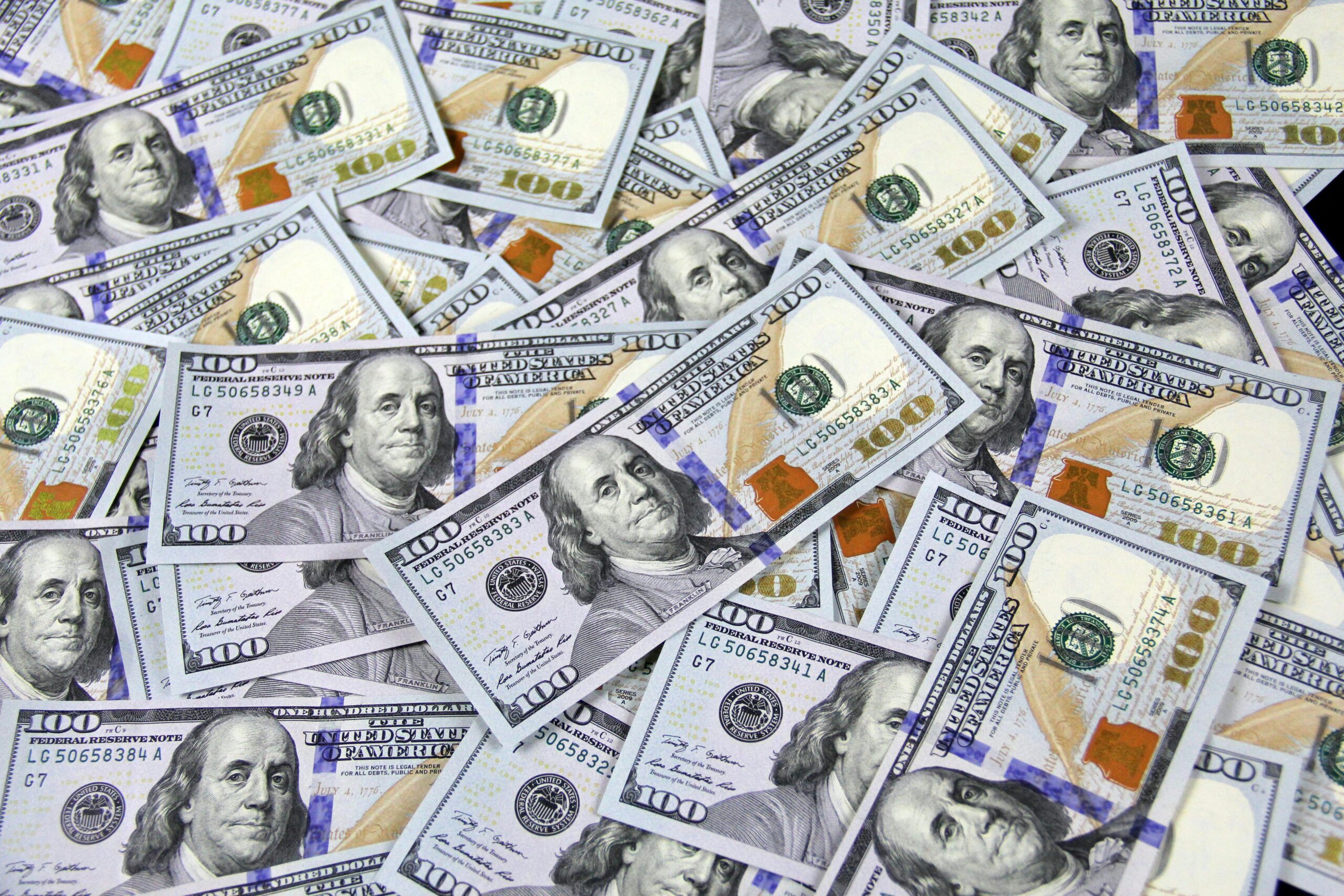
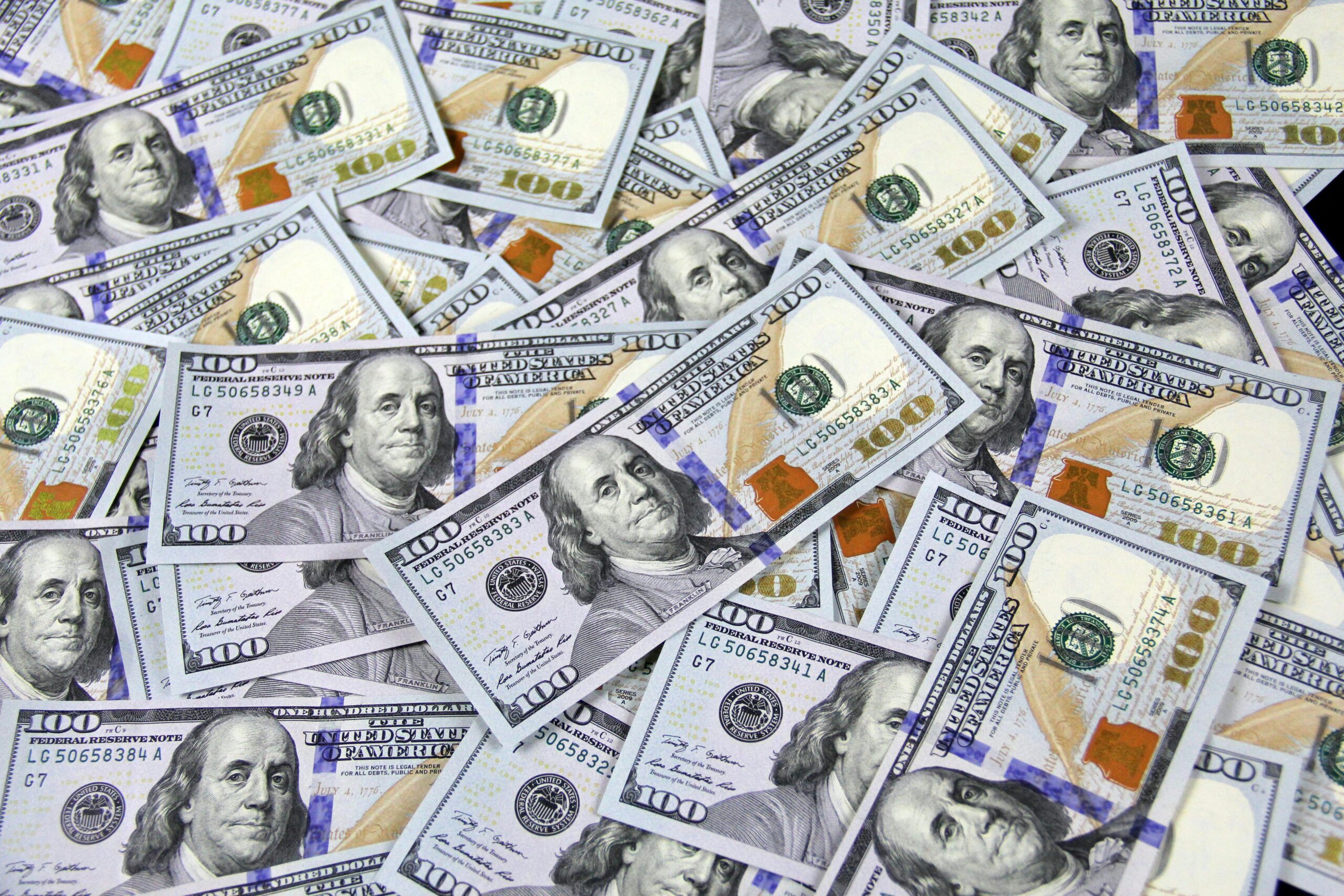
The Impact of AI on HR, Part 4: Understand how to reward employees so they stick around
The Impact of AI on HR, Part 4: Understand how to reward employees so they stick around
The Impact of AI on HR, Part 4: Understand how to reward employees so they stick around
The Impact of AI on HR, Part 4: Understand how to reward employees so they stick around
Mar 30, 2024
Mar 30, 2024
Explore
Explore
Blog
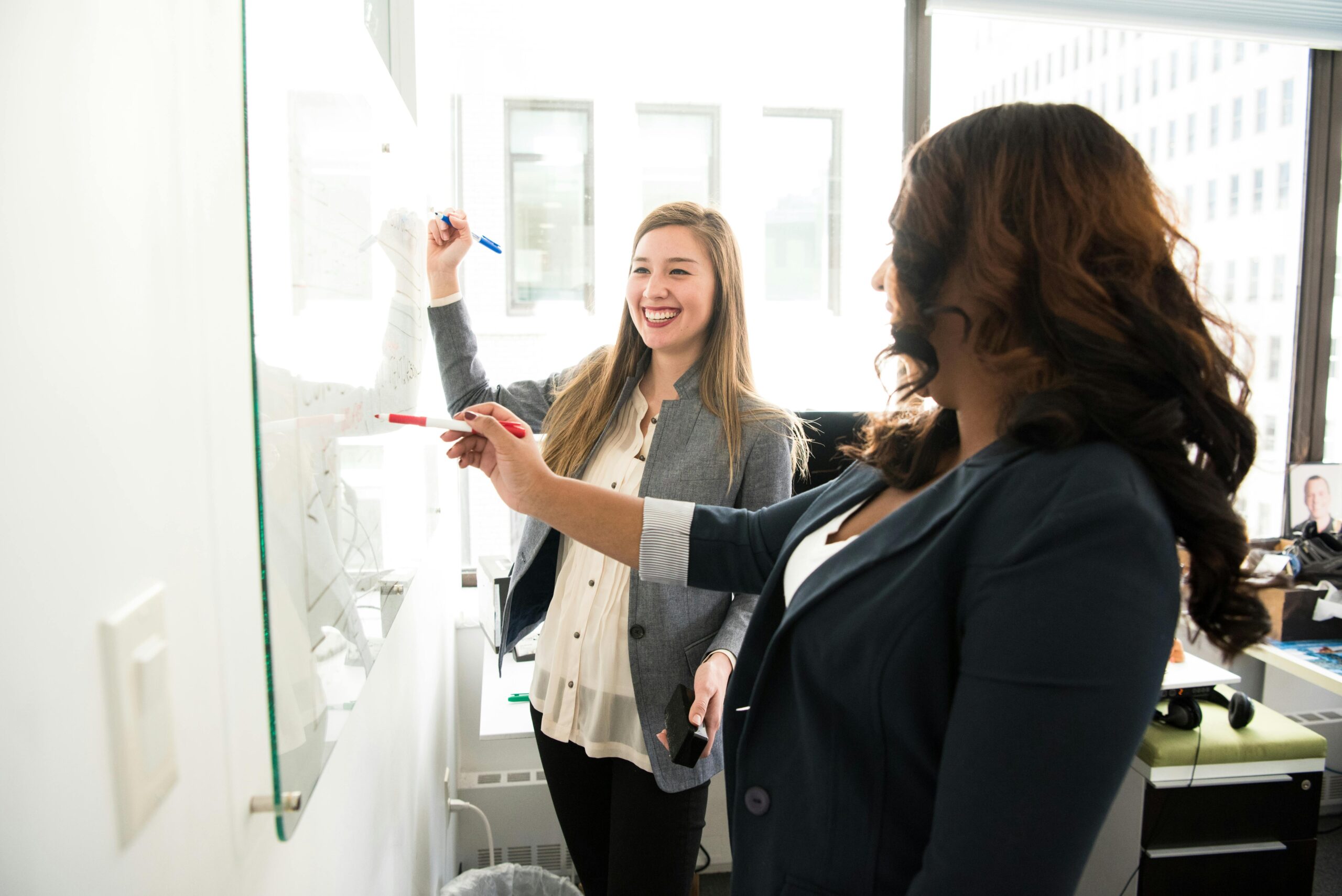
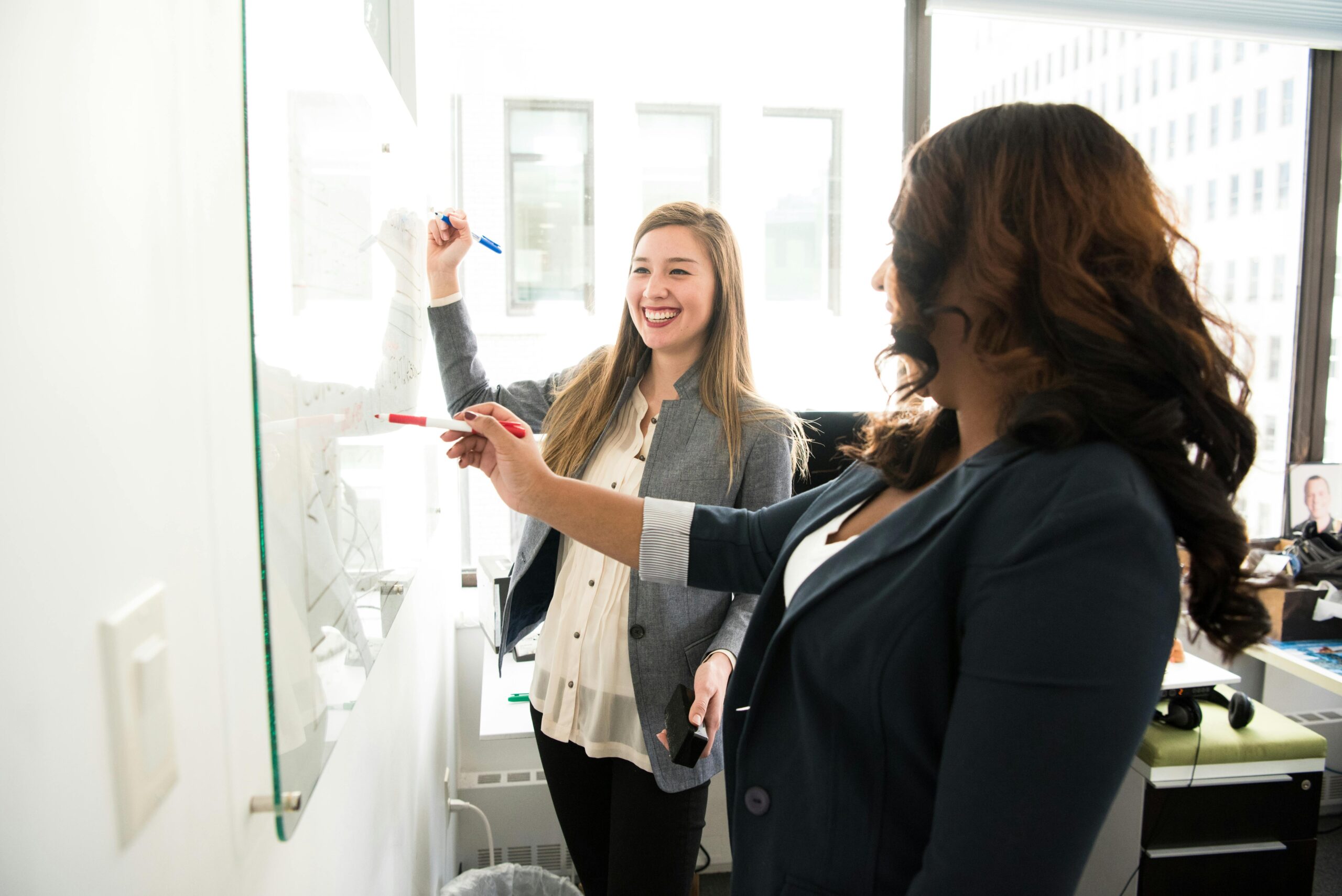
The Impact of AI on HR, Part 5: Why You Should Take a Chance on an Intern
The Impact of AI on HR, Part 5: Why You Should Take a Chance on an Intern
The Impact of AI on HR, Part 5: Why You Should Take a Chance on an Intern
The Impact of AI on HR, Part 5: Why You Should Take a Chance on an Intern
Mar 1, 2024
Mar 1, 2024
Explore
Explore
Blog
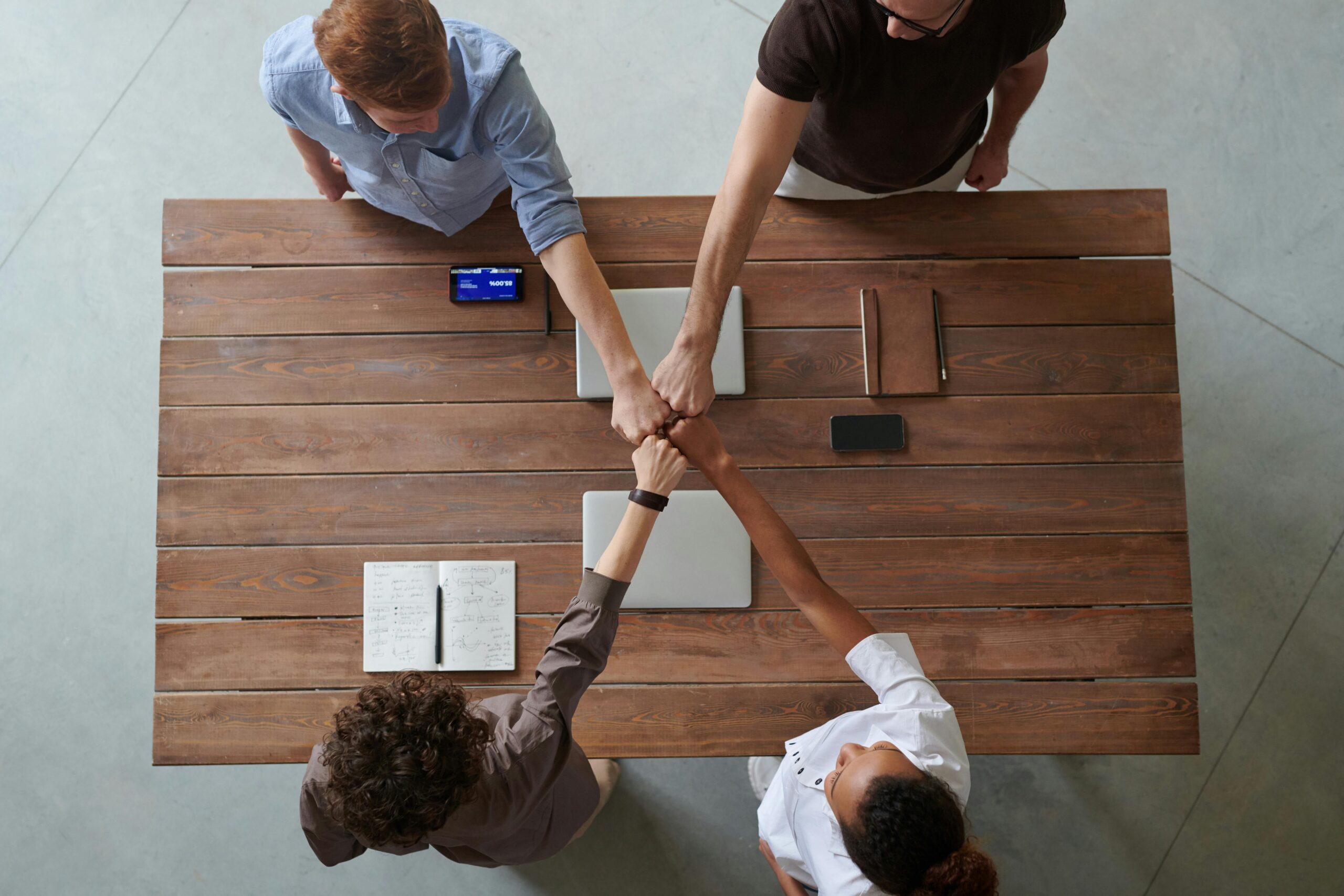
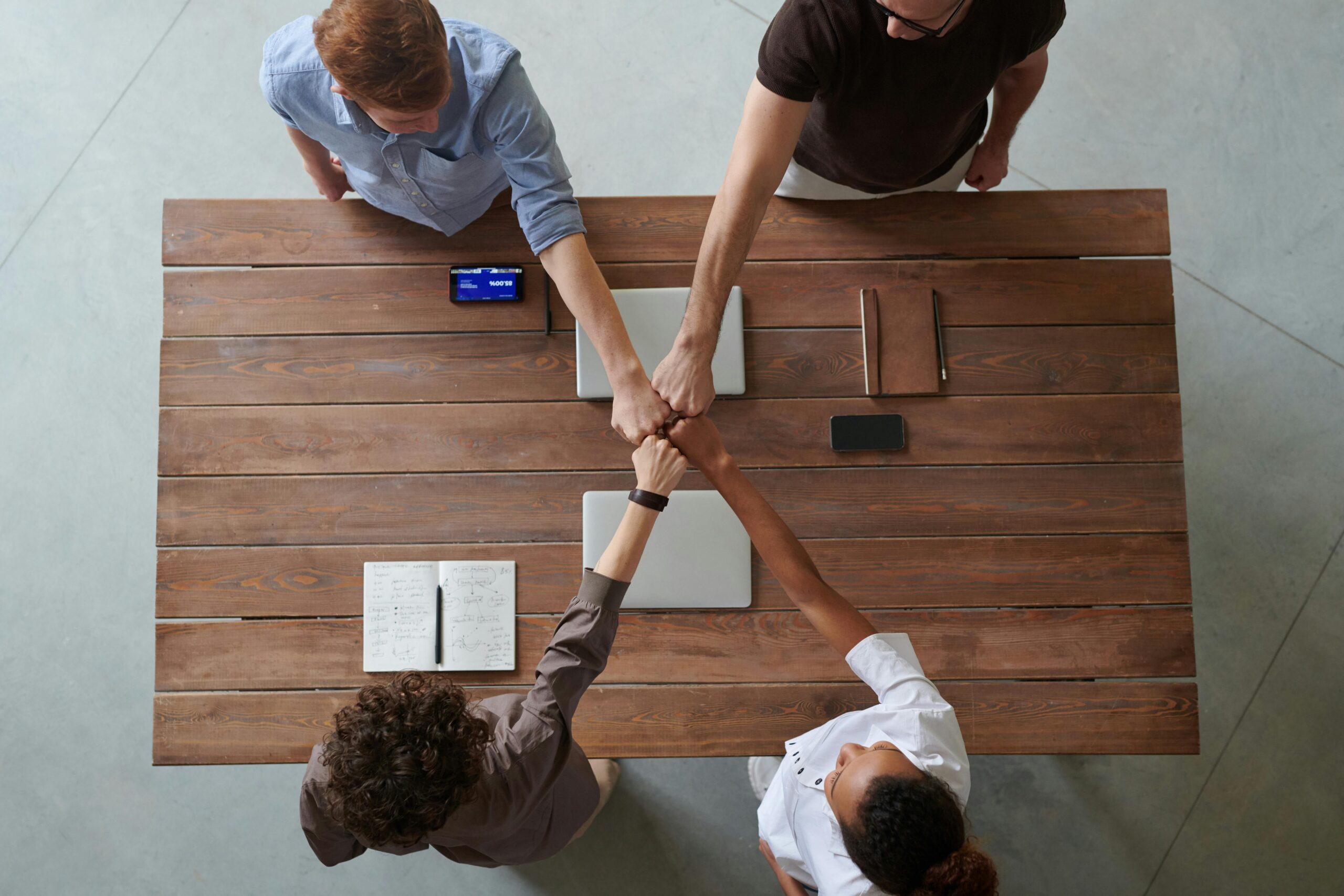
The Impact of AI on HR, Part 6: How to Address Resistance to AI in Your Organization
The Impact of AI on HR, Part 6: How to Address Resistance to AI in Your Organization
The Impact of AI on HR, Part 6: How to Address Resistance to AI in Your Organization
The Impact of AI on HR, Part 6: How to Address Resistance to AI in Your Organization
Apr 2, 2024
Apr 2, 2024
Explore
Explore
Blog
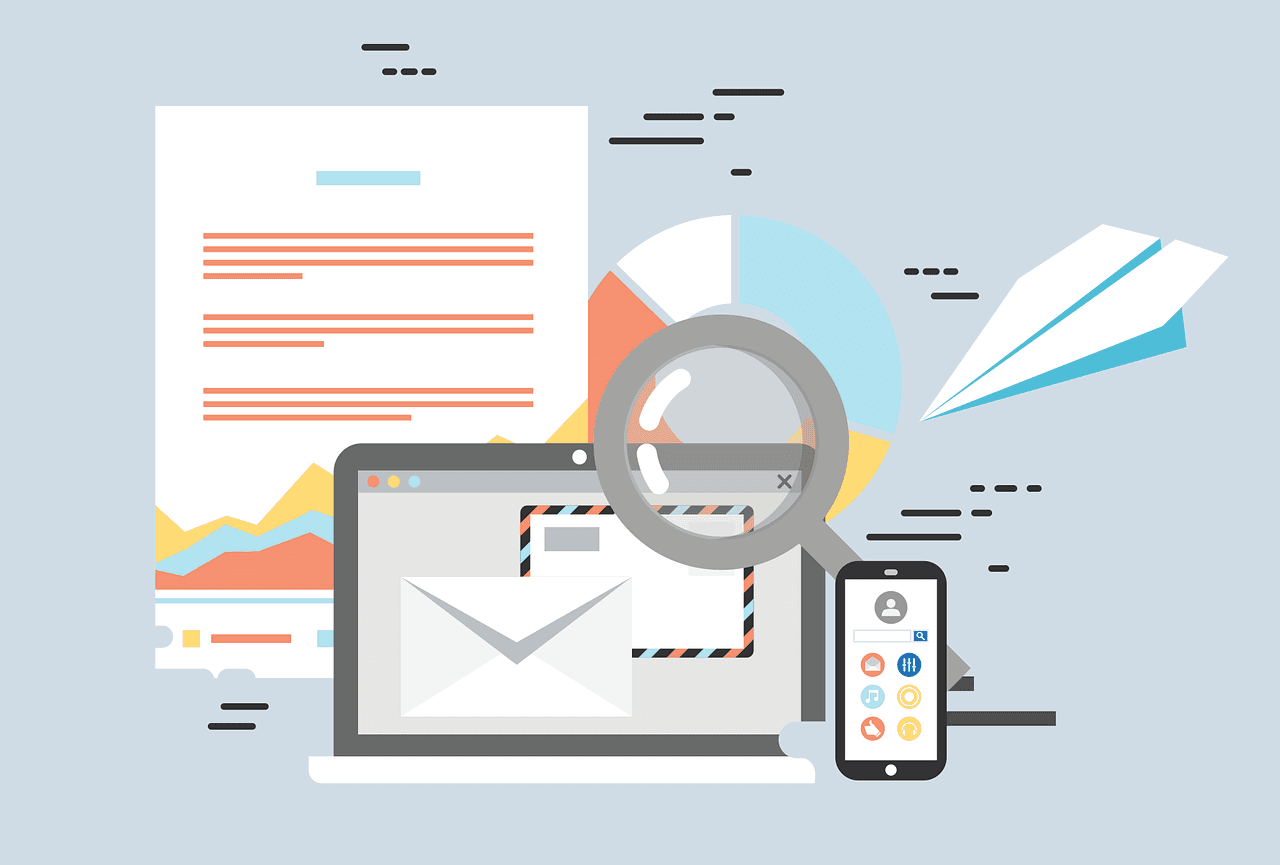
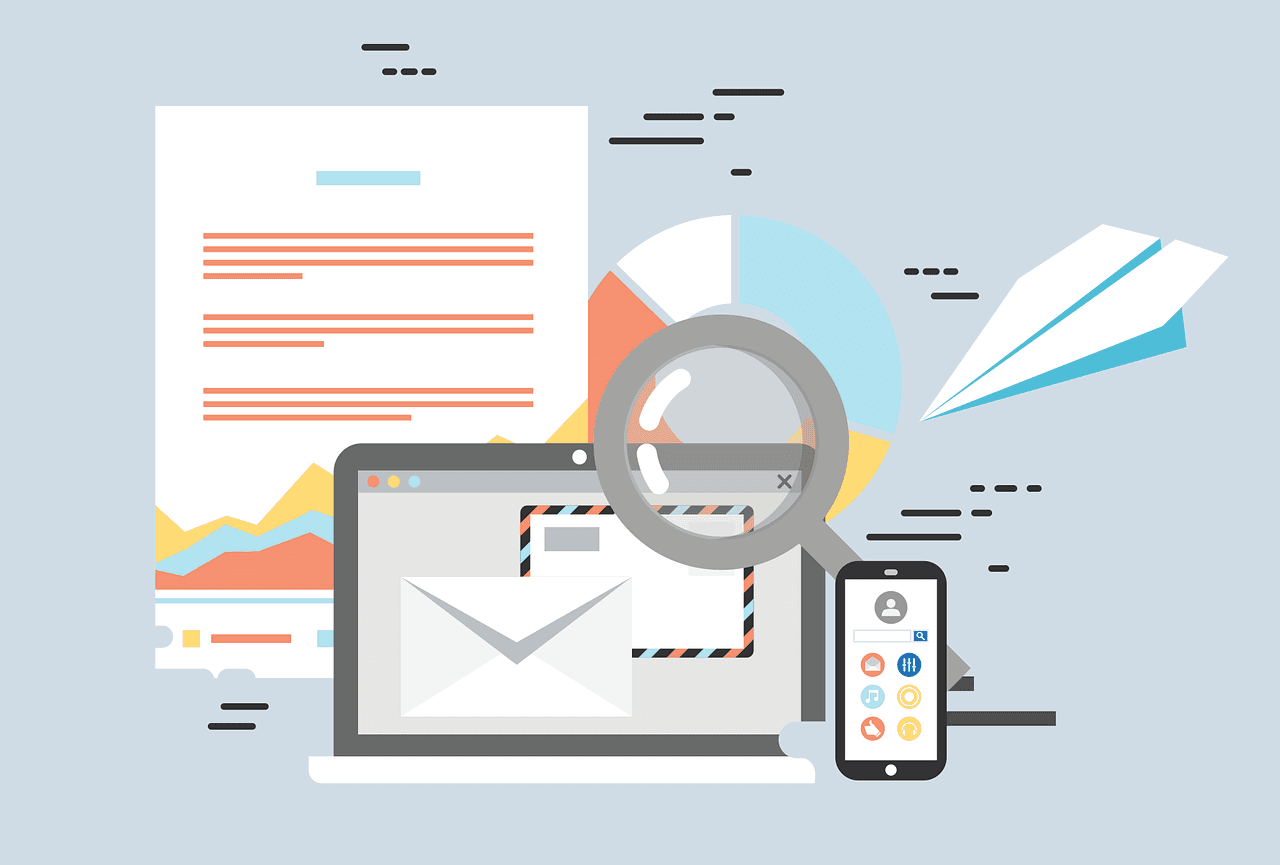
The Power of Emotional Benefits in Your Communication
The Power of Emotional Benefits in Your Communication
The Power of Emotional Benefits in Your Communication
The Power of Emotional Benefits in Your Communication
Jan 26, 2024
Jan 26, 2024
Explore
Explore
Blog
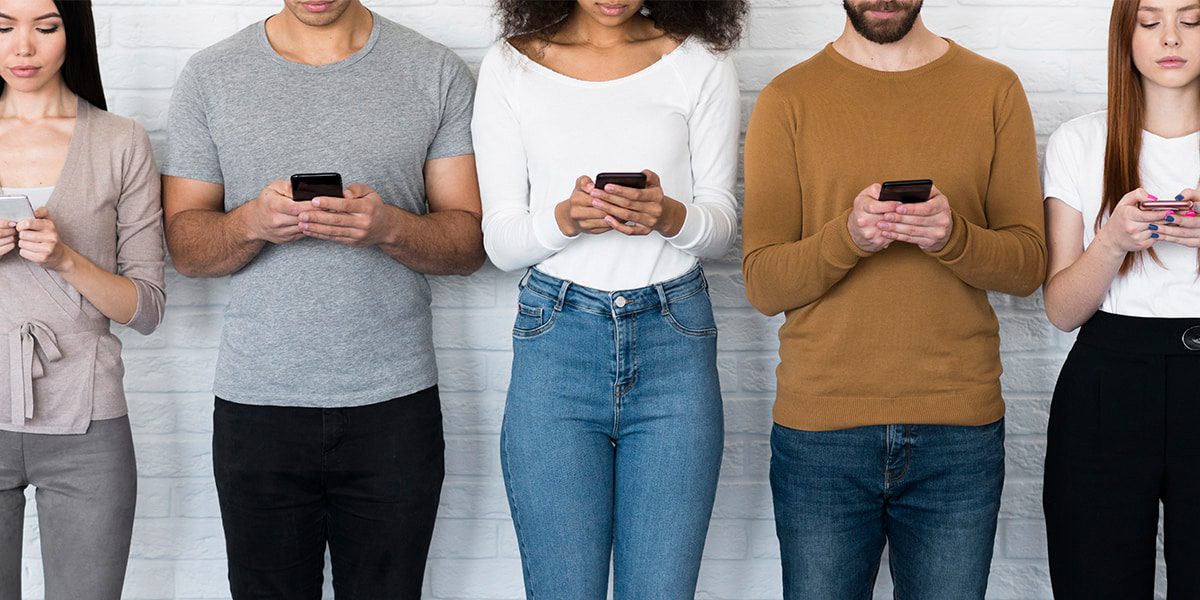
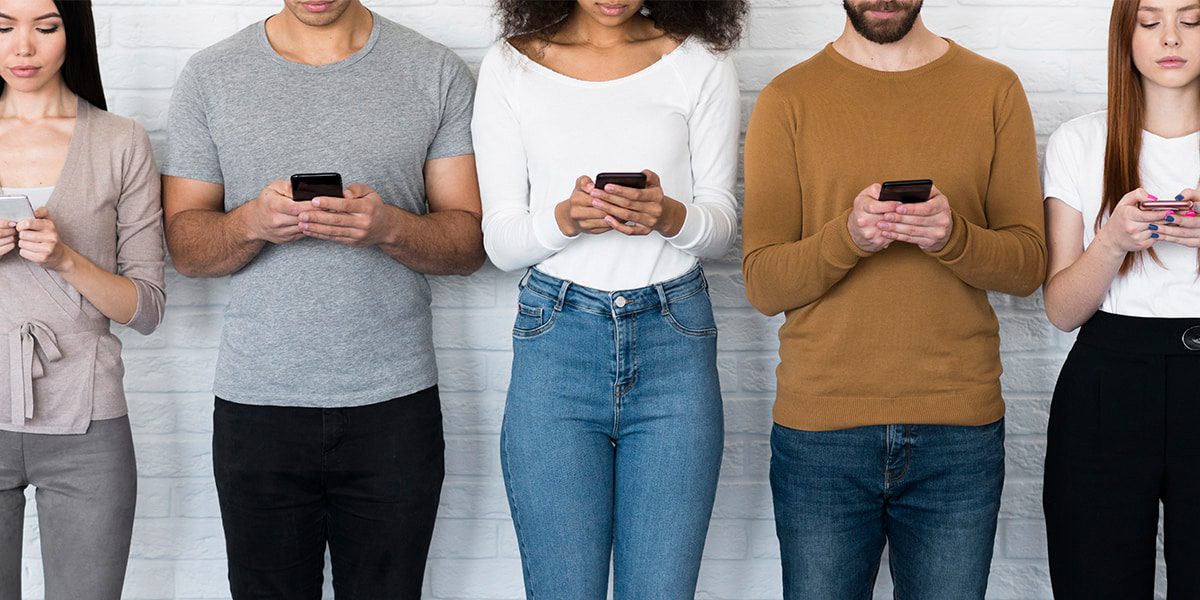
The rise of micro-moment: tiny moments, BIG impact
The rise of micro-moment: tiny moments, BIG impact
The rise of micro-moment: tiny moments, BIG impact
The rise of micro-moment: tiny moments, BIG impact
Mar 13, 2020
Mar 13, 2020
Explore
Explore
Blog
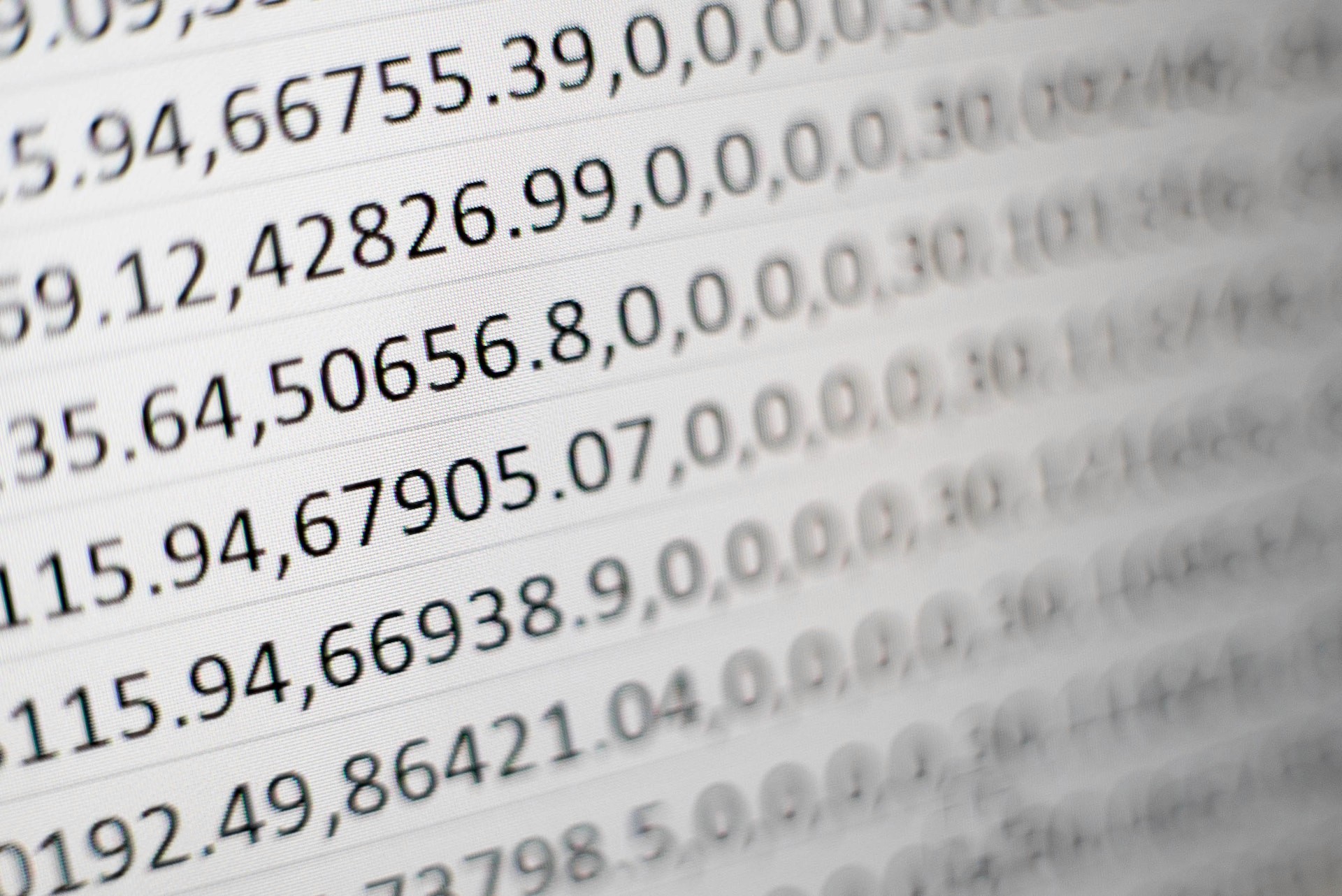
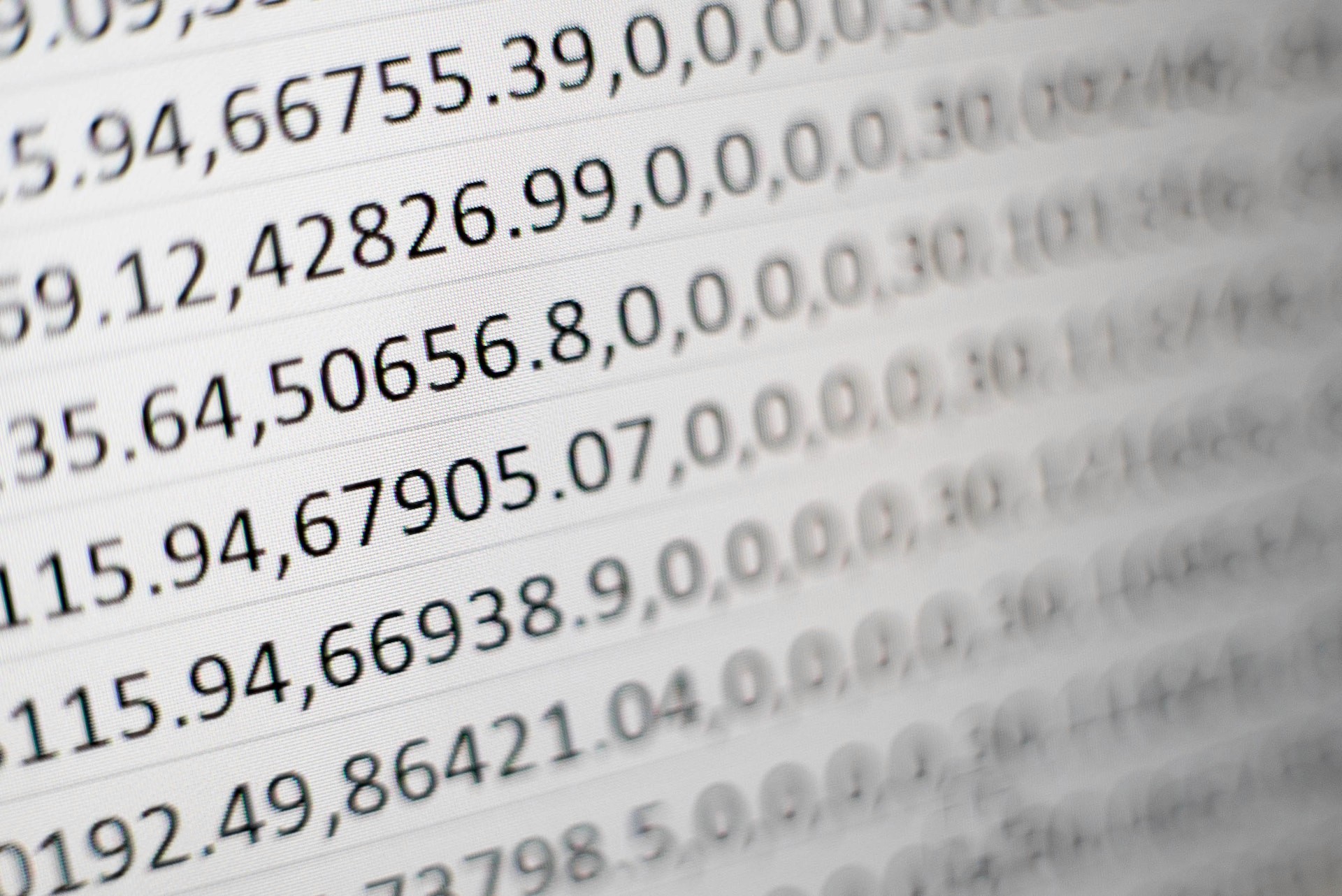
The Science of Match Scores: Predicting Customer Engagement
The Science of Match Scores: Predicting Customer Engagement
The Science of Match Scores: Predicting Customer Engagement
The Science of Match Scores: Predicting Customer Engagement
Apr 18, 2023
Apr 18, 2023
Explore
Explore
Blog
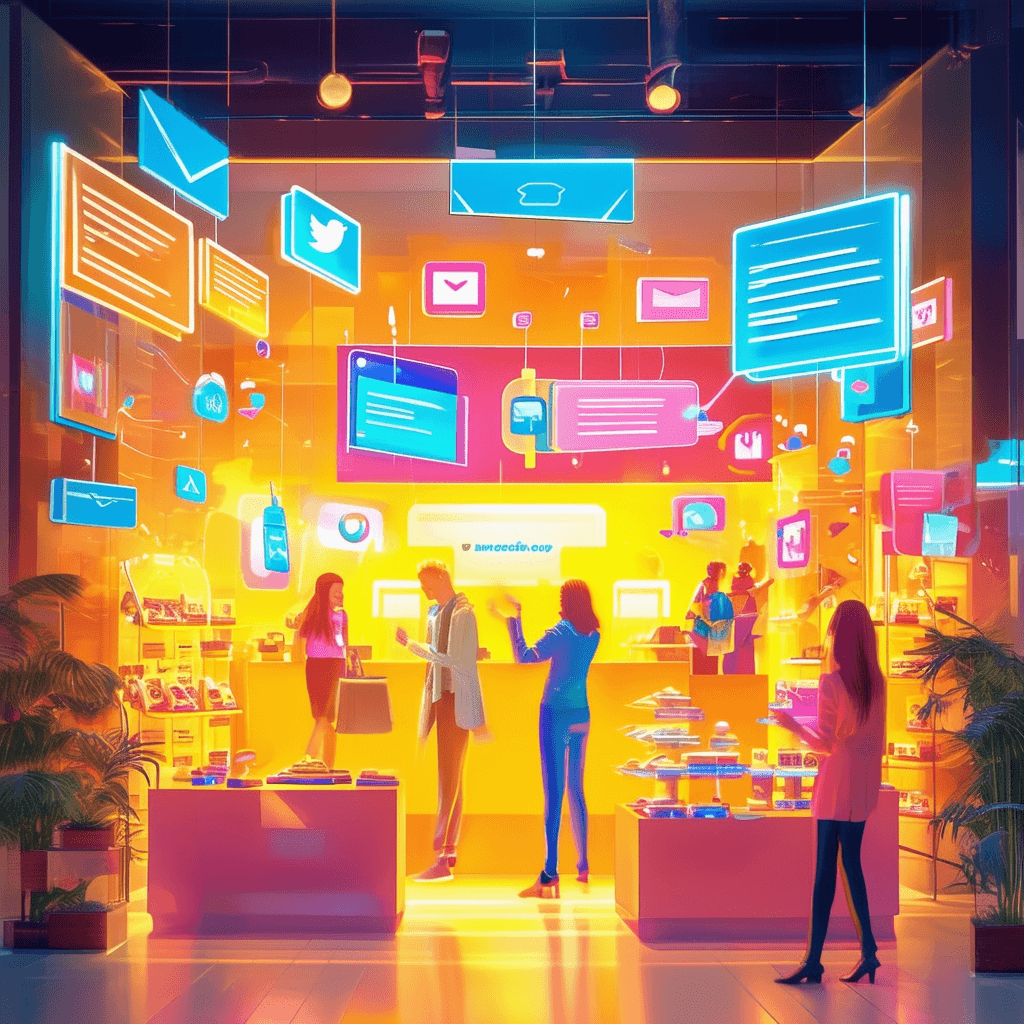
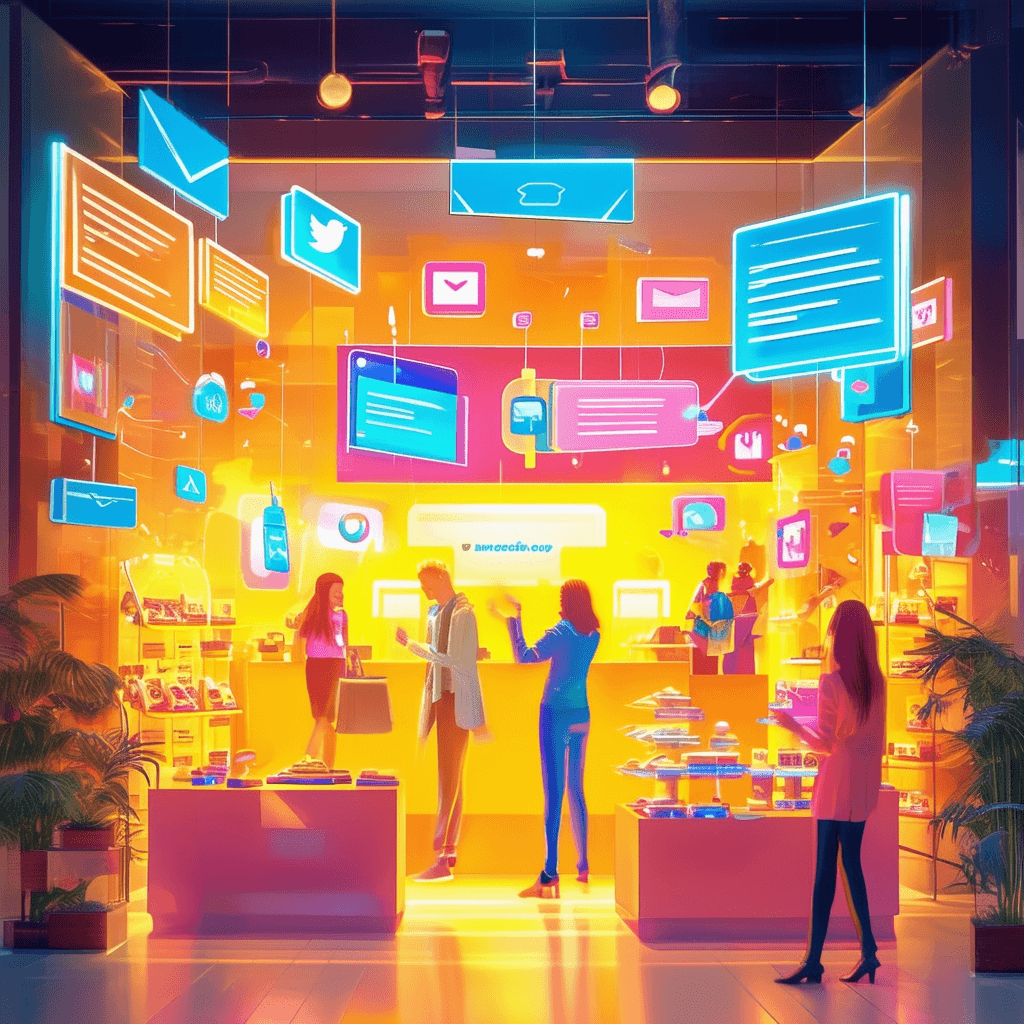
The shift toward guarding customer data will boost influencer marketing
The shift toward guarding customer data will boost influencer marketing
The shift toward guarding customer data will boost influencer marketing
The shift toward guarding customer data will boost influencer marketing
Feb 29, 2020
Feb 29, 2020
Explore
Explore
Blog
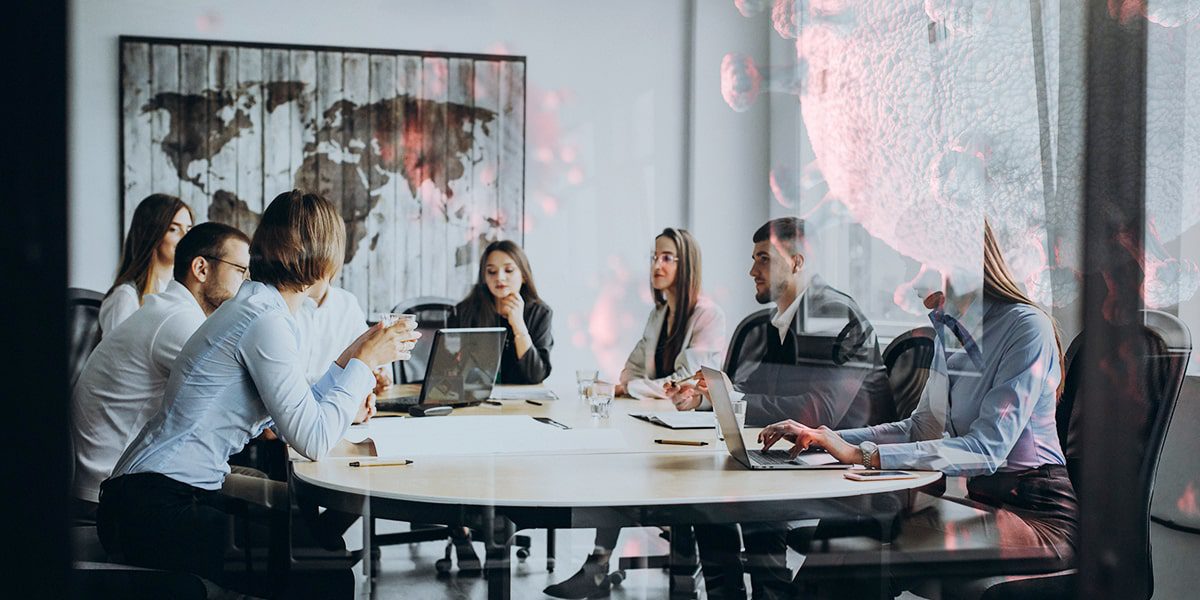
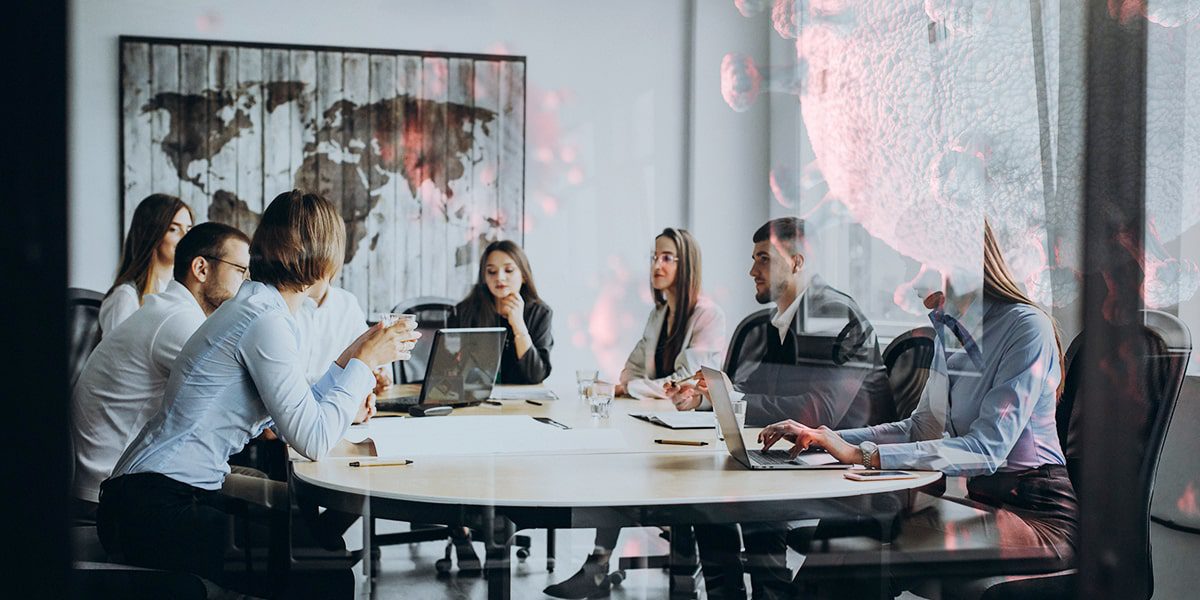
This is a Good Time to Revisit Your Value Proposition
This is a Good Time to Revisit Your Value Proposition
This is a Good Time to Revisit Your Value Proposition
This is a Good Time to Revisit Your Value Proposition
May 22, 2020
May 22, 2020
Explore
Explore
Blog
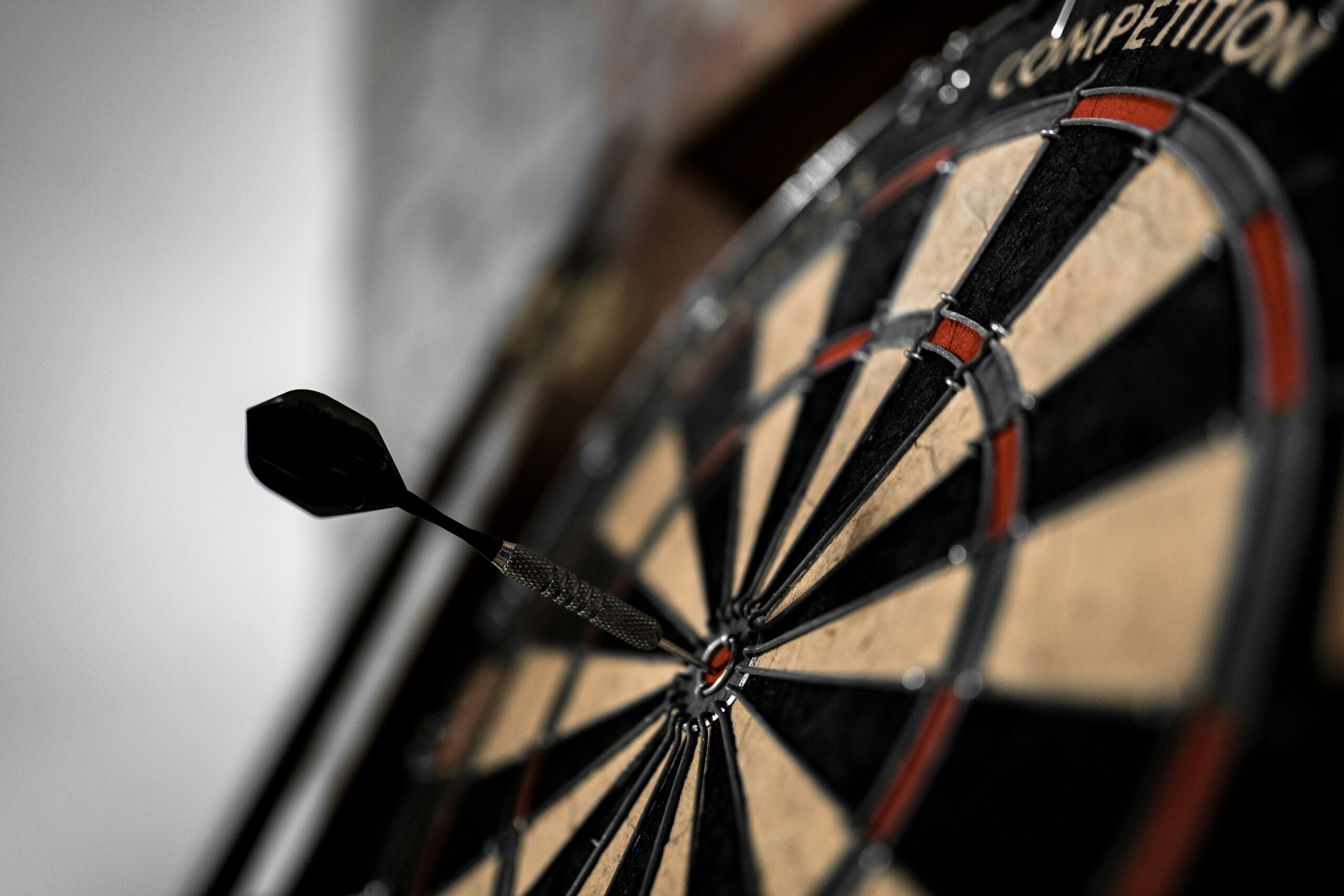
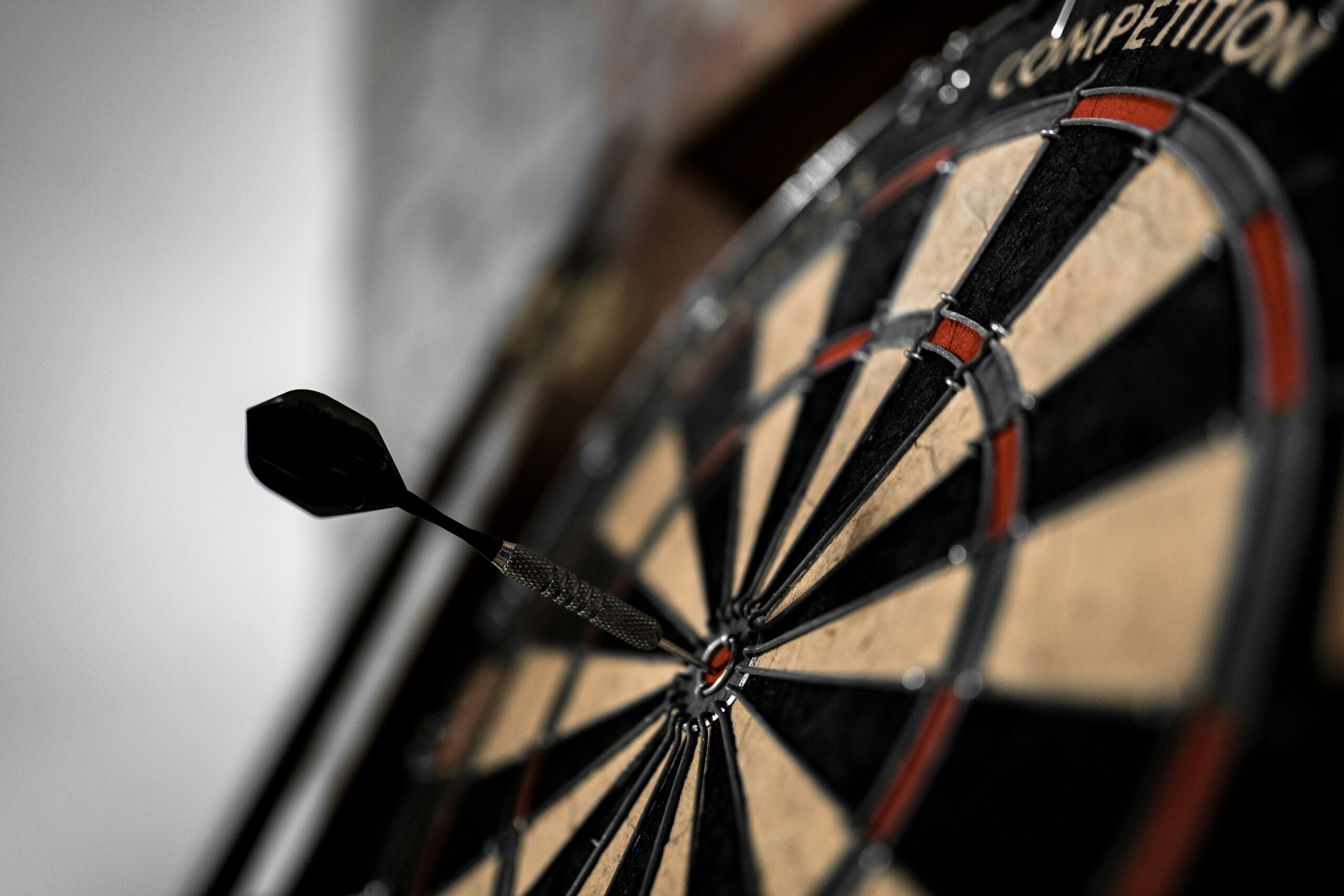
Transform Your Marketing with Personalization Based on Customer Preferences
Transform Your Marketing with Personalization Based on Customer Preferences
Transform Your Marketing with Personalization Based on Customer Preferences
Transform Your Marketing with Personalization Based on Customer Preferences
Jan 29, 2024
Jan 29, 2024
Explore
Explore
Blog
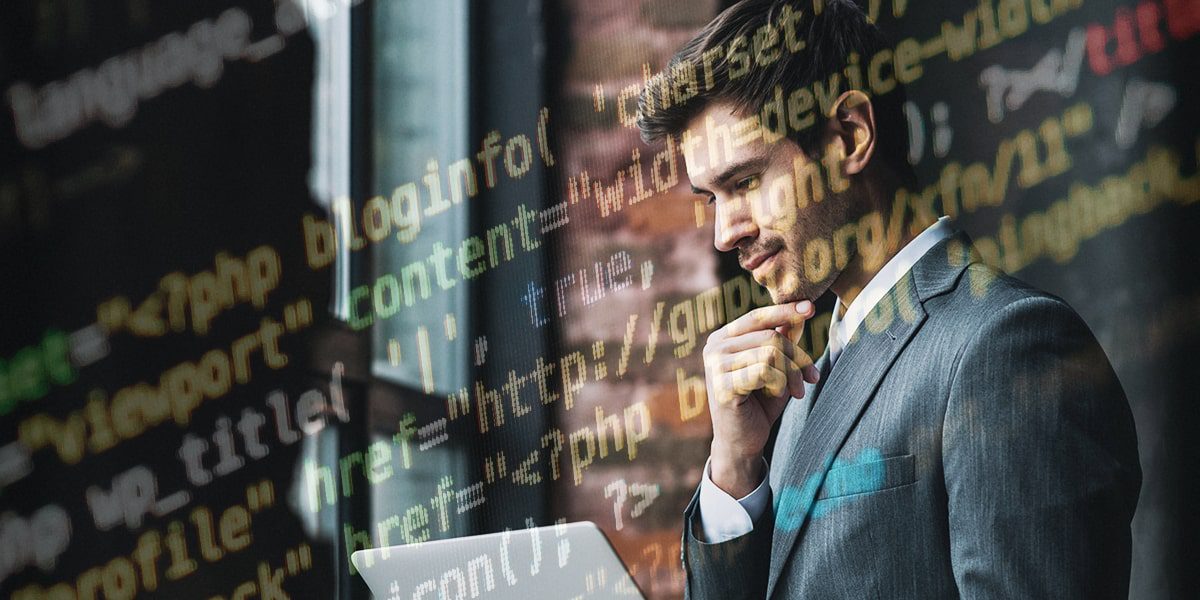
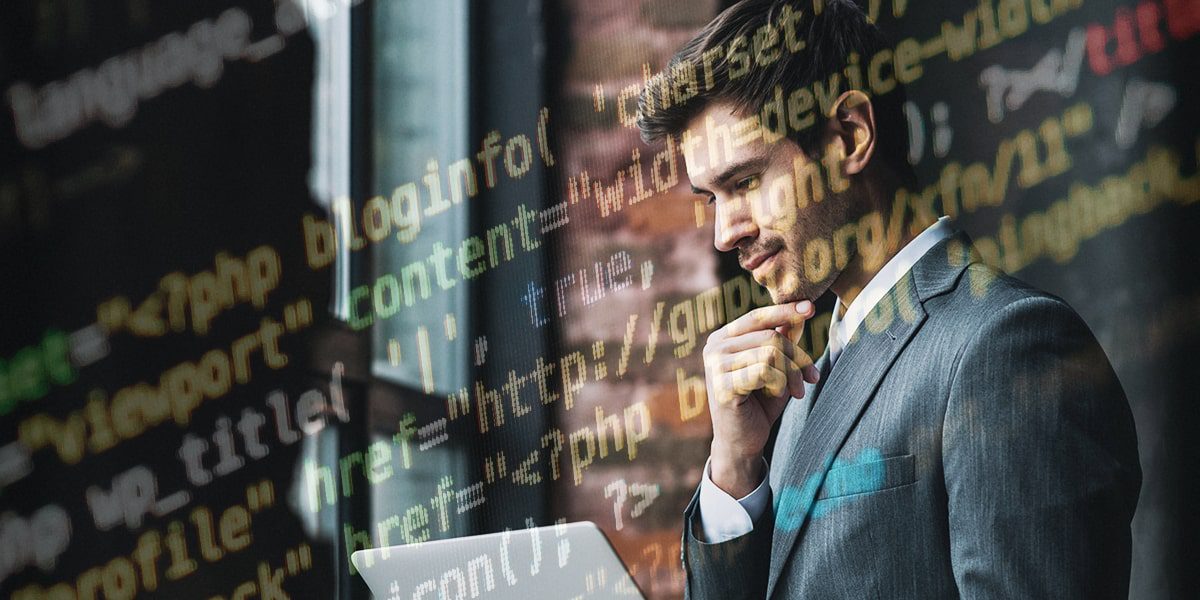
Using AI to do business mid-crisis and beyond
Using AI to do business mid-crisis and beyond
Using AI to do business mid-crisis and beyond
Using AI to do business mid-crisis and beyond
May 15, 2020
May 15, 2020
Explore
Explore
Blog
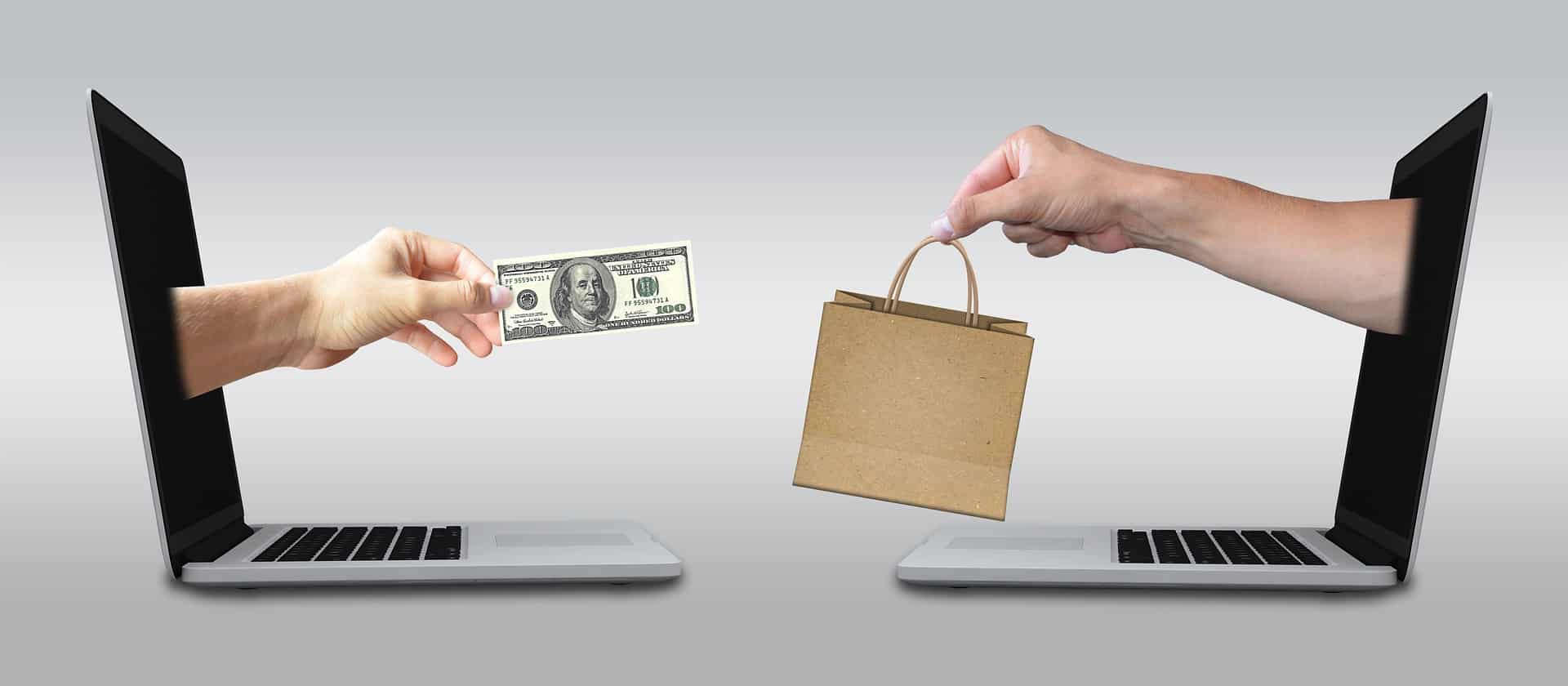
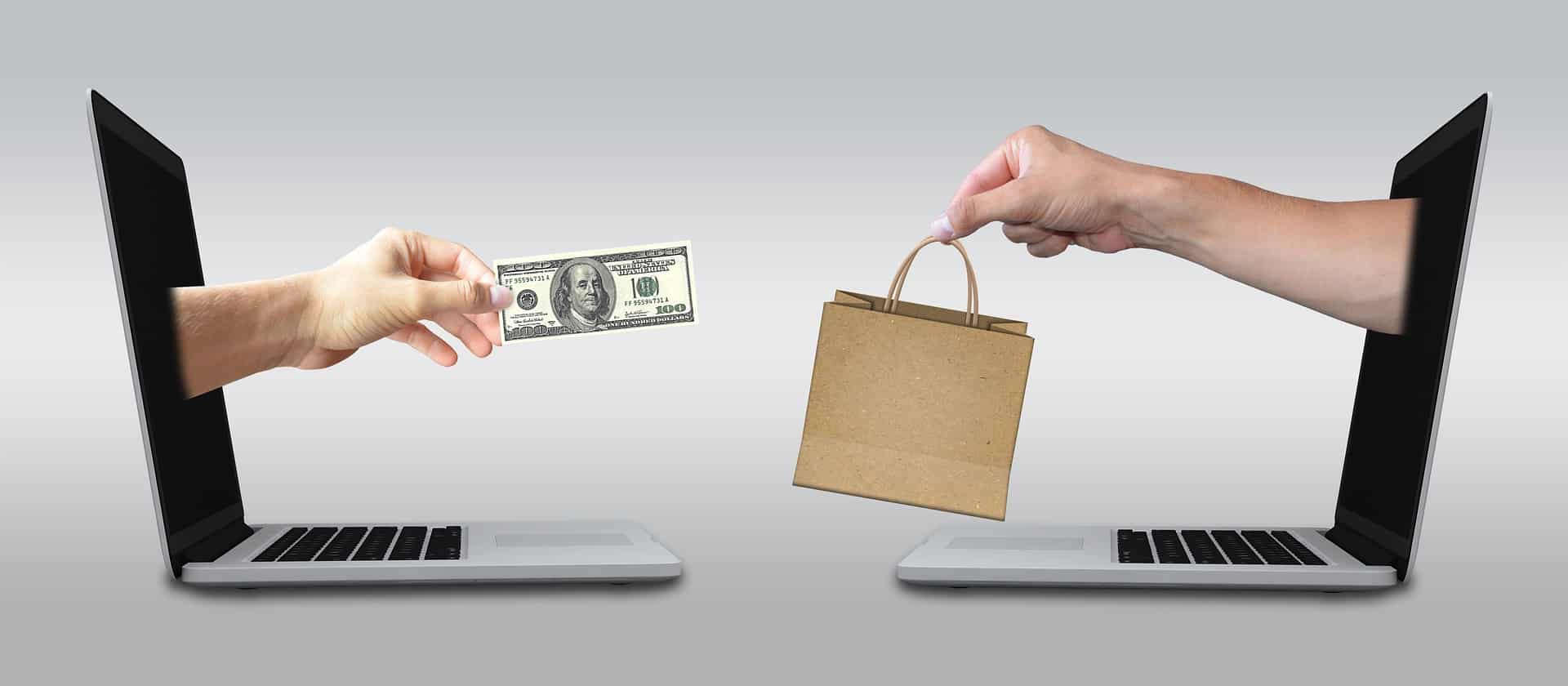
Why Behavioral Segmentation Matters More Than Demographics
Why Behavioral Segmentation Matters More Than Demographics
Why Behavioral Segmentation Matters More Than Demographics
Why Behavioral Segmentation Matters More Than Demographics
Apr 27, 2023
Apr 27, 2023
Explore
Explore
Blog
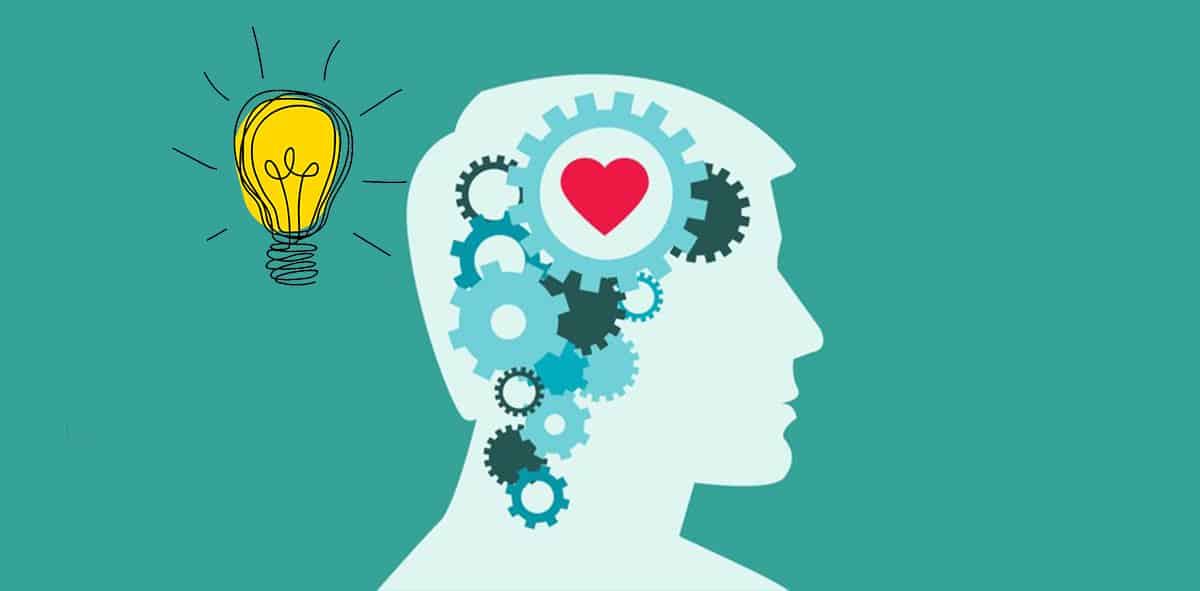
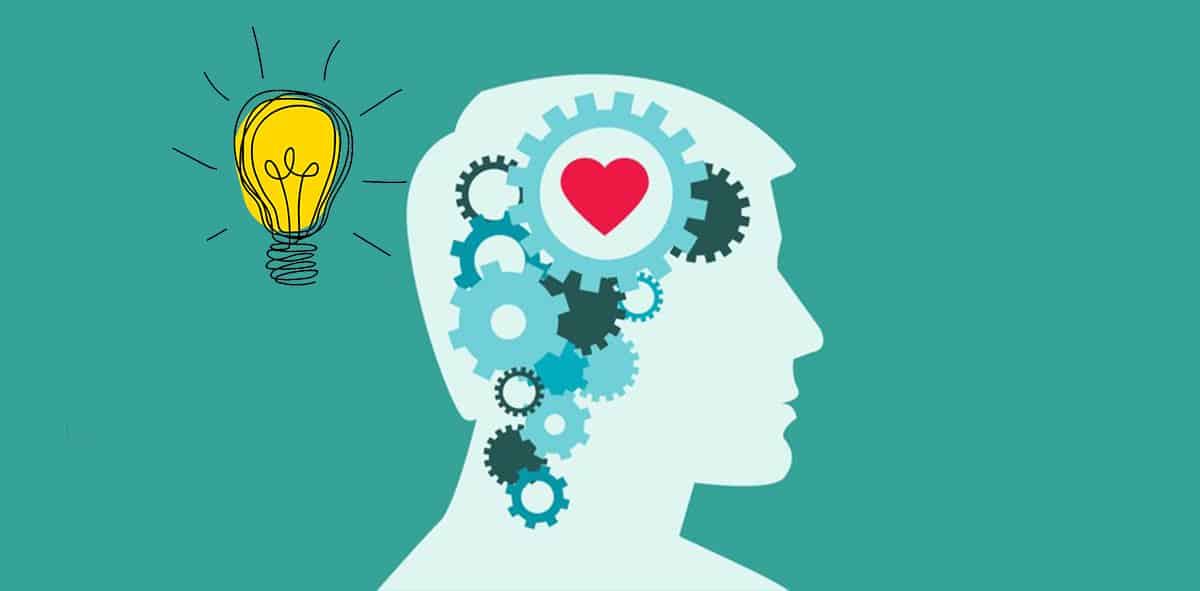
Why EQ (Emotional Intelligence) in Marketing & Sales Is More Important Than Ever
Why EQ (Emotional Intelligence) in Marketing & Sales Is More Important Than Ever
Why EQ (Emotional Intelligence) in Marketing & Sales Is More Important Than Ever
Why EQ (Emotional Intelligence) in Marketing & Sales Is More Important Than Ever
Jul 24, 2020
Jul 24, 2020
Explore
Explore
Blog
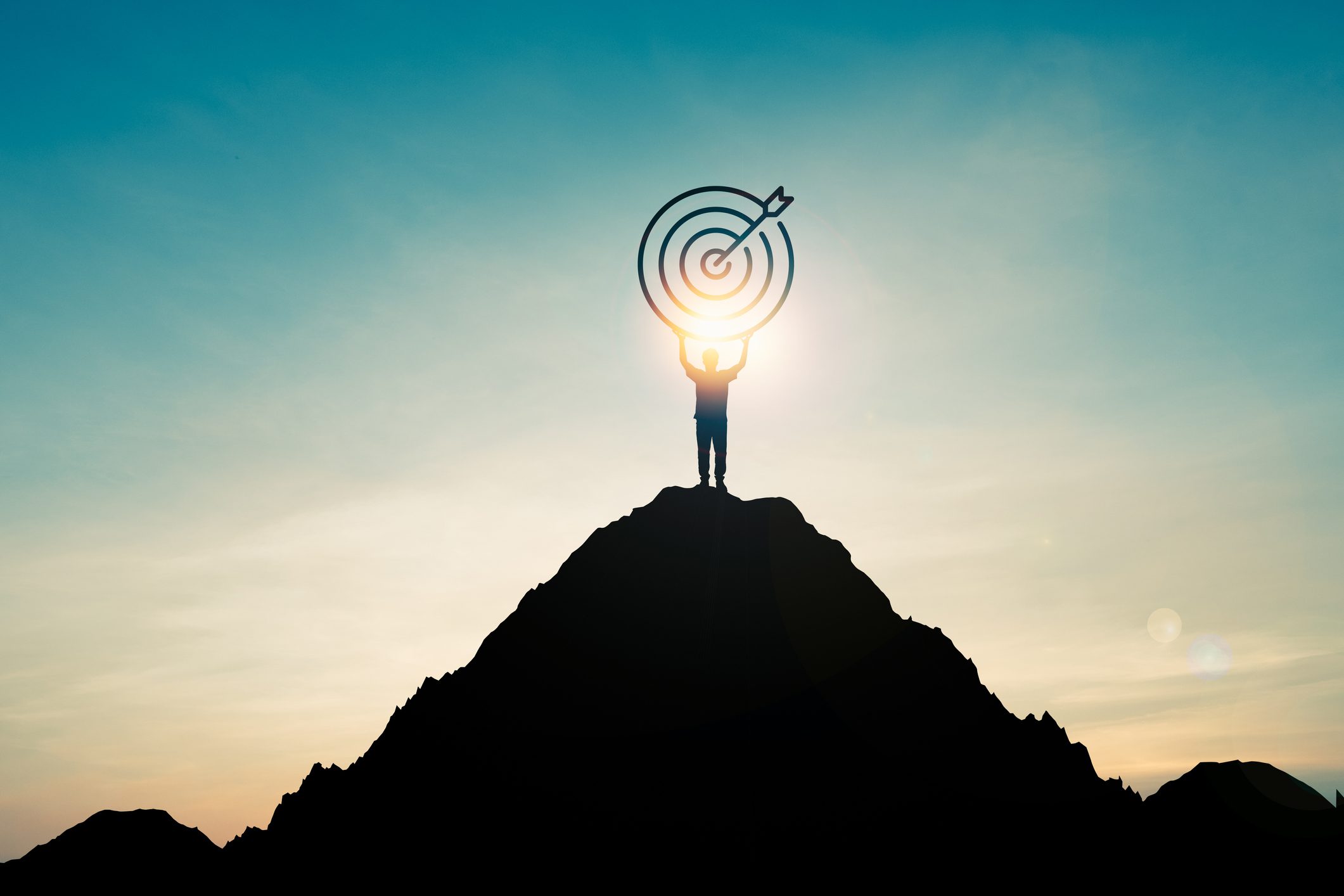
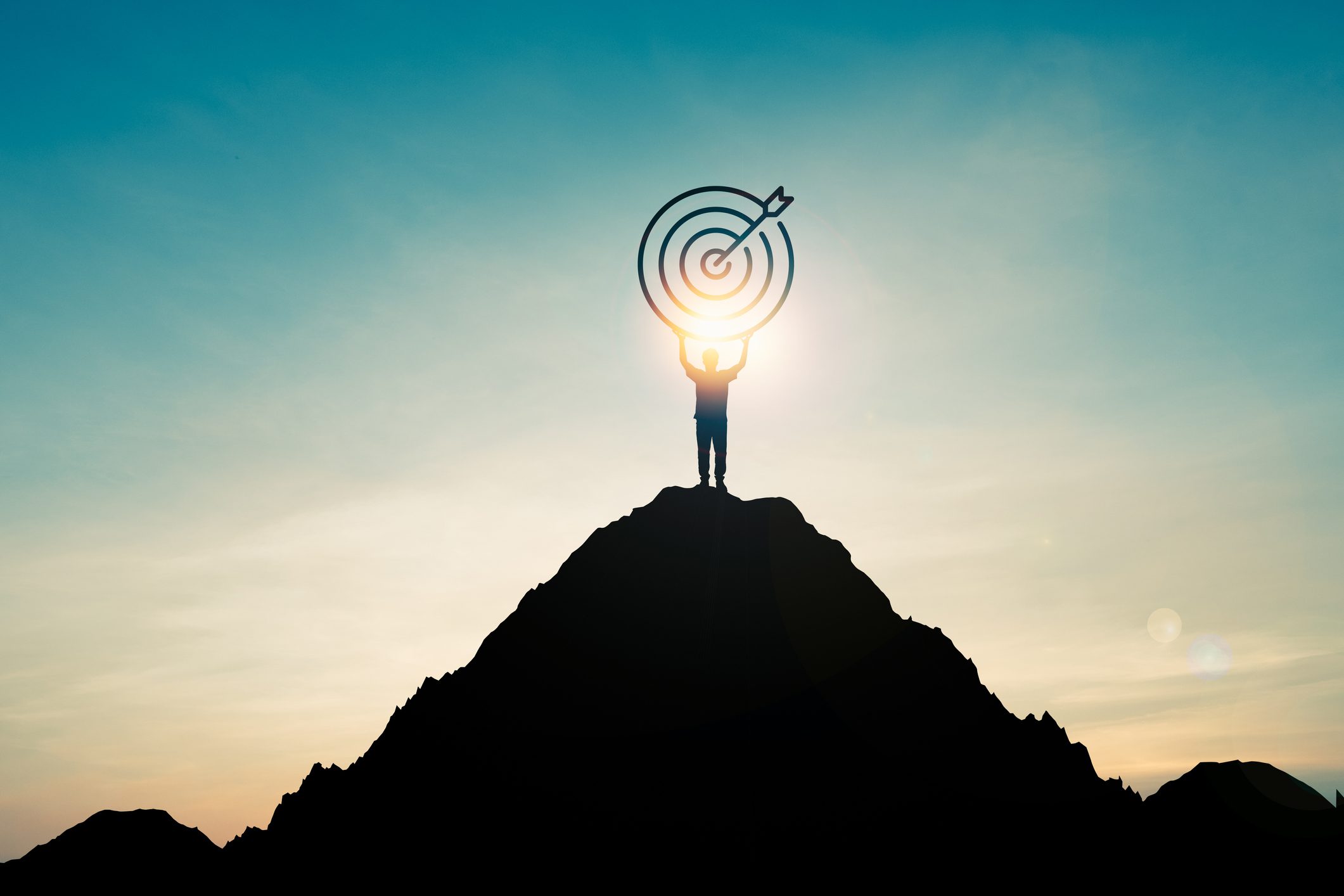
Wrench.ai & Rule27 Design Announce New AI Personalization App for Salesforce
Wrench.ai & Rule27 Design Announce New AI Personalization App for Salesforce
Wrench.ai & Rule27 Design Announce New AI Personalization App for Salesforce
Wrench.ai & Rule27 Design Announce New AI Personalization App for Salesforce
Nov 10, 2023
Nov 10, 2023
Explore
Explore
News
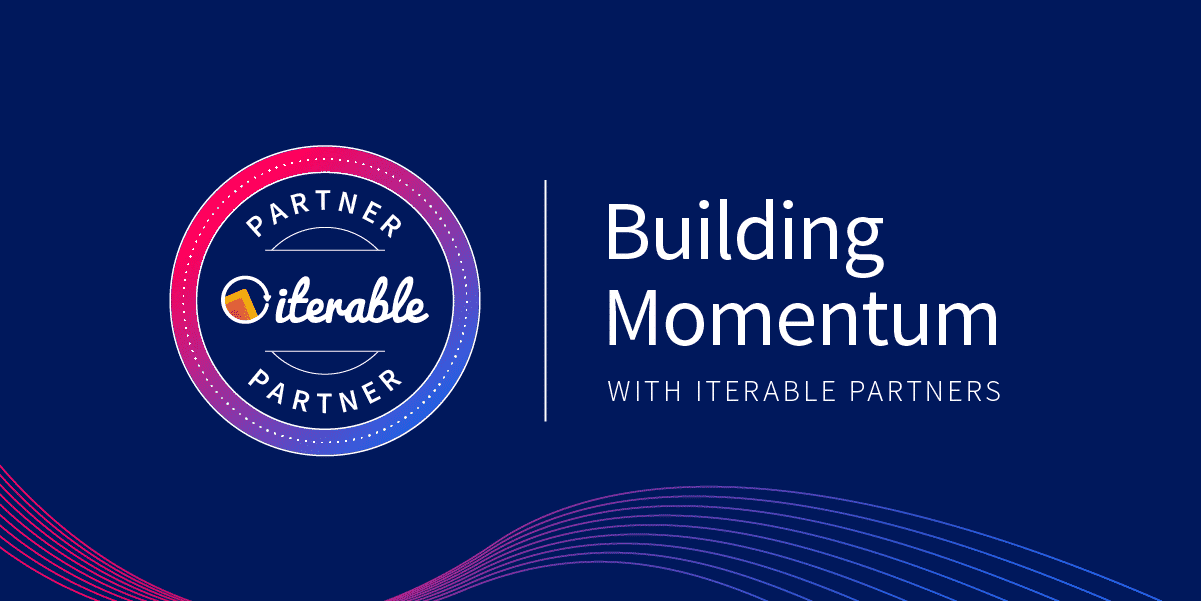
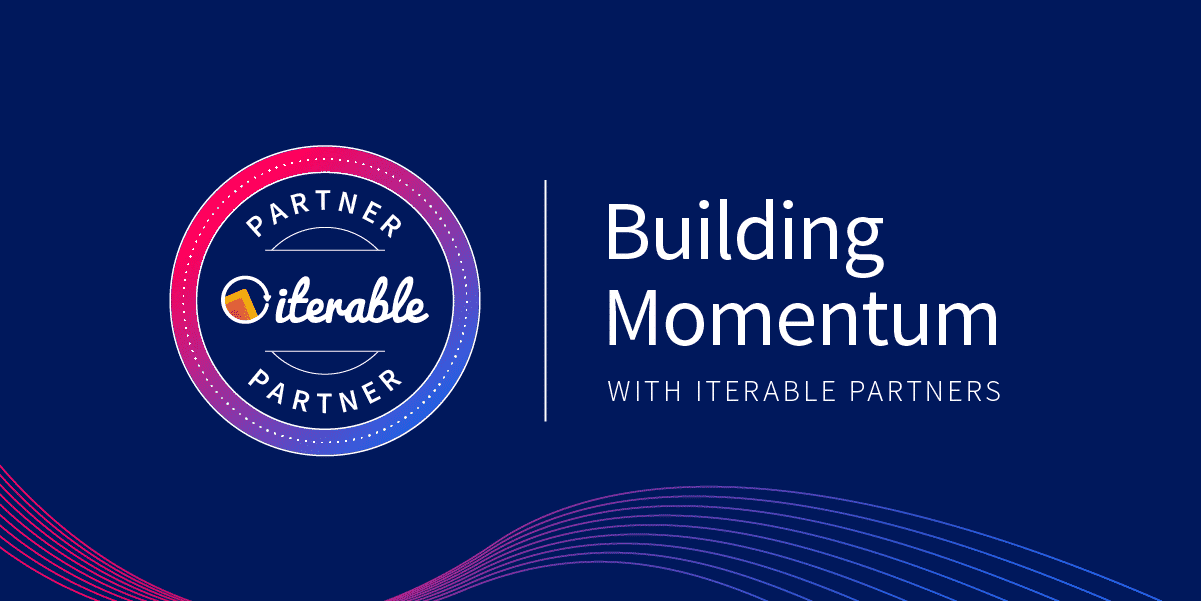
Wrench.ai and Iterable Simplify the Path to Personalization
Wrench.ai and Iterable Simplify the Path to Personalization
Wrench.ai and Iterable Simplify the Path to Personalization
Wrench.ai and Iterable Simplify the Path to Personalization
Jan 3, 2019
Jan 3, 2019
Explore
Explore
News
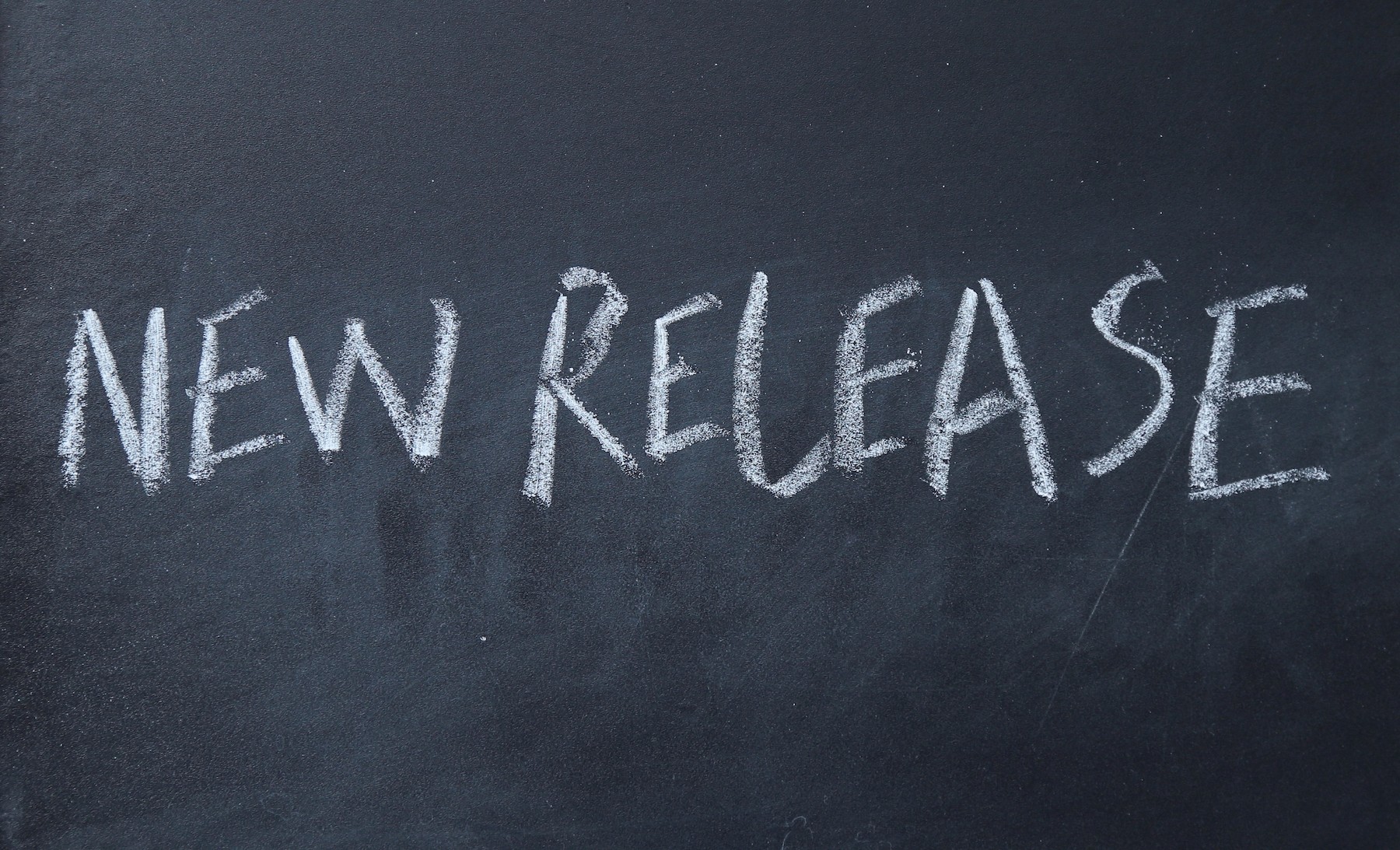
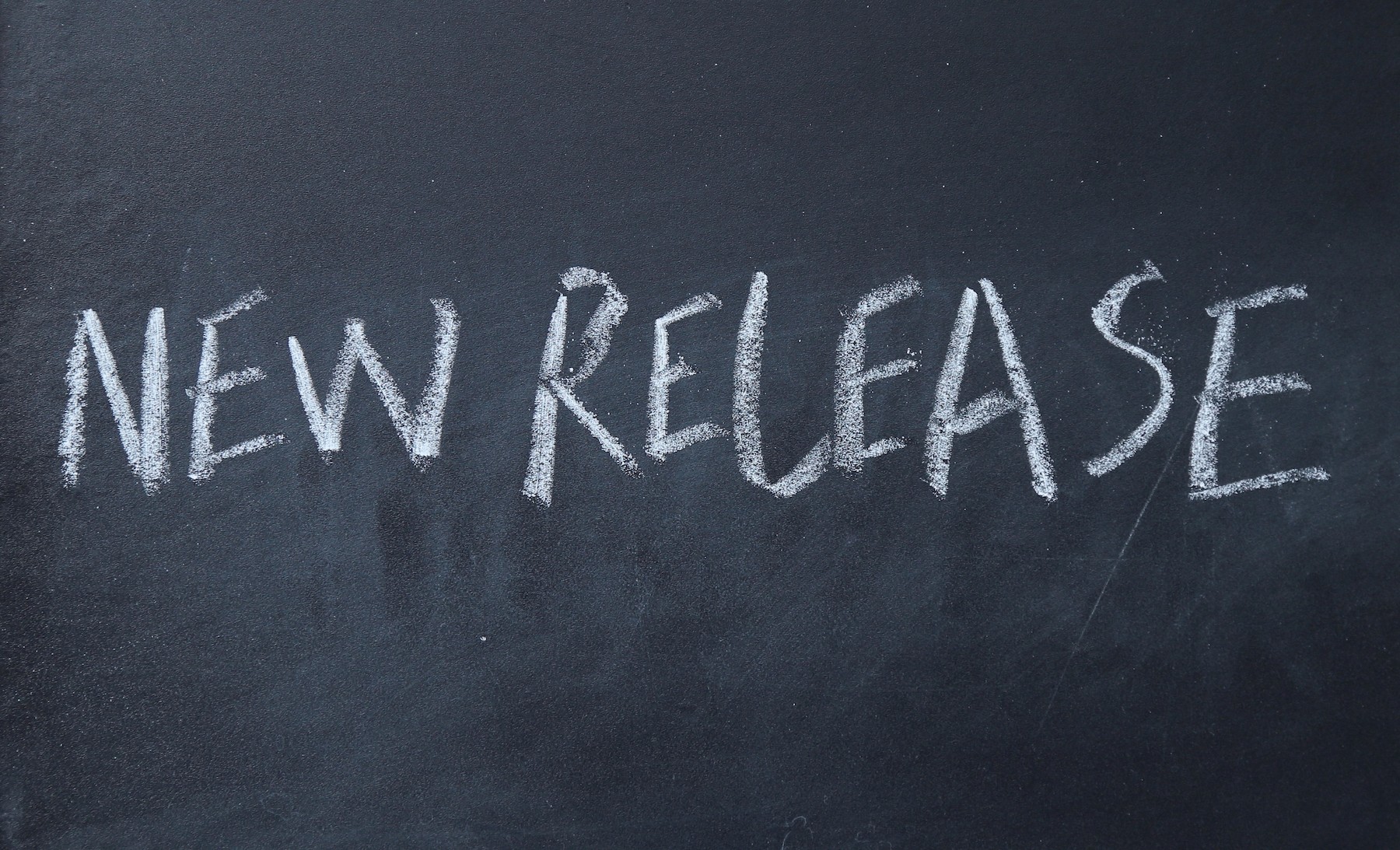
Wrench.ai Launches Creative Content AI: Content Generation for Marketing & Sales at Scale
Wrench.ai Launches Creative Content AI: Content Generation for Marketing & Sales at Scale
Wrench.ai Launches Creative Content AI: Content Generation for Marketing & Sales at Scale
Wrench.ai Launches Creative Content AI: Content Generation for Marketing & Sales at Scale
Mar 30, 2023
Mar 30, 2023
Explore
Explore
Blog


Wrench.ai Partners with Marketing Leader Refuel Agency for Revolutionary AI-Driven Campaigns
Wrench.ai Partners with Marketing Leader Refuel Agency for Revolutionary AI-Driven Campaigns
Wrench.ai Partners with Marketing Leader Refuel Agency for Revolutionary AI-Driven Campaigns
Wrench.ai Partners with Marketing Leader Refuel Agency for Revolutionary AI-Driven Campaigns
Nov 8, 2023
Nov 8, 2023
Explore
Explore
News
Contact us for consultation
Info
Quick Links
Get In Touch
Hours: Mon-Fri 9:00AM - 5:00PM
Contact us for consultation
Info
Quick Links
Get In Touch
Hours: Mon-Fri 9:00AM - 5:00PM
Contact us for consultation
Info
Quick Links
Get In Touch
Hours: Mon-Fri 9:00AM - 5:00PM
Contact us for consultation
Info
Quick Links
Get In Touch
Hours: Mon-Fri 9:00AM - 5:00PM